Swarm intelligence for new materials
Computational Materials Science(2022)
摘要
With the help of deep learning technology, constructing the neural network model between structures and properties of materials is one of important methods to speed up the new materials discovery and design. However, both the training efficiency and accuracy of model play important roles in application of machine learning on materials. At present, the commonly used traditional neural network model algorithm in materials is single, easy to fall into local optimization, and difficult to converge in the training process, and the training model has a low prediction power. In this paper, a novel neural network optimization method based on swarm algorithms is proposed to discover better parameters in developing new materials. In this neural network model, particle swarm and Bayesian optimization are used to improve the crossover, mutation and selection strategies of genetic algorithm. Using advantages of each algorithm to realize the optimal parameters selection of the neural network and network optimization, and to improve the prediction ability of the neural network model. By comparing energies predicted by traditional networks and the proposed network, more stable models with high efficiency and precision are trained.
更多查看译文
关键词
Neural network model,Hybrid algorithm,Swarm intelligence,Network optimization,Material prediction
AI 理解论文
溯源树
样例
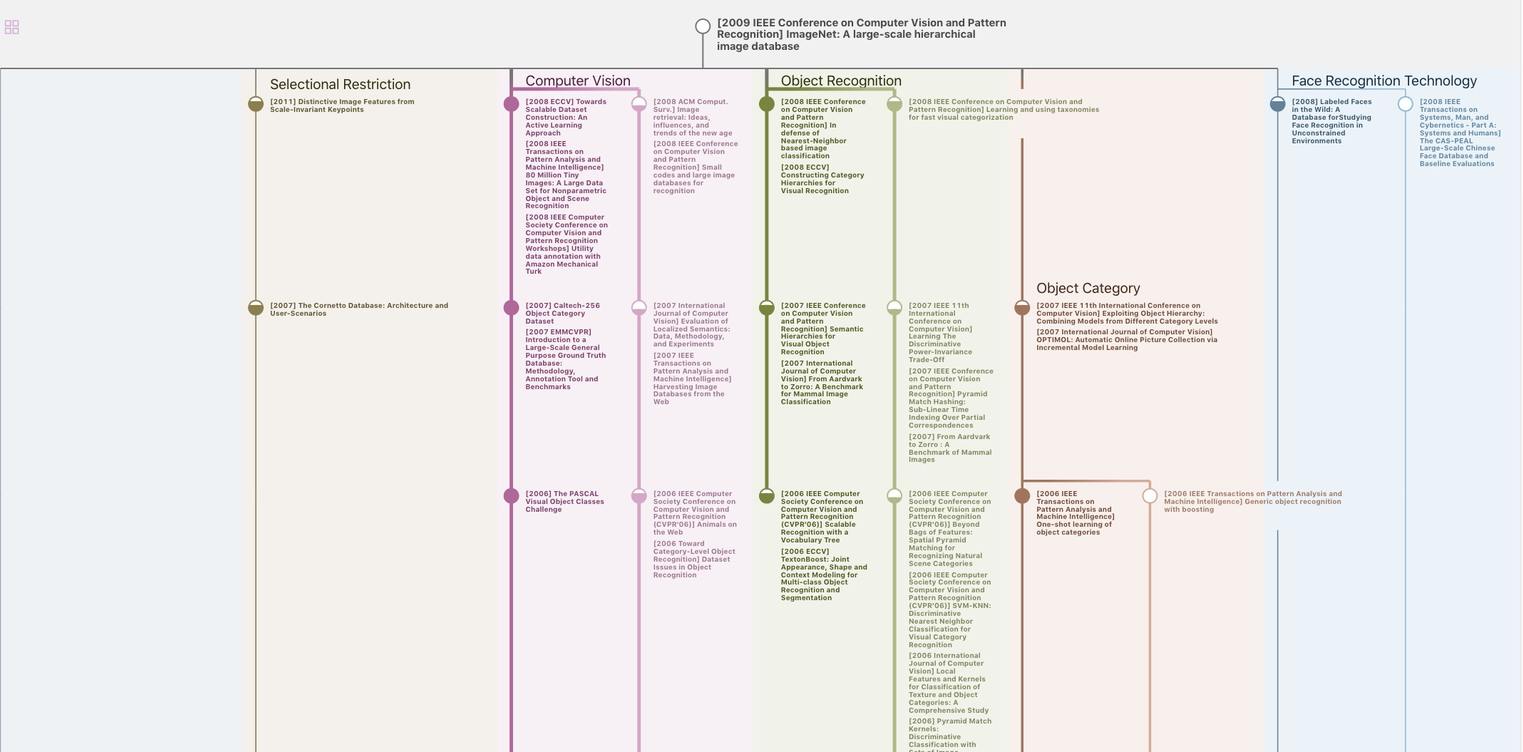
生成溯源树,研究论文发展脉络
Chat Paper
正在生成论文摘要