Lifespan prognostics for lithium-ion batteries using Long Short Term Memory
2022 Prognostics and Health Management Conference (PHM-2022 London)(2022)
摘要
Lithium battery safety accidents occur frequently, and its lifespan prognostics has become a research focus at home and abroad, but it is still very challenging to accurately predict the lifespan of lithium battery. Approaches, which using machine learning techniques, are becoming more and more attractive to predict lifespan. In this study, a method based on a sparse autoencoder (SAE) and a long short term memory (LSTM) is developed for improving lifespan prognostics performance, using only previous capacity measurements. The SAE was firstly used to extract temporal features within a fragment of previous capacity measurements. LSTM was then used to fuse the extracted information with the previous input information and the current input and output ones, so as to obtain accurate lifespan prognostics. The proposed method’s performance is tested on a benchmark lithium-ion battery degradation dataset. The results show that it can accurately predict lifespan of batteries.
更多查看译文
关键词
machine learning, lifespan prognostics, feature extraction, long short term memory
AI 理解论文
溯源树
样例
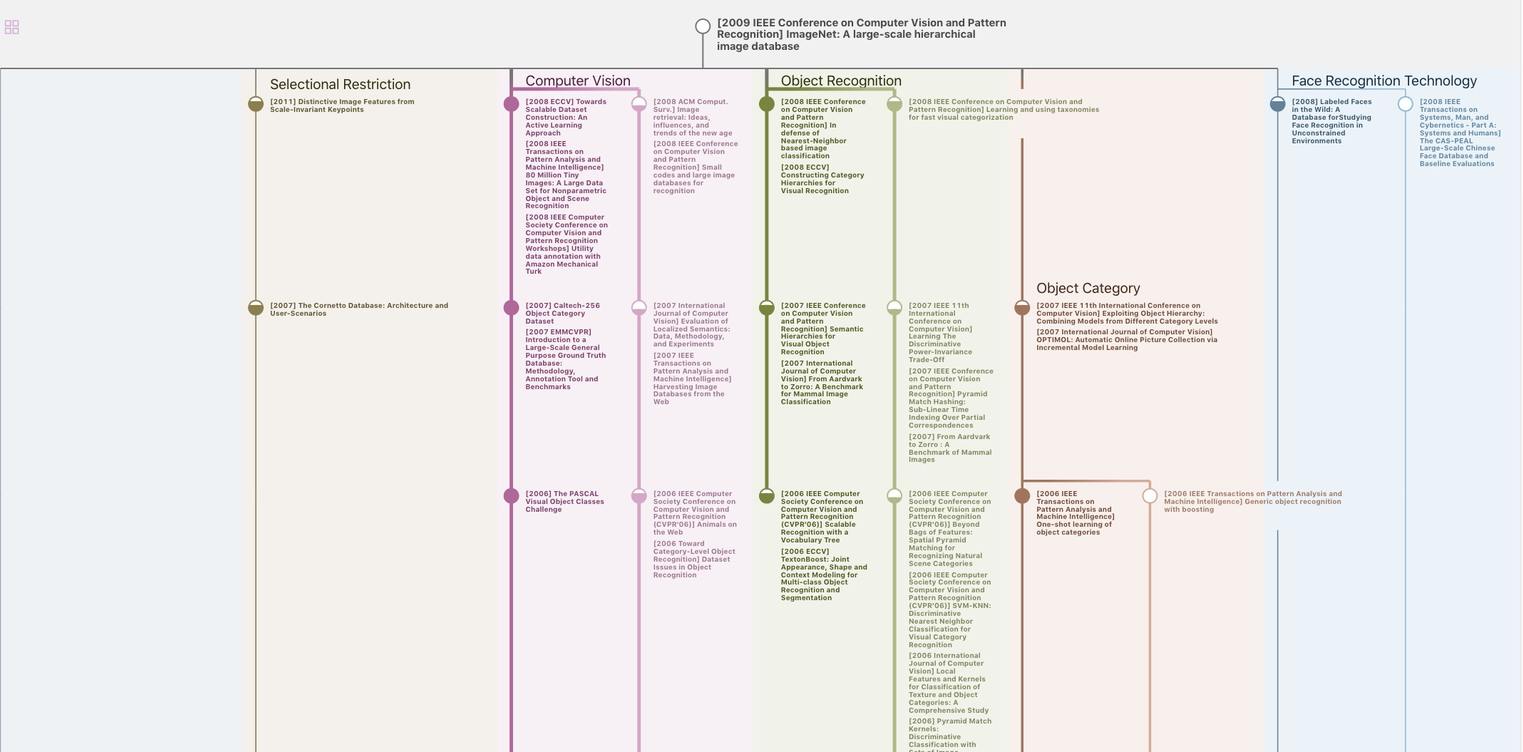
生成溯源树,研究论文发展脉络
Chat Paper
正在生成论文摘要