Fast Convergence of Random Reshuffling Under Over-Parameterization and the Polyak-Lojasiewicz Condition
arXiv (Cornell University)(2023)
Abstract
Modern machine learning models are often over-parameterized and as a result they can interpolate the training data. Under such a scenario, we study the convergence properties of a sampling-without-replacement variant of stochastic gradient descent (SGD) known as random reshuffling (RR). Unlike SGD that samples data with replacement at every iteration, RR chooses a random permutation of data at the beginning of each epoch and each iteration chooses the next sample from the permutation. For under-parameterized models, it has been shown RR can converge faster than SGD under certain assumptions. However, previous works do not show that RR outperforms SGD in over-parameterized settings except in some highly-restrictive scenarios. For the class of Polyak-Lojasiewicz (PL) functions, we show that RR can outperform SGD in over-parameterized settings when either one of the following holds: (i) the number of samples (n) is less than the product of the condition number (kappa) and the parameter (alpha) of a weak growth condition (WGC), or (ii) n is less than the parameter (rho) of a strong growth condition (SGC).
MoreTranslated text
Key words
random reshuffling,fast convergence,over-parameterization
AI Read Science
Must-Reading Tree
Example
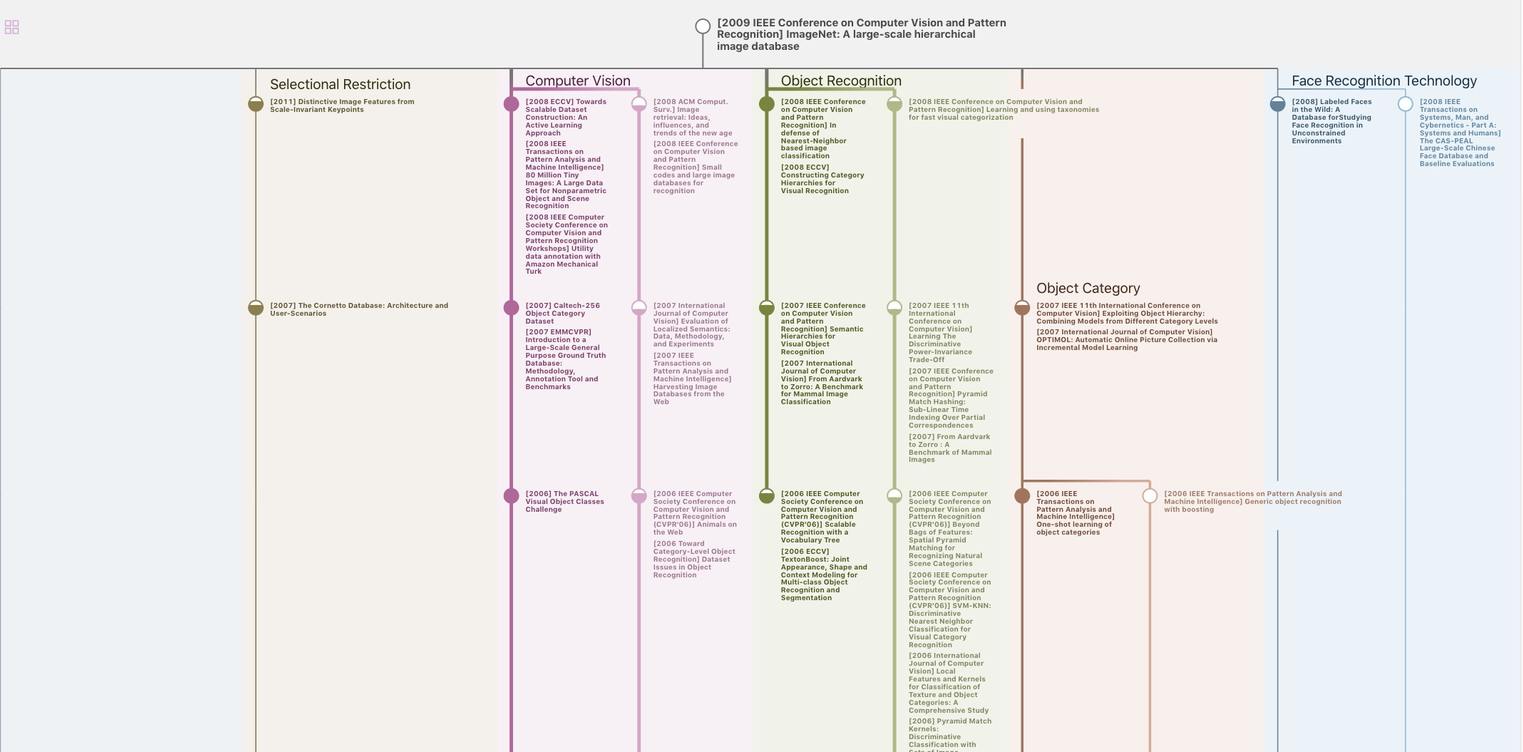
Generate MRT to find the research sequence of this paper
Chat Paper
Summary is being generated by the instructions you defined