Pilot-wave dynamics: Using dynamic mode decomposition to characterize bifurcations, routes to chaos, and emergent statistics
arXiv (Cornell University)(2023)
摘要
We develop a data-driven characterization of the pilot-wave hydrodynamic system in which a bouncing droplet self-propels along the surface of a vibrating bath. We consider drop motion in a confined one-dimensional geometry and apply the dynamic mode decomposition (DMD) in order to characterize the evolution of the wave field as the bath's vibrational acceleration is increased progressively. Dynamic mode decomposition provides a regression framework for adaptively learning a best-fit linear dynamics model over snapshots of spatiotemporal data. Thus, DMD reduces the complex nonlinear interactions between pilot waves and droplet to a low-dimensional linear superposition of DMD modes characterizing the wave field. In particular, it provides a low-dimensional characterization of the bifurcation structure of the pilot-wave physics, wherein the excitation and recruitment of additional modes in the linear superposition models the bifurcation sequence. This DMD characterization yields a fresh perspective on the bouncing-droplet problem that forges valuable new links with the mathematical machinery of quantum mechanics. Specifically, the analysis shows that as the vibrational acceleration is increased, the pilot-wave field undergoes a series of Hopf bifurcations that ultimately lead to a chaotic wave field. The established relation between the mean pilot-wave field and the droplet statistics allows us to characterize the evolution of the emergent statistics with increased vibrational forcing from the evolution of the pilot-wave field. We thus develop a numerical framework with the same basic structure as quantum mechanics, specifically a wave theory that predicts particle statistics.
更多查看译文
关键词
dynamics mode decomposition,bifurcations,chaos,pilot-wave
AI 理解论文
溯源树
样例
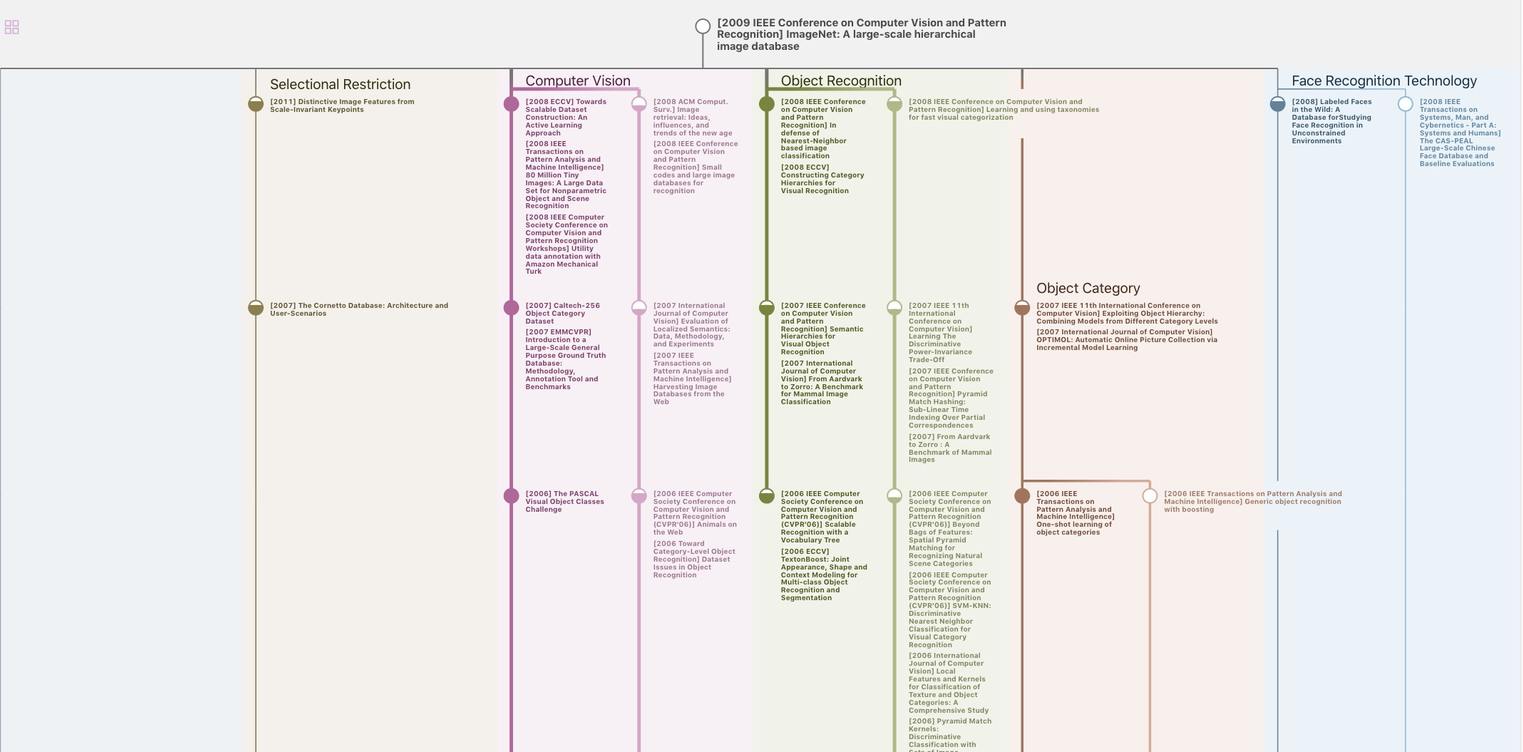
生成溯源树,研究论文发展脉络
Chat Paper
正在生成论文摘要