A Sequential Monte Carlo Approach To Anomaly Detection In Tracking Visual Events
2007 IEEE CONFERENCE ON COMPUTER VISION AND PATTERN RECOGNITION, VOLS 1-8(2007)
摘要
In this paper we propose a technique to detect anomalies in individual and interactive event sequences. We categorize anomalies into two classes: abnormal event, and abnormal context, and model them in the Sequential Monte Carlo framework which is extended by Markov Random Field for tracking interactive events. Firstly, we propose a novel pixel-wise event representation method to construct feature images, in which each blob corresponds to a visual event. Then we transform the original blob-level features into subspaces to model probabilistic appearance manifolds for each event-class. With the probability of an observation associated with each event-class (or state) derived from probabilistic manifolds, and state transitional probability, the prior and posterior state distributions can be estimated. We demonstrate in experiments that the approach can reliably detect such anomalies with low false alarm rates.
更多查看译文
关键词
markov processes,state transition,pixel,anomaly detection,sliding mode control,feature extraction,sun,monte carlo methods,sequential monte carlo,computer science,false alarm rate
AI 理解论文
溯源树
样例
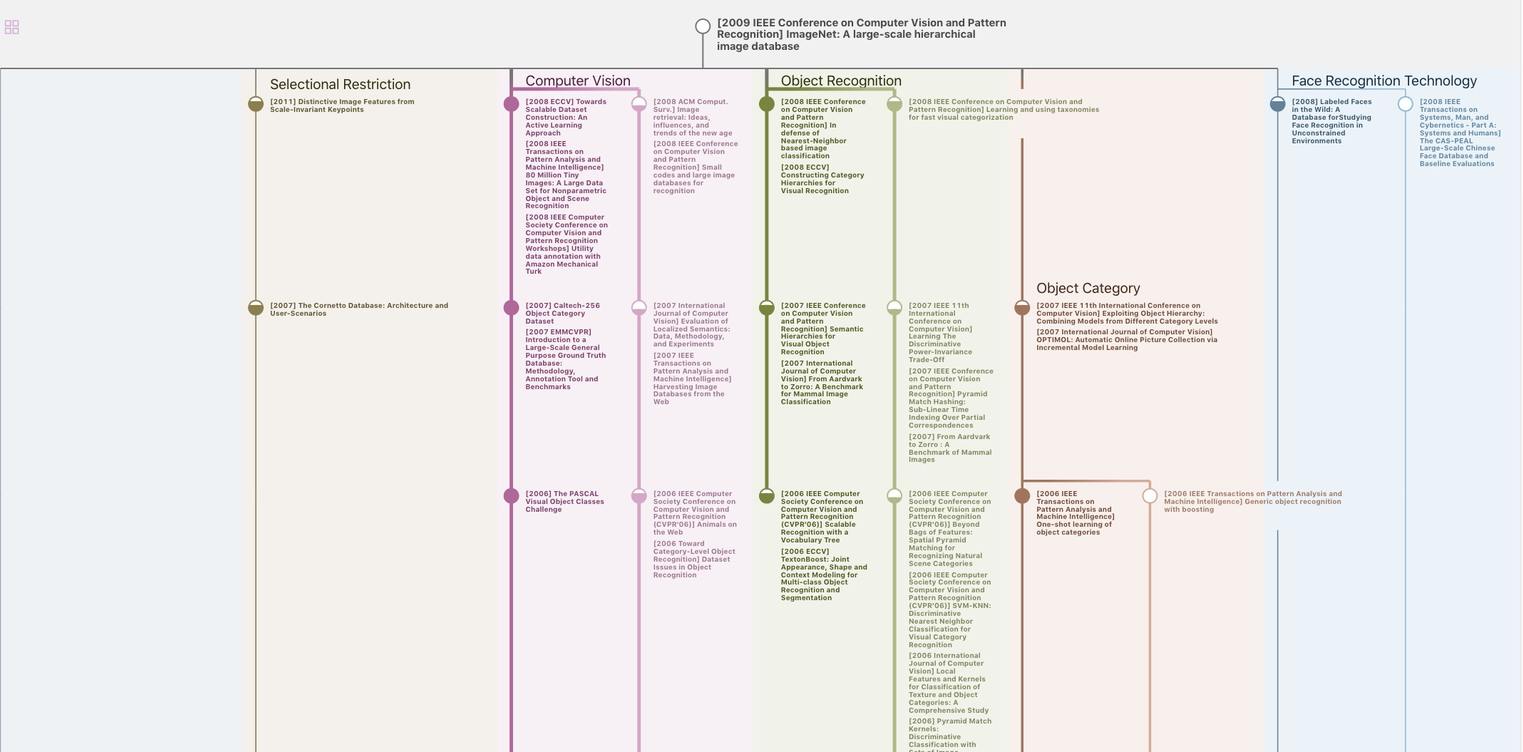
生成溯源树,研究论文发展脉络
Chat Paper
正在生成论文摘要