Scheduling single-satellite observation and transmission tasks by using hybrid Actor-Critic reinforcement learning
Advances in Space Research(2022)
摘要
Earth observation satellites(EOS) generate a large amount of observation data in intensive observation scenarios, while the ability of data storage on the EOS is limited. It makes integrating satellite observation and data transmission tasks for EOS imperative. This paper establishes a time-continuous model for the single EOS integrated scheduling problem, which considers data transmission and observa-tion simultaneously. A hybrid Actor-Critic reinforcement learning method is adopted to solve the EOS integrated scheduling problem for a more efficient solution in intensive observation scenarios. Furthermore, the algorithm can flexibly determine the start and end time of the data transmission task. Experimental results show that the hybrid Actor-Critic reinforcement learning method deals with a large scale of problems with high efficiency and good results. (c) 2022 COSPAR. Published by Elsevier B.V. All rights reserved.
更多查看译文
关键词
Earth observation satellite,Integrated scheduling problem,Deep-reinforcement learning
AI 理解论文
溯源树
样例
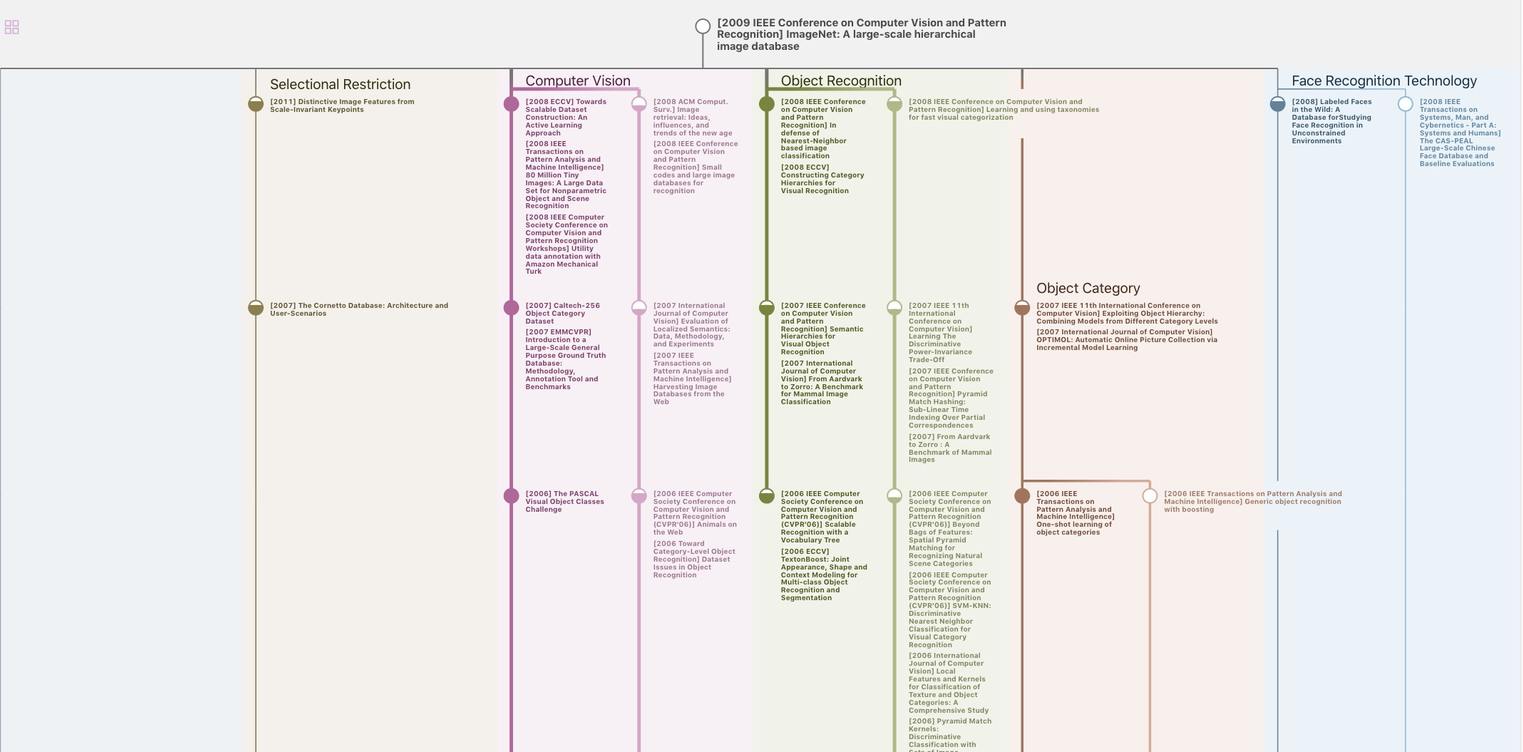
生成溯源树,研究论文发展脉络
Chat Paper
正在生成论文摘要