CSMOTE: Contrastive Synthetic Minority Oversampling for Imbalanced Time Series Classification.
International Conference on Neural Information Processing(2021)
摘要
The class imbalanced classification is pervasive in real-world applications for a long time, such as image, tabular, textual, and video data analysis. The imbalance issue of time series data attracts especial attention recently, with the development of the Industrial Internet. The Oversampling method is one of the most popular techniques, which usually heuristically re-establishes the balance of the dataset, i.e., interpolation or adversarial generative technology for minority class instances augmentation. However, the high dimensional and temporal dependence characteristics pose great challenge to time series minority oversampling. To this end, this paper proposes a Contrastive Synthetic Minority Oversampling (CSMOTE) for imbalanced time series classification. Specifically, we assume that the minority class example is composed of its peculiar private information and common information shared with majority classes. According to the variational Bayes technology, we encode this information into two separated Gaussian latent spaces. The minority class synthetic instances are generated from the combination of private and common representation draws from the two latent spaces. We evaluate CSMOTE’s performance on five real-world benchmark datasets, and it outperforms other oversampling baselines in most of the cases.
更多查看译文
关键词
contrastive synthetic minority oversampling,classification
AI 理解论文
溯源树
样例
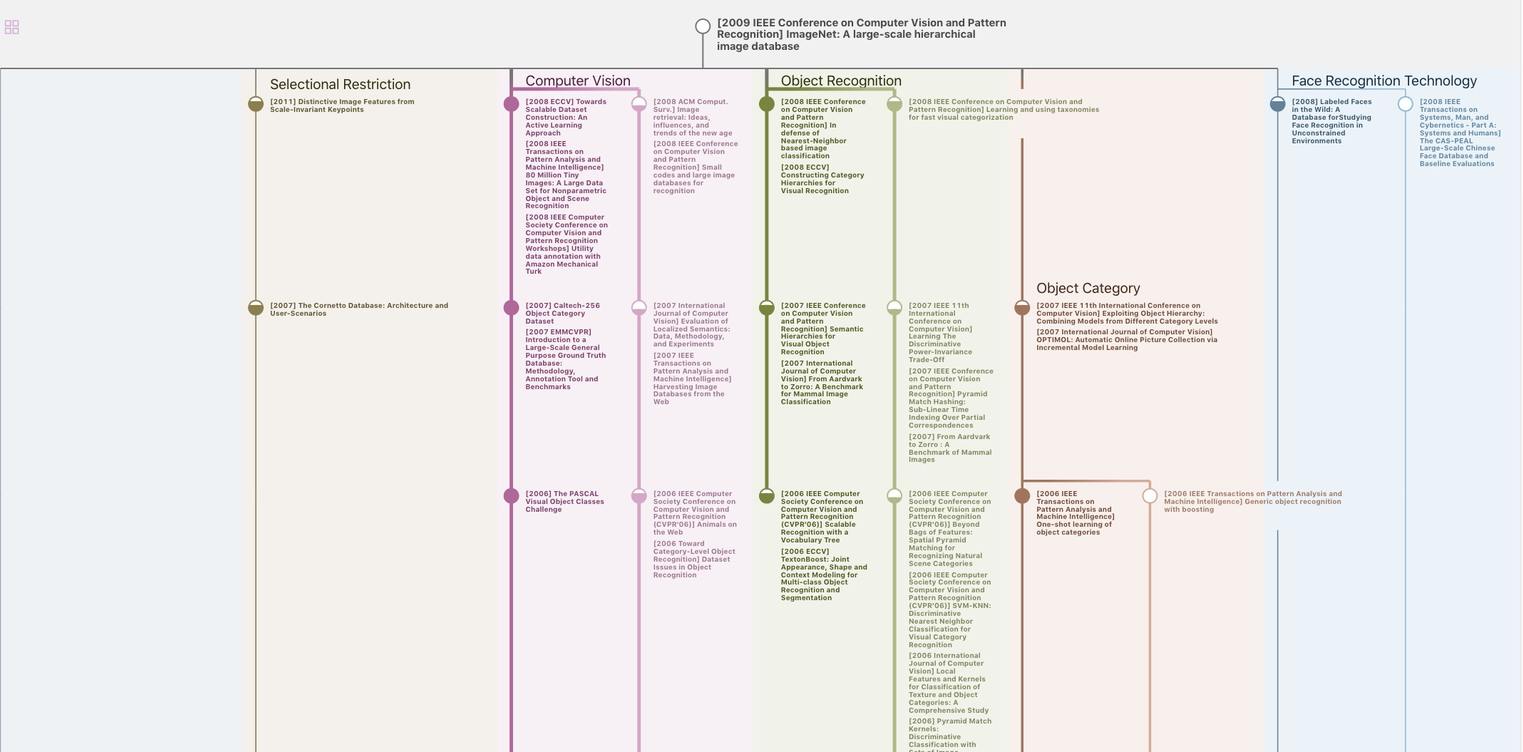
生成溯源树,研究论文发展脉络
Chat Paper
正在生成论文摘要