Estimation of shrub willow biophysical parameters across time and space from Sentinel-2 and unmanned aerial system (UAS) data
Field Crops Research(2022)
摘要
Shrub willow can be used as a perennial energy crop to produce biomass for biofuels, bioproducts, and bioenergy to help address environmental issues. Leaf chlorophyll content (LCC), leaf area index (LAI), and canopy chlorophyll content (CCC), which are closely related to vegetation health status, provide valuable information to assess willow vigor and crop management activities. We developed models for estimating LCC, LAI, and CCC from Sentinel-2 and unmanned aerial system (UAS) data using a number of vegetation indices (VIs) and applying four analytical tools: regression analysis, partial least squares regression (PLSR), random forest (RF), and neural networks (NNs). We investigated twenty VIs using regression analysis, finding the red-edge normalized difference vegetation index (NDVIre) as the optimal VI based on predictive performance and so this was included as an input variable for the PLSR, RF, and NN methods. For RF and NN models, sensitivity analysis was implemented to help tune hyperparameters and provide information to guide future studies. Results showed that the best averaged performance of Sentinel-2 and UAS data for LCC, LAI, and CCC (normalized root mean square error (NRMSE) = 9%, 12%, and 10%) of the same site in the same year was derived from models based on the NN algorithm using blue (B), green (G), red (R), red-edge (RE), and near-infrared (NIR) bands. In order to test model transferability, we collected UAS and Sentinel-2 data across different years and sites. Based on NRMSE, NN models using B, G, R, RE, and NIR bands provided the best averaged predictive performance for LCC estimation (16%) and the regression modeling using NDVIre performed best for LAI (21%) and CCC (13%) estimation for both Sentinel-2 and UAS data. Moreover, CCC estimation had smaller NRMSE values than LCC and LAI estimation for both Sentinel-2 and UAS data. Based on this study, we found that UAS data has the potential to complement Sentinel-2 data by mitigating limitations caused by temporal resolution and cloud cover. We also recommend use of the CCC parameter to evaluate shrub willow health status and perform time-series monitoring across time and space.
更多查看译文
关键词
Chlorophyll content,Leaf area index,Vegetation index,Red-edge band,Regression,Machine learning
AI 理解论文
溯源树
样例
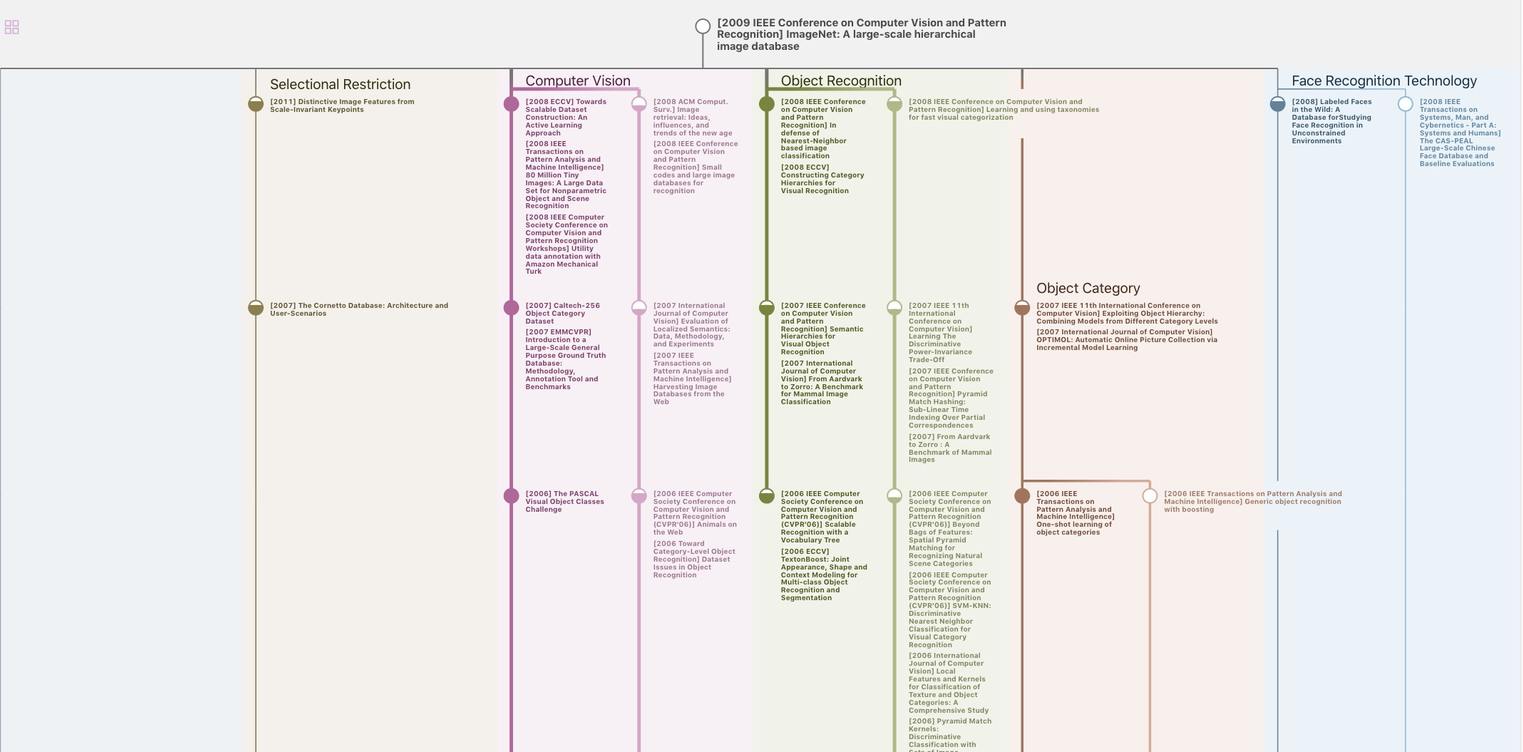
生成溯源树,研究论文发展脉络
Chat Paper
正在生成论文摘要