Constructing Natural Language Explanations via Saliency Map Verbalization
arxiv(2022)
摘要
Saliency maps can explain a neural model's prediction by identifying important input features. While they excel in being faithful to the explained model, saliency maps in their entirety are difficult to interpret for humans, especially for instances with many input features. In contrast, natural language explanations (NLEs) are flexible and can be tuned to a recipient's expectations, but are costly to generate: Rationalization models are usually trained on specific tasks and require high-quality and diverse datasets of human annotations. We combine the advantages from both explainability methods by verbalizing saliency maps. We formalize this underexplored task and propose a novel methodology that addresses two key challenges of this approach -- what and how to verbalize. Our approach utilizes efficient search methods that are task- and model-agnostic and do not require another black-box model, and hand-crafted templates to preserve faithfulness. We conduct a human evaluation of explanation representations across two natural language processing (NLP) tasks: news topic classification and sentiment analysis. Our results suggest that saliency map verbalization makes explanations more understandable and less cognitively challenging to humans than conventional heatmap visualization.
更多查看译文
AI 理解论文
溯源树
样例
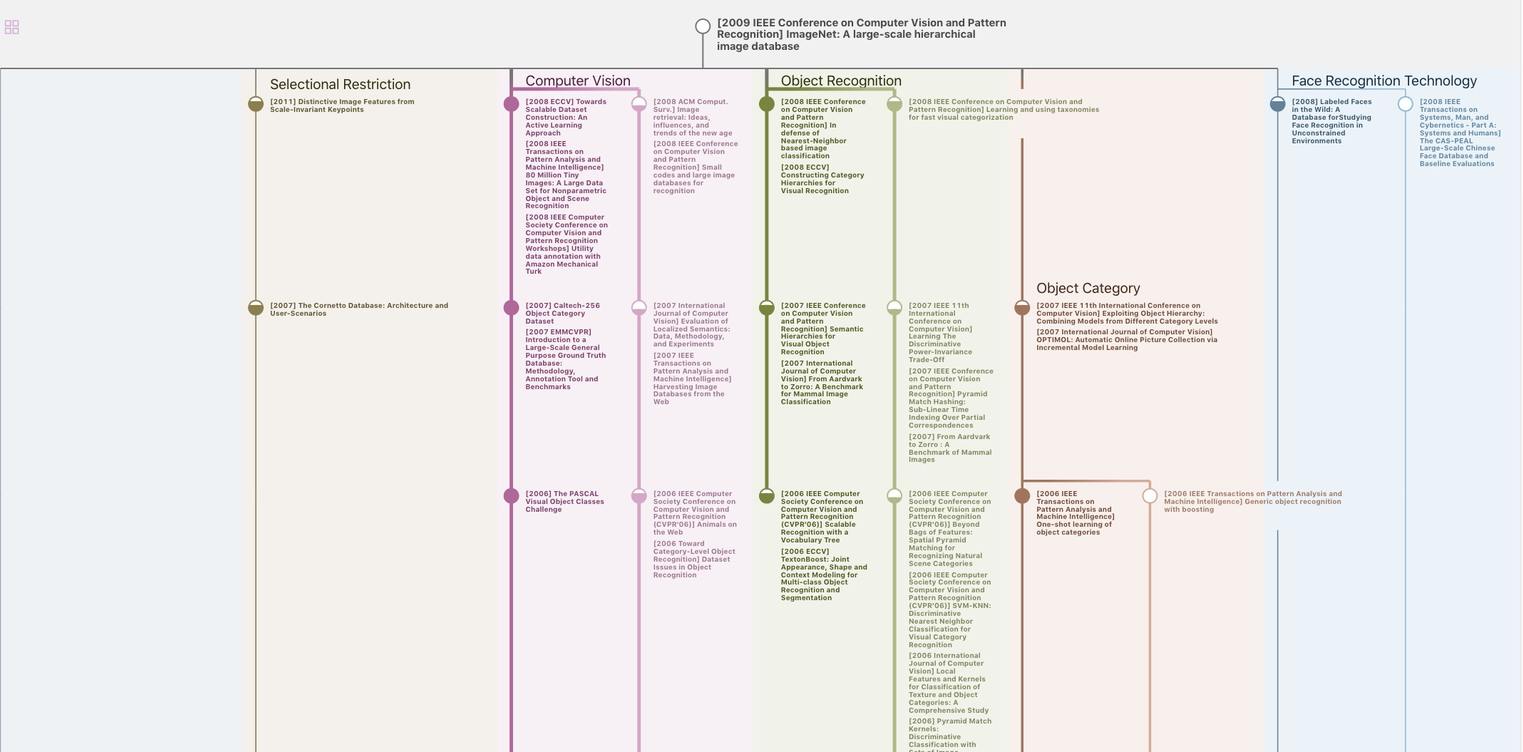
生成溯源树,研究论文发展脉络
Chat Paper
正在生成论文摘要