Neuromorphic Spectrum Occupancy Learning in Cognitive Cloud Radio Access System
2022 IEEE 7th International conference for Convergence in Technology (I2CT)(2022)
摘要
This paper presents a neuromorphic spectrum occupancy learning using convolutional neural networks and long short-term memory, in a cognitive radio enabled cloud radio access system. Cloud radio access networks can prove useful in future generation wireless communication systems, because of their distributed signal processing capability. Owing to its excellent noise immunity property, cyclostationarity-based spectrum sensing is utilized as the method to detect the active sub-bands at the baseband unit. However, the accuracy of sensing is corrupted due to impairments, such as chromatic dispersion, caused by the optical fiber front-haul channel. Hence, application of deep learning techniques like convolutional neural networks and long short-term memory are necessary to compensate for the front-haul channel. Our simulation results indicate that the proposed method can lead to improved signal detection in presence of optical fiber impairment in cloud radio system.
更多查看译文
关键词
Cyclostationarity,Cloud Radio Access Network,Active Interference Cancellation,Deep Learning,Fast Convolution Filter Bank
AI 理解论文
溯源树
样例
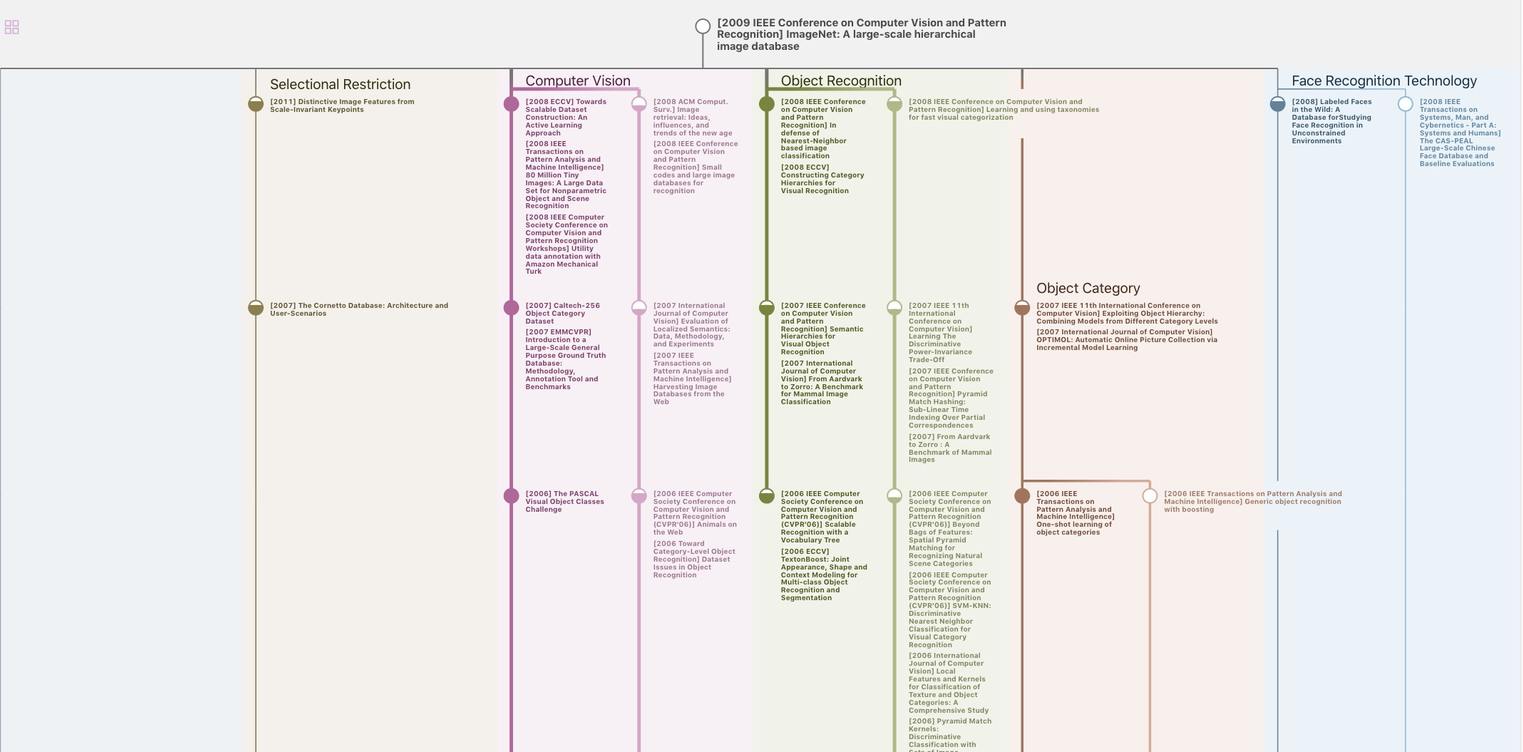
生成溯源树,研究论文发展脉络
Chat Paper
正在生成论文摘要