Robust self-supervised monocular visual odometry based on prediction-update pose estimation network.
Eng. Appl. Artif. Intell.(2022)
摘要
Visual odometry aims at estimating the camera pose from video sequence, which is an important part of visual Simultaneous Localization and Mapping (SLAM). In this paper, we propose a novel prediction-update pose estimation network, PU-PoseNet, for self-supervised monocular visual odometry. It allows the network to use the effective information of the previous frame in estimating the current pose. The long-time pose consistency constraint-based motion weighted photometric loss is designed to make the network to pay more attention to the pixels of stationary objects and enhance the time consistency of estimation results. The depth image-based occlusion detection, the depth smoothness loss and auto-Masking are used to construct the depth consistency constraint loss term to reduce the influences of interferences such as occlusion. To further improve the robustness and the accuracy of the proposed method, both the depth consistency constraint and the variational auto-encoder are used for network training. For frame missing cases, a novel frame missing training strategy is used to make our method adapt frame missing cases. Extensive experiments on the KITTI dataset have validated the effectiveness of our proposed method.
更多查看译文
关键词
Monocular visual odometry,Deep learning,Pose estimation,Depth estimation
AI 理解论文
溯源树
样例
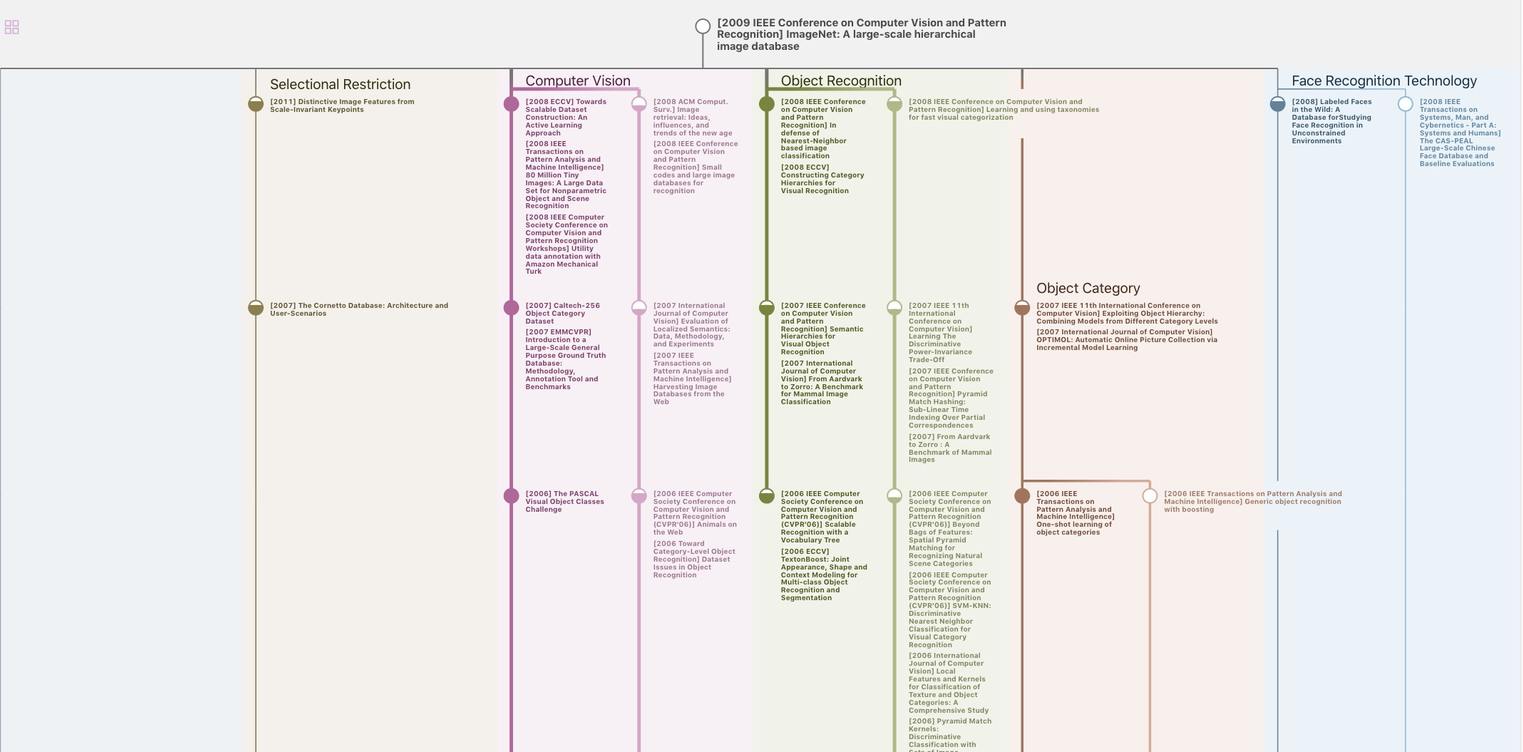
生成溯源树,研究论文发展脉络
Chat Paper
正在生成论文摘要