Virtual sensors to generate turbine runner blade strains from indirect measurements
IOP Conference Series: Earth and Environmental Science(2022)
摘要
Abstract Strain measurements on turbine blades are difficult and costly tasks. Such measurements, when carried out, generally only happen during the runner commissioning. This gives rise to two problems. The first is that some of the sensors often stop functioning properly during the measurement campaign, which leads to distorted data, and the second is that runner blade strains are not available for long-term monitoring after the measurement campaign. To alleviate the consequences of distorted or missing values, we propose the use of neural networks to automate the imputations of missing values in measurement campaign data using virtual sensors. Three types of network architecture are proposed: Long Short-Term Memory (LSTM) in different multi-stage/multi-layer configurations in Nonlinear Auto-Regressive Neural Networks with exogenous input (NARXNN), injector multi-scale attention network (Injector MA-Net), and a combined architecture using both. The performance of these architectures will be compared in four situations: the loss of strain gauge rosette branches; the loss of a complete strain gauge rosette; the loss of data on a complete blade; and the absence of strain data, which is related to the problem of identifying which sensors could be used for long-term monitoring. The performance of the proposed algorithms will be evaluated on real case scenarios from a measurement campaign during a recent unit commissioning.
更多查看译文
关键词
turbine runner blade,virtual sensors
AI 理解论文
溯源树
样例
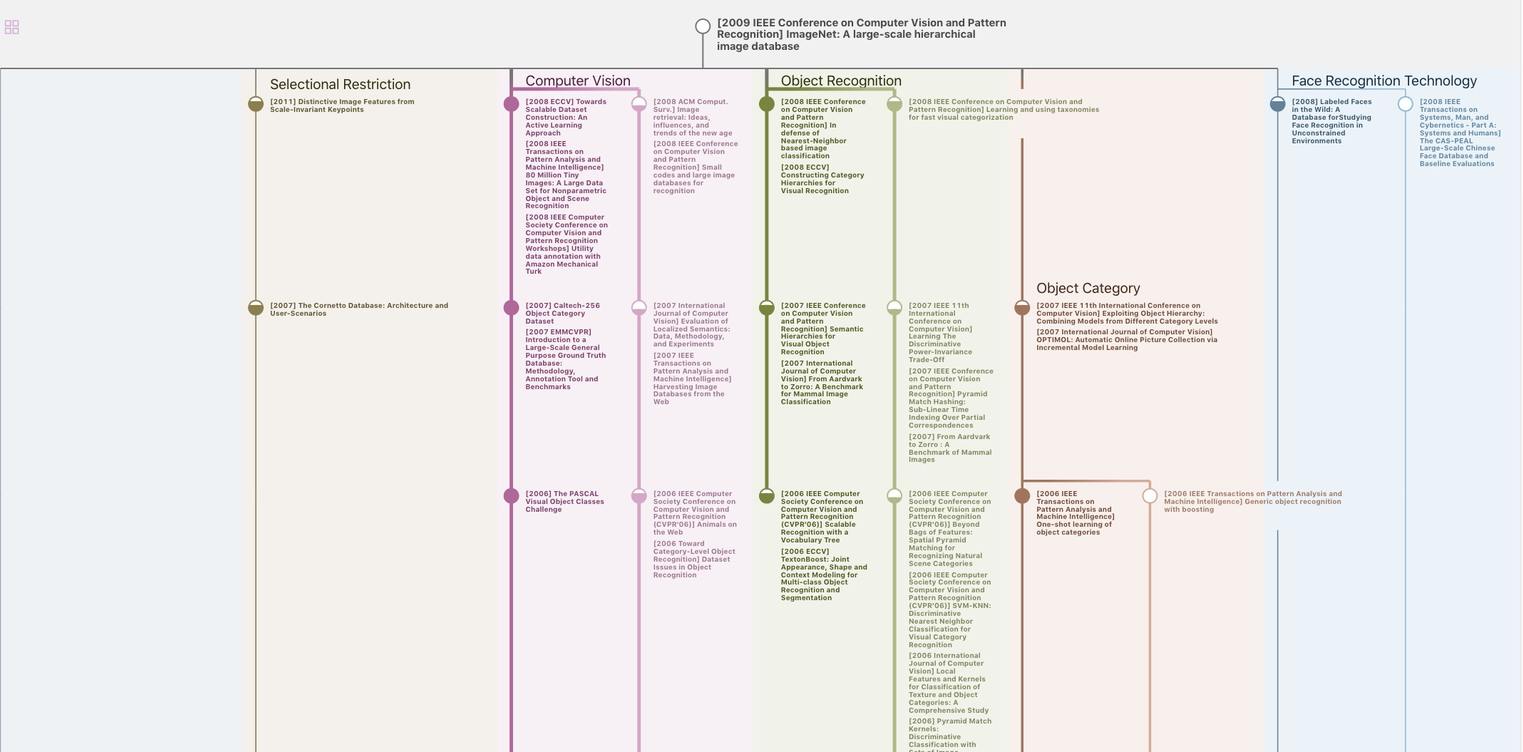
生成溯源树,研究论文发展脉络
Chat Paper
正在生成论文摘要