Correction of Susceptibility Distortion in EPI: A Semi-supervised Approach with Deep Learning.
CDMRI@MICCAI(2022)
摘要
Echo planar imaging (EPI) is the most common approach for acquiring diffusion and functional MRI data due to its high temporal resolution. However, this comes at the cost of higher sensitivity to susceptibility-induced B field inhomogeneities around air/tissue inter-faces. This leads to severe geometric distortions along the phase encoding direction (PED). To correct this distortion, the standard approach involves an analogous acquisition using an opposite PED leading to images with inverted distortions and then non-linear image registration, with a transformation model constrained along the PED, to estimate the voxelwise shift that undistorts the image pair and generates a distortion-free image. With conventional image registration approaches, this type of processing is computationally intensive. Recent advances in unsupervised deep learning-based approaches to image registration have been proposed to drastically reduce the computational cost of this task. However, they rely on maximizing an intensity-based similarity measure, known to be suboptimal surrogate measures of image alignment. To address this limitation, we propose a semi-supervised deep learning algorithm that directly leverages ground truth spatial transformations during training. Simulated and real data experiments demonstrate improvement to distortion field recovery compared to the unsupervised approach, improvement image similarity compared to supervised approach and precision similar to TOPUP but with much faster processing.
### Competing Interest Statement
The authors have declared no competing interest.
更多查看译文
关键词
susceptibility distortion,epi,deep learning,semi-supervised
AI 理解论文
溯源树
样例
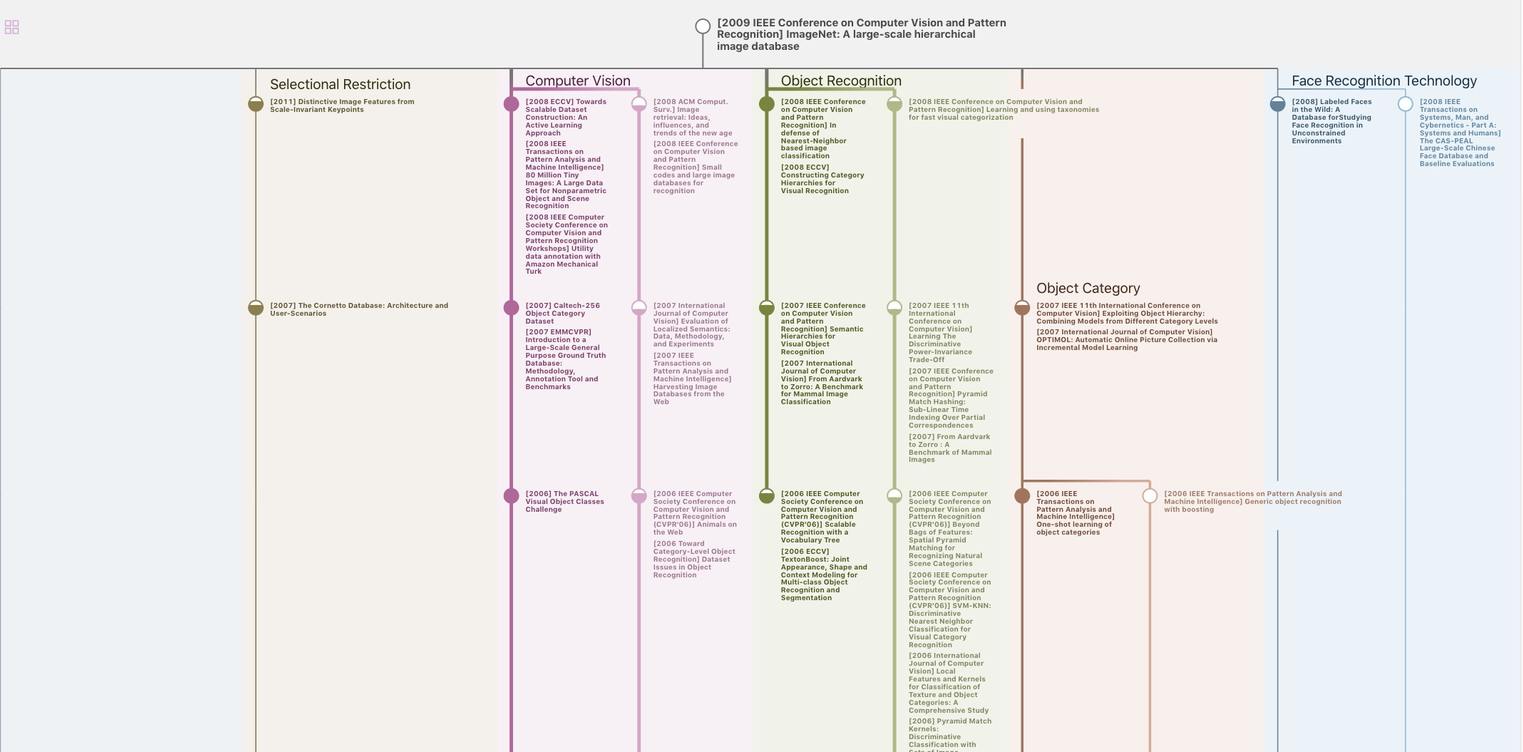
生成溯源树,研究论文发展脉络
Chat Paper
正在生成论文摘要