Uncertainty quantification of metallic microstructures using principal image moments
Computational Materials Science(2022)
Abstract
The present work uses Markov Random Field (MRF) algorithm to construct large-scale and statistically-equivalent samples from small-scale experimental data of metallic microstructures. While the MRF method can build such digital material representations in large computational domains, its algorithmic stochasticity (epistemic uncertainty) causes variations in the resulting microstructural features, such as the texture and grain topology. This work addresses the effects of the epistemic uncertainty on homogenized mechanical properties by characterizing the variations in the microstructural features using a shape descriptor based on the concept of moment invariants. In particular, 2D and 3D synthetic microstructure data for Titanium-7wt%Aluminum (Ti-7Al) alloy is generated with the MRF method using smaller-scale 2D experimental data. To quantify the uncertainty of the reconstructed synthetic samples, a graphical method and five different metrics of statistical variability are developed. Next, the propagation of the microstructural uncertainty on homogenized properties is studied using an analytical uncertainty quantification (UQ) algorithm and Gaussian Process Regression (GPR).
MoreTranslated text
Key words
Microstructure reconstruction,Uncertainty quantification,Shape moment,Gaussian process
AI Read Science
Must-Reading Tree
Example
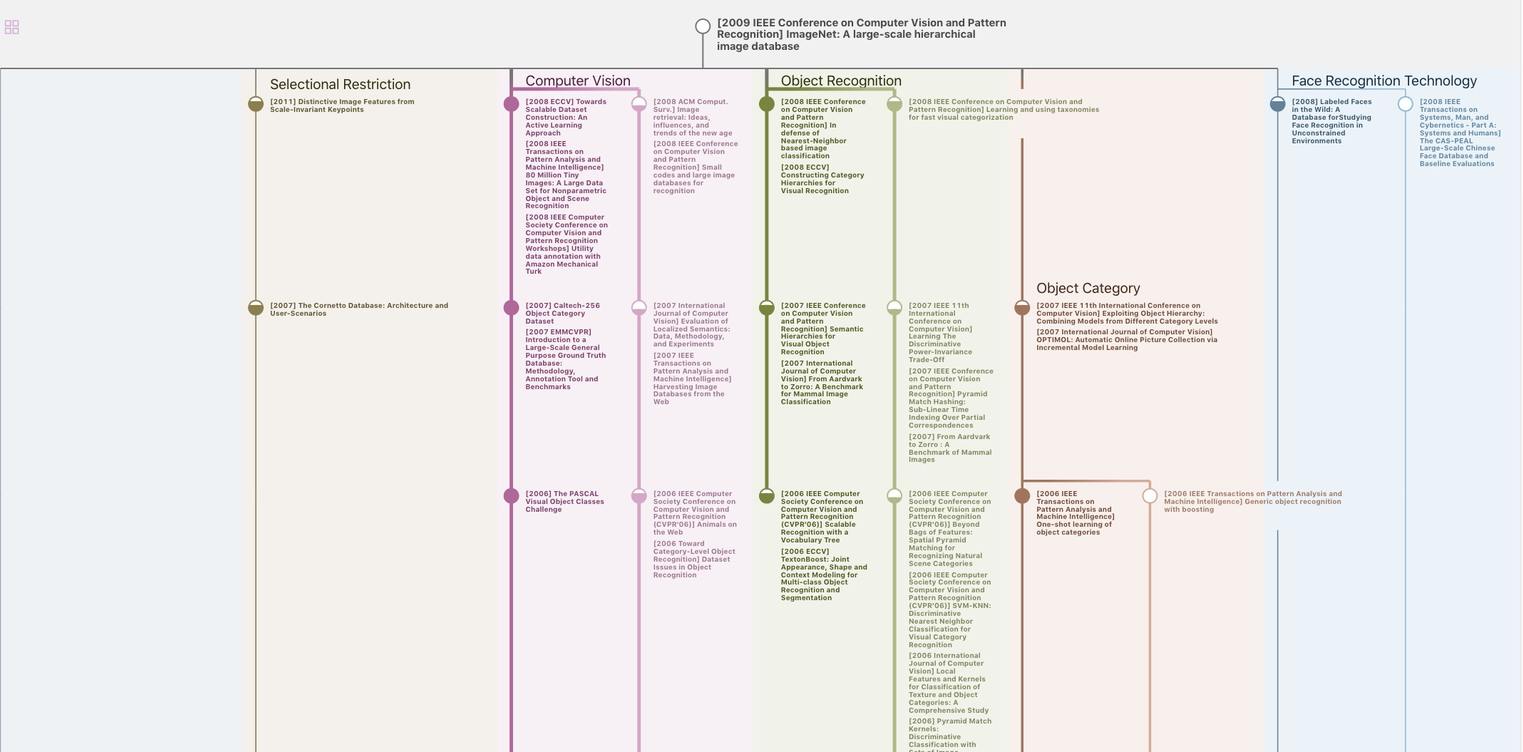
Generate MRT to find the research sequence of this paper
Chat Paper
Summary is being generated by the instructions you defined