Enhancing deep learning based classifiers with inpainting anatomical side markers (L/R markers) for multi-center trials
Computer Methods and Programs in Biomedicine(2022)
摘要
Background and objective
The protocol for placing anatomical side markers (L/R markers) in chest radiographs varies from one hospital or department to another. However, the markers have strong signals that can be useful for deep learning-based classifier to predict diseases. We aimed to enhance the performance of a deep learning-based classifiers in multi-center datasets by inpainting the L/R markers.
Methods
The L/R marker was detected with using the EfficientDet detection network; only the detected regions were inpainted using a generative adversarial network (GAN). To analyze the effect of the inpainting in detail, deep learning-based classifiers were trained using original images, marker-inpainted images, and original images clipped using the min-max value of the marker-inpainted images. Binary classification, multi-class classification, and multi-task learning with segmentation and classification were developed and evaluated. Furthermore, the performances of the network on internal and external validation datasets were compared using DeLong's test for two correlated receiver operating characteristic (ROC) curves in binary classification and Stuart-Maxwell test for marginal homogeneity in multi-class classification and multi-task learning. In addition, the qualitative results of activation maps were evaluated using the gradient-class activation map (Grad-CAM).
Results
Marker-inpainting preprocessing improved the classification performances. In the binary classification based on the internal validation, the area under the curves (AUCs) and accuracies were 0.950 and 0.900 for the model trained on the min-max clipped images and 0.911 and 0.850 for the model trained on the original images, respectively (P-value=0.006). In the external validation, the AUCs and accuracies were 0.858 and 0.677 for the model trained using the inpainted images and 0.723 and 0.568 for the model trained using the original images (P-value<0.001), respectively. In addition, the models trained using the marker inpainted images showed the best performance in multi-class classification and multi-task learning. Furthermore, the activation maps obtained using the Grad-CAM improved with the proposed method. The 5-fold validation results also showed improvement trend according to the preprocessing strategies.
Conclusions
Inpainting an L/R marker significantly enhanced the classifier's performance and robustness, especially in internal and external studies, which could be useful in developing a more robust and accurate deep learning-based classifier for multi-center trials. The code for detection is available at: https://github.com/mi2rl/MI2RLNet. And the code for inpainting is available at: https://github.com/mi2rl/L-R-marker-inpainting.
更多查看译文
关键词
Anatomical side marker (L/R marker),Chest radiograph,Deep learning,Generative adversarial network (GAN),Gradient-class activation map (Grad-CAM),Inpainting
AI 理解论文
溯源树
样例
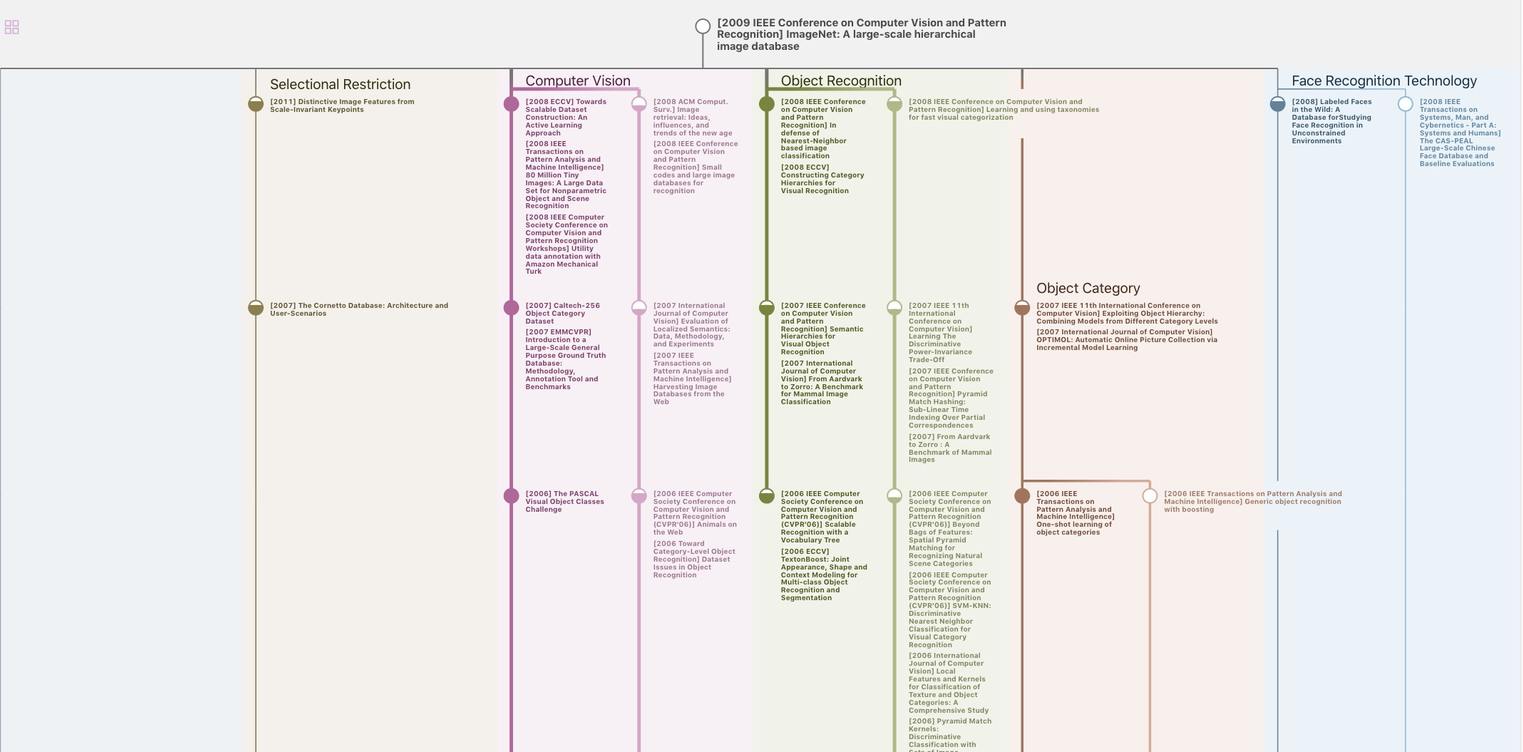
生成溯源树,研究论文发展脉络
Chat Paper
正在生成论文摘要