Deep Learning-driven Proactive Maintenance Management of IoT-empowered Smart Toilet
IEEE Internet of Things Journal(2022)
摘要
The recent proliferation of Internet of Things (IoT) sensors has driven a myriad of industrial and urban applications. Through analyzing massive data collected by these sensors, the proactive maintenance management can be achieved such that the maintenance schedule of the installed equipment can be optimized. Despite recent progress in proactive maintenance management in industrial scenarios, there are few studies on proactive maintenance management in urban informatics. In this article, we present an integrated framework of IoT and cloud computing platform for the proactive maintenance management in smart city. Our framework consists of: 1) an IoT monitoring system for collecting time-series data of operating and ambient conditions of the equipment and 2) a hybrid deep learning model, namely, convolutional bidirectional long short-term memory (CBLM) model for forecasting the operating and ambient conditions based on the collected time-series data. In addition, we also develop a naïve Bayes classifier to detect abnormal operating and ambient conditions and assist management personnel in scheduling maintenance tasks. To evaluate our framework, we deployed the IoT system in a Hong Kong public toilet, which is the first application of proactive maintenance management for a public hygiene and sanitary facility to the best of our knowledge. We collected the sensed data more than 33 days (808 h) in this real system. Extensive experiments on the collected data demonstrated that our proposed CBLM outperformed six traditional machine learning algorithms.
更多查看译文
关键词
Cloud computing,convolutional neural network (CNN),deep learning,Internet of Things (IoT),long short-term memory (LSTM),machine learning,proactive maintenance management
AI 理解论文
溯源树
样例
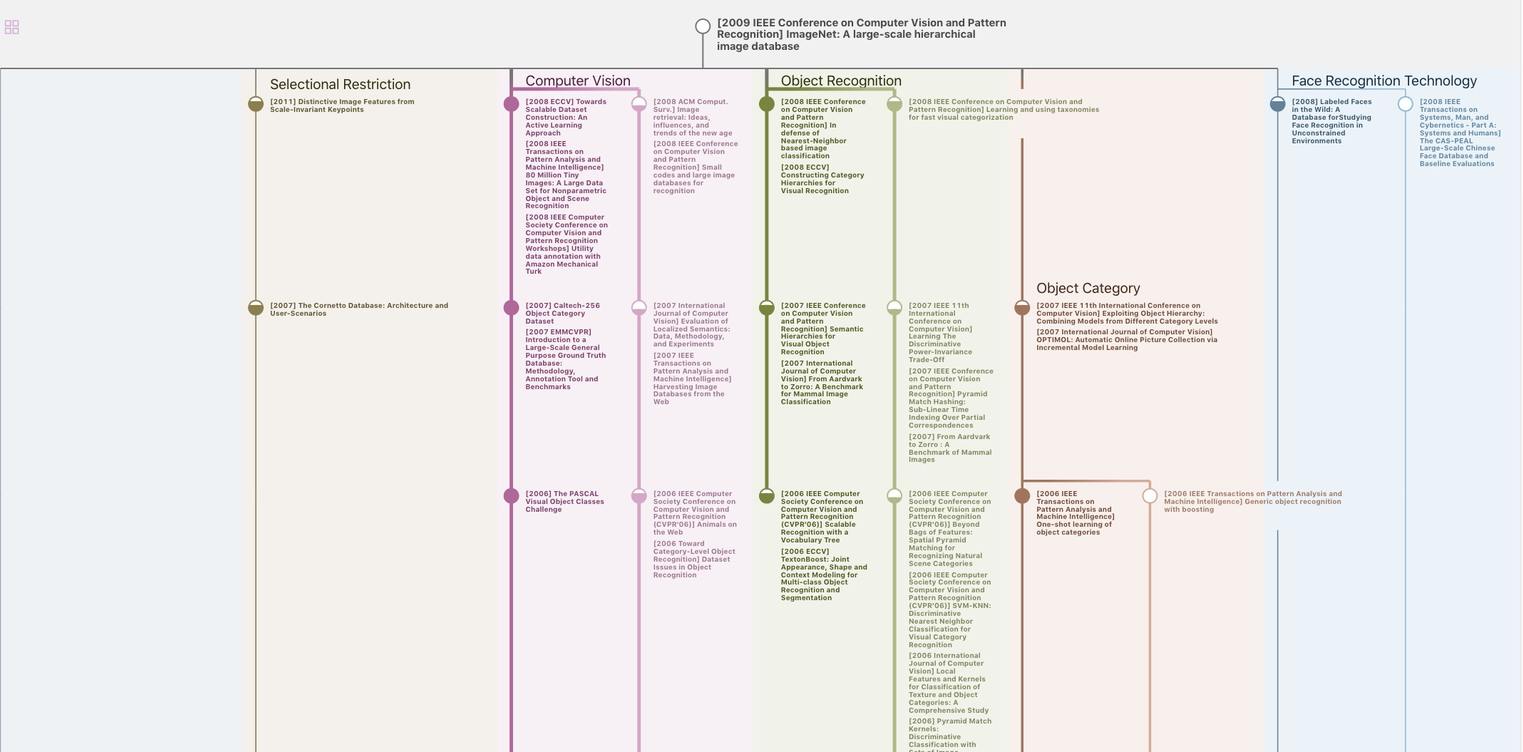
生成溯源树,研究论文发展脉络
Chat Paper
正在生成论文摘要