Machine learning model-based risk prediction of severe complications after off-pump coronary artery bypass grafting
Advances in clinical and experimental medicine : official organ Wroclaw Medical University(2023)
摘要
Background. Compared with coronary artery bypass grafting (CABG) under cardiopulmonary bypass, off-pump coronary artery bypass (OPCAB) is minimally invasive and reduces the risk of intraoperative blood transfusion and acute kidney injury. Nonetheless, OPCAB-related complications still pose a threat. Machine learning technology can analyze a large number of clinical data, establish risk prediction models and help clinicians make early and correct clinical decisions. Objectives. Risk prediction models are available for mortality and morbidity after cardiac surgery, but they are not specific to OPCAB. This study aimed to develop a predictive model of severe complications after OPCAB, based on machine learning. Materials and methods. Anesthesia records of OPCAB from the General Hospital of the Northern Theater Command (Shenyang, China) collected between January 1, 2019, and June 15, 2020, were analyzed. The endpoint of the study was the occurrence of serious complications after OPCAB (postoperative unplanned intra-aortic balloon pump, secondary surgery and death). The features entered into the models were as follows: intraoperative ventricular fibrillation, number of saphenous vein grafts, nerve block (NeB), venous oxygen saturation (SvO(2)), skin incision-bypass time, and hypertension. A total of 8 machine learning algorithms were tested: logistic regression analysis (LRA), k-nearest neighbor (KNN), naive Bayes (NB), support vector machine (SVM), random forest (RF), extreme gradient boosting (XGBoost), light gradient boosting machine (LightGBM), and categorical features gradient boosting (CatBoost). Results. Among the 506 patients found in the records, 27 met the endpoint. The highest area under the curve (AUC) value was achieved with the XGBoost model (AUC = 0.94), and the lowest with the SVM model (AUC = 0.75). The highest and lowest accuracy were observed with the XGBoost and NB models, respectively, while the highest and lowest precision were achieved using the SVM and NB models, respectively. Based on the receiver operating characteristic (ROC) curves, the XGBoost model was selected as the most useful in this study. Conclusions. This study suggests using the XGBoost model to predict the risk of complications after OPCAB.
更多查看译文
关键词
complications,machine learning,off-pump coronary artery bypass grafting,prediction model
AI 理解论文
溯源树
样例
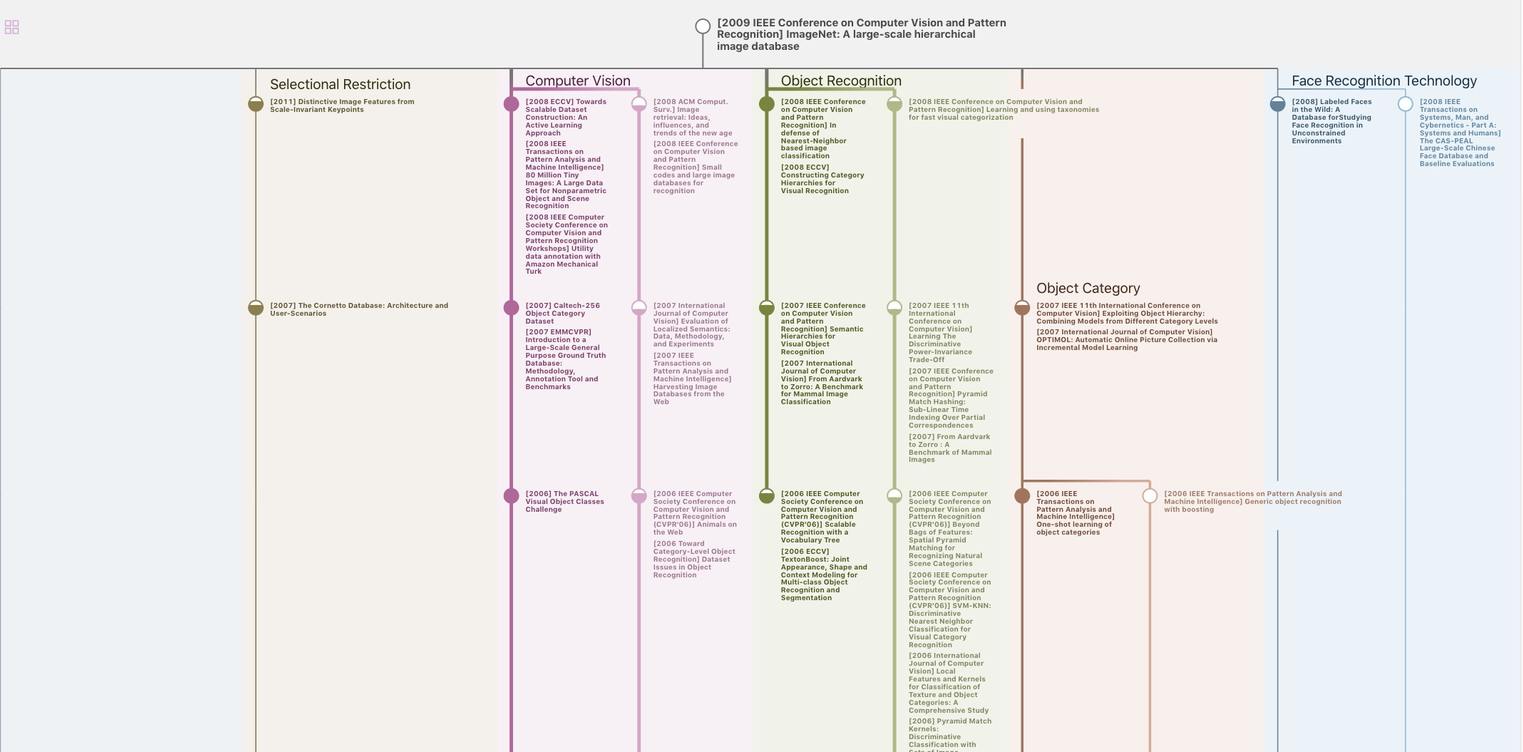
生成溯源树,研究论文发展脉络
Chat Paper
正在生成论文摘要