Alzheimer's Disease Prediction Algorithm Based on Group Convolution and a Joint Loss Function.
COMPUTATIONAL AND MATHEMATICAL METHODS IN MEDICINE(2022)
摘要
Alzheimer's disease (AD) can effectively predict by 18F-fluorodeoxyglucose positron emission tomography (18F-FDG PET) of the brain, but current PET images still suffer from indistinct lesion features, low signal-to-noise ratios, and severe artefacts, resulting in poor prediction accuracy for patients with mild cognitive impairment (MCI) and unclear lesion features. In this paper, an AD prediction algorithm based on group convolution and a joint loss function is proposed. First, a group convolutional backbone network based on ResNet18 is designed to extract lesion features from multiple channels, which makes the expression ability of the network improved to a great extent. Then, a hybrid attention mechanism is presented, which enables the network to focus on target regions and learn feature weights, so as to enhance the network's learning ability of the lesion regions that are relevant to disease diagnosis. Finally, a joint loss function, that avoids the overfitting phenomenon, increases the generalization of the model, and improves prediction accuracy by adding a regularization loss function to the conventional cross-entropy function, is proposed. Experiments conducted on the public Alzheimer's Disease Neuroimaging Initiative (ADNI) dataset show that the algorithm we proposed gives a prediction accuracy improvement of 2.4% over that of the current AD prediction algorithm, thus proving the effectiveness and availability of the new algorithm.
更多查看译文
关键词
group convolution,alzheimers,disease prediction algorithm
AI 理解论文
溯源树
样例
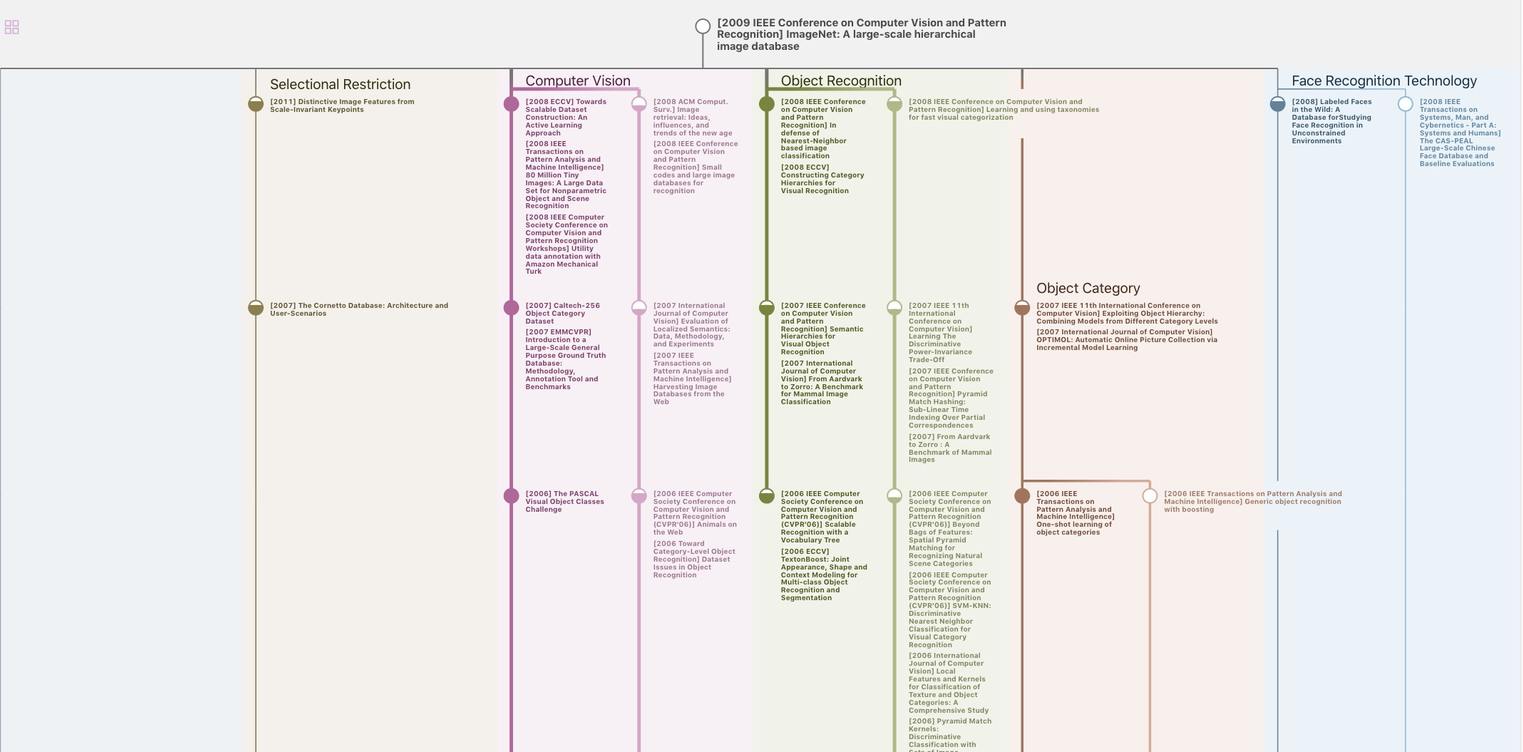
生成溯源树,研究论文发展脉络
Chat Paper
正在生成论文摘要