Approximated Gene Expression Trajectories (AGETs) for Gene Regulatory Network Inference on Cell Tracks
biorxiv(2024)
摘要
The study of pattern formation has greatly benefited from our ability to reverse-engineer gene regulatory network (GRN) structure from spatio-temporal quantitative gene expression data. Traditional approaches omit tissue morphogenesis, and focus on systems where the timescales of pattern formation and morphogenesis can be separated. In such systems, pattern forms as an emergent property of the underlying GRN and mechanistic insight can be obtained from the GRNs alone. However, this is not the case in most animal patterning systems, where patterning and morphogenesis are co-occurring and tightly linked. To address the mechanisms driving pattern formation in such systems we need to adapt our GRN inference methodologies to explicitly accommodate cell movements and tissue shape changes. In this work we present a novel framework to reverse-engineer GRNs underlying pattern formation in tissues undergoing morphogenetic changes and cell rearrangements. By integrating quantitative data from live and fixed embryos, we approximate gene expression trajectories (AGETs) in single cells and use a subset to reverse-engineer candidate GRNs using a Markov Chain Monte Carlo approach. GRN fit is assessed by simulating on cell tracks (live-modelling) and comparing the output to quantitative data-sets. This framework generates candidate GRNs that recapitulate pattern formation at the level of the tissue and the single cell. To our knowledge, this inference methodology is the first to integrate cell movements and gene expression data, making it possible to reverse-engineer GRNs patterning tissues undergoing morphogenetic changes.
### Competing Interest Statement
The authors have declared no competing interest.
更多查看译文
AI 理解论文
溯源树
样例
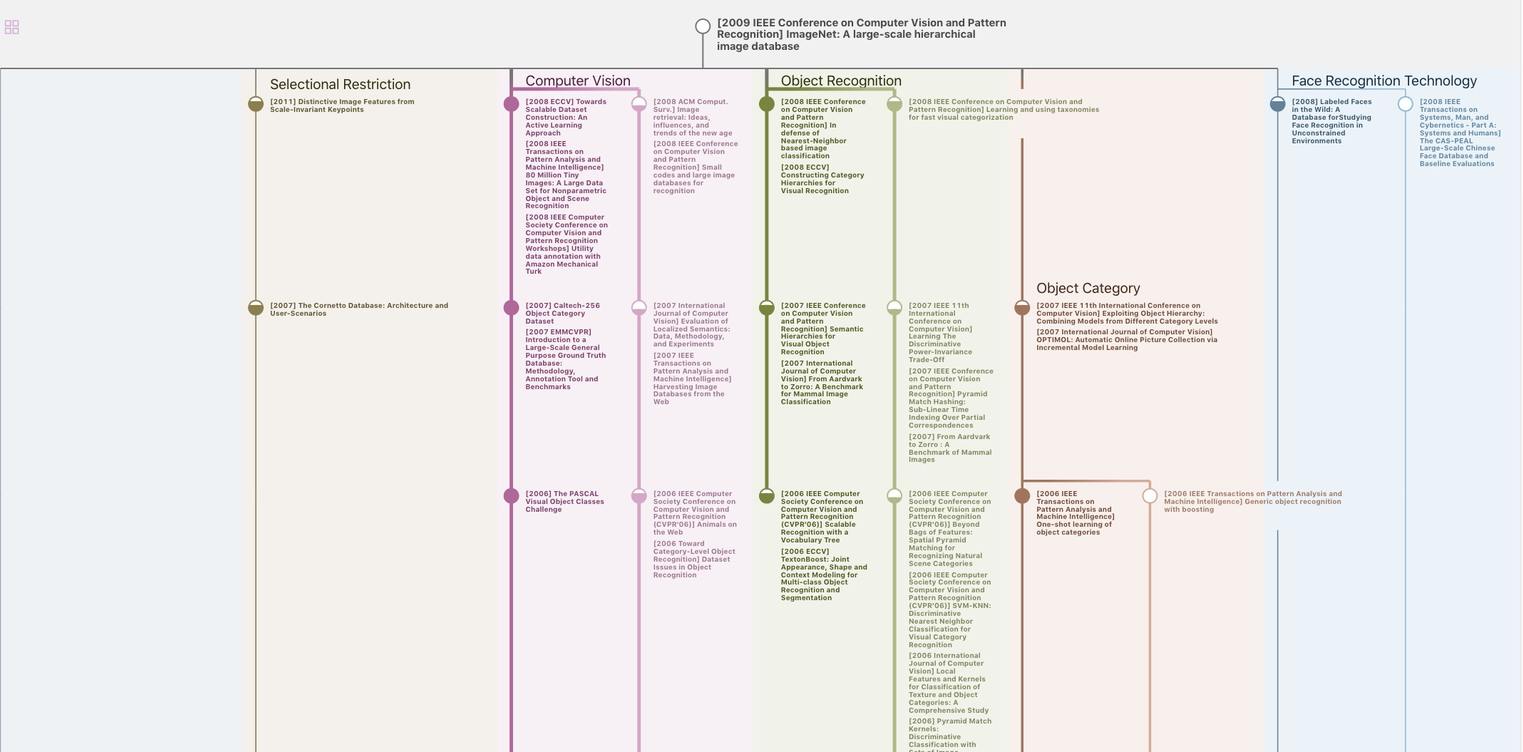
生成溯源树,研究论文发展脉络
Chat Paper
正在生成论文摘要