Deep Reinforcement Learning- based load balancing strategy for multiple controllers in SDN
e-Prime - Advances in Electrical Engineering, Electronics and Energy(2022)
摘要
In Software-Defined Network (SDN) with multiple controllers, static mapping relationship between switches and controllers may cause some controllers to be overloaded, while some controller resources are underutilized. A Deep Reinforcement Learning-based switch migration strategy (DRL-SMS) is proposed to solve the load imbalance problem in the multi-controller control plane. Based on Markov Decision Process (MDP), modeling analysis is performed for SDN to obtain system state, migration action set, and system reward. Q-values of switch migration actions are obtained by fitting approximate function using Double Deep Q-Network (DDQN), and then the DDQN is trained by using the experience replay mechanism to optimize Q-Network parameters. After training, the DRL-based strategy calculates the Q-value in the current system state and selects the migration action corresponding to the maximum Q-value to perform switch migration. Simulation experiments show that DRL-SMS can effectively balance the controller load and significantly reduce the balance time.
更多查看译文
关键词
Software-Defined Network (SDN),Controller load balancing,Deep Reinforcement Learning (DRL),Switch immigration
AI 理解论文
溯源树
样例
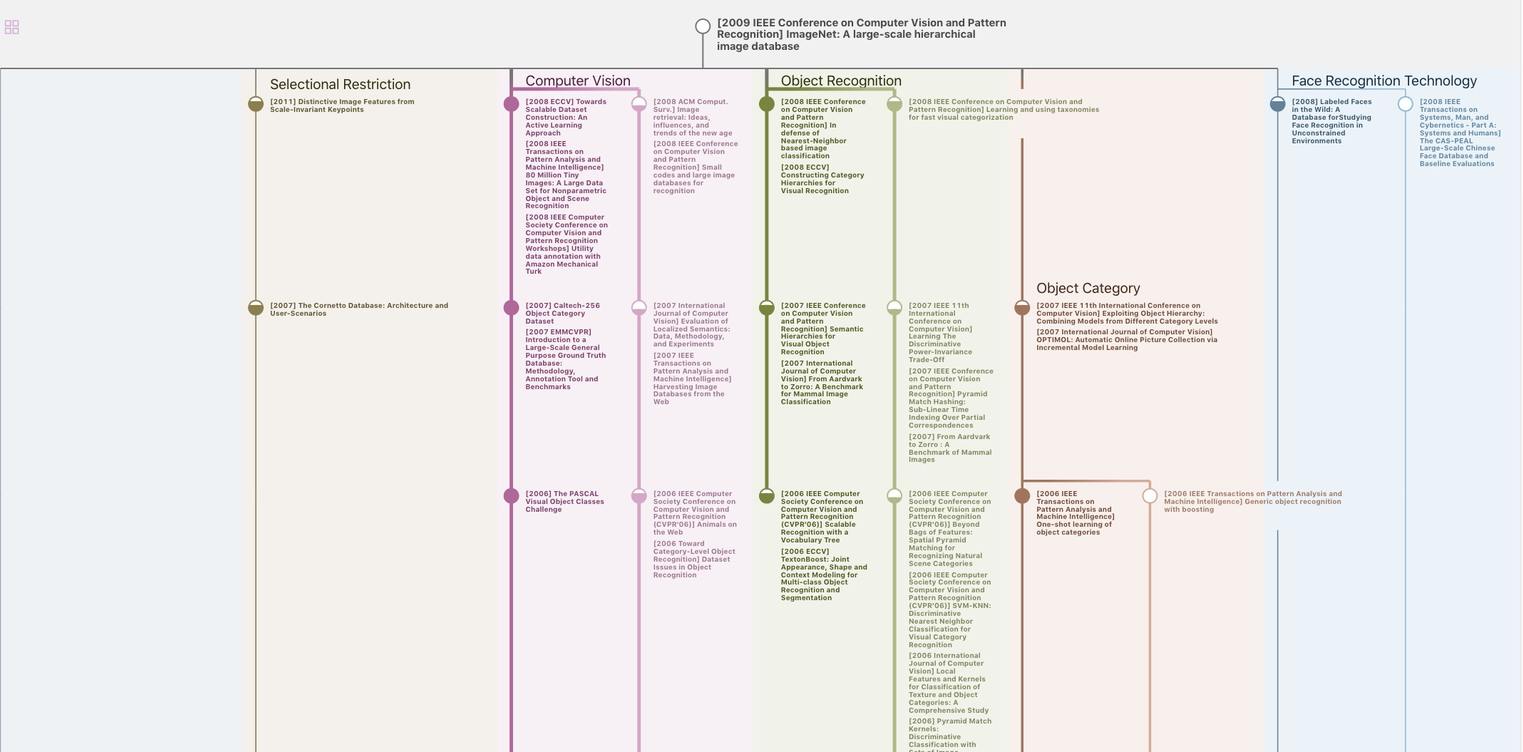
生成溯源树,研究论文发展脉络
Chat Paper
正在生成论文摘要