Variance-Aware Estimation of Kernel Mean Embedding
arxiv(2022)
摘要
An important feature of kernel mean embeddings (KME) is that the rate of convergence of the empirical KME to the true distribution KME can be bounded independently of the dimension of the space, properties of the distribution and smoothness features of the kernel. We show how to speed-up convergence by leveraging variance information in the RKHS. Furthermore, we show that even when such information is a priori unknown, we can efficiently estimate it from the data, recovering the desiderata of a distribution agnostic bound that enjoys acceleration in fortuitous settings. We illustrate our methods in the context of hypothesis testing and robust parametric estimation.
更多查看译文
关键词
kernel mean,estimation,variance-aware
AI 理解论文
溯源树
样例
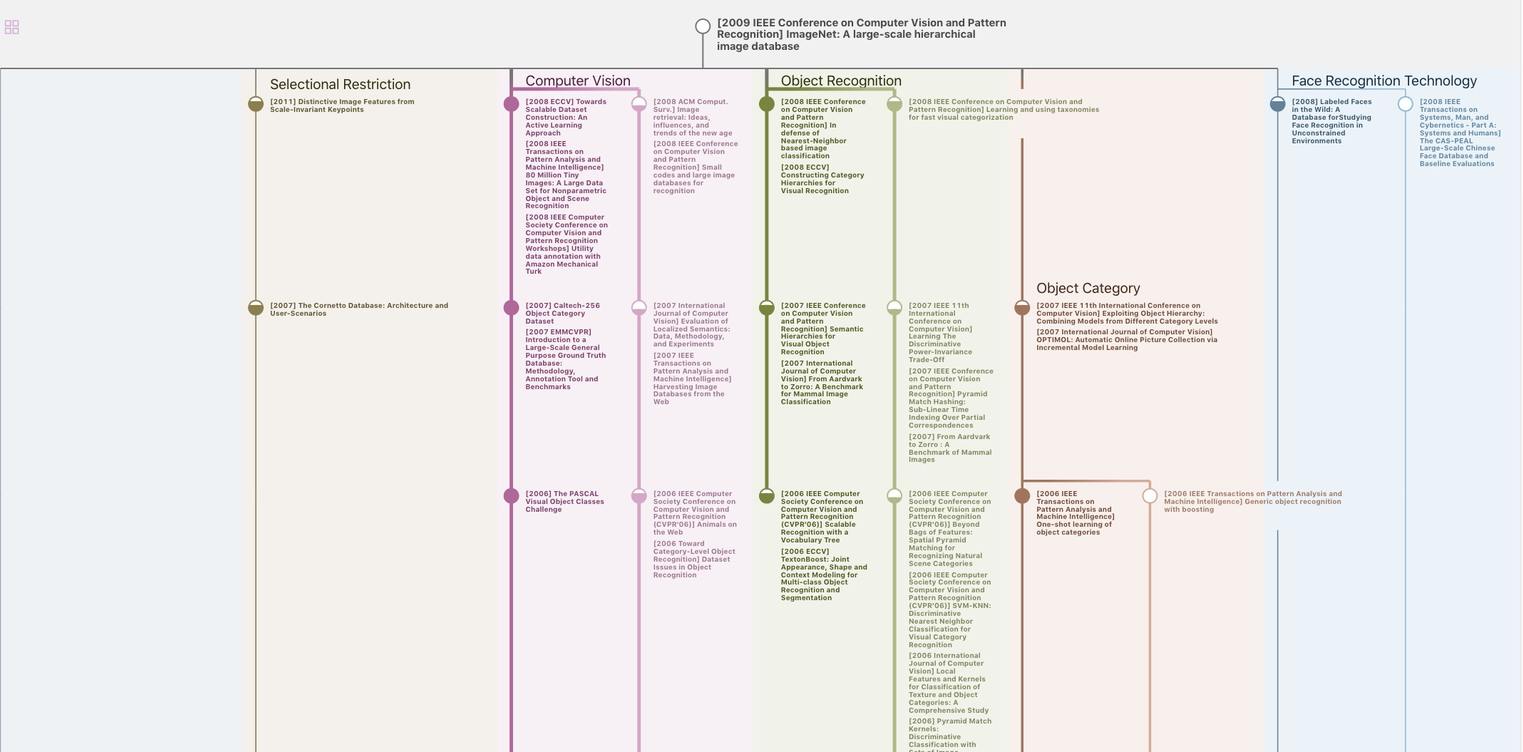
生成溯源树,研究论文发展脉络
Chat Paper
正在生成论文摘要