Find Your Friends: Personalized Federated Learning with the Right Collaborators
arxiv(2022)
摘要
In the traditional federated learning setting, a central server coordinates a network of clients to train one global model. However, the global model may serve many clients poorly due to data heterogeneity. Moreover, there may not exist a trusted central party that can coordinate the clients to ensure that each of them can benefit from others. To address these concerns, we present a novel decentralized framework, FedeRiCo, where each client can learn as much or as little from other clients as is optimal for its local data distribution. Based on expectation-maximization, FedeRiCo estimates the utilities of other participants' models on each client's data so that everyone can select the right collaborators for learning. As a result, our algorithm outperforms other federated, personalized, and/or decentralized approaches on several benchmark datasets, being the only approach that consistently performs better than training with local data only.
更多查看译文
关键词
Federated learning,Personalized federated learning,Decentralized federated learning
AI 理解论文
溯源树
样例
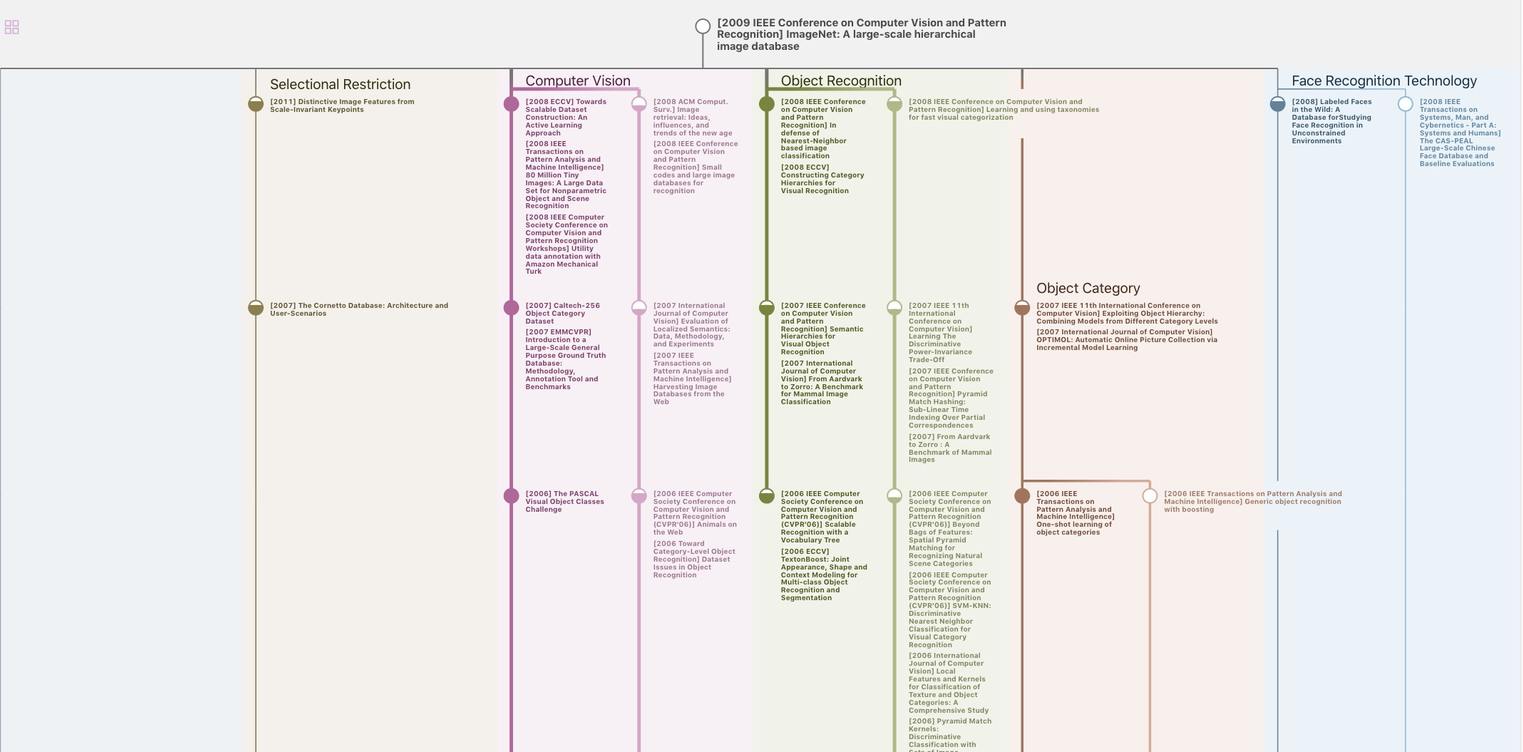
生成溯源树,研究论文发展脉络
Chat Paper
正在生成论文摘要