DCR: Dual Compression Method for Traffic Signs Recognition and Embedded Deployment
2022 7th International Conference on Computational Intelligence and Applications (ICCIA)(2022)
摘要
In order to solve the problems of large memory consumption and complex model of CNN in traffic signs recognition, we propose an improved deep learning method DCR (Dual Compression Recognition) based on BNN (Binary Neural Network) model. We compress BNN's network structure and dataset (remove the color information on the dataset). Experiments show that the DCR model with only one binary convolutional layer is enough to achieve about 75.18% accuracy on the GTSRB dataset, while the trainable parameters are only 1.4M and the model memory is only 16.1M. Moreover, the DCR model with three binary convolutional layers has an accuracy comparable to that of the classical CNN's models, with an accuracy of 92.86%, while the trainable parameters is only 0.83M and the model memory is only 9.60M. As a result, most arithmetic operations have been simplified, and the memory access and memory size have been reduced by 32 times. In addition, because the color information of the dataset is removed, the training speed of the model is effectively improved. All these make our model more suitable for embedded deployment with lower cost.
更多查看译文
关键词
traffic signs recognition,embedded deployment,binary neural networks,image compression,model compression
AI 理解论文
溯源树
样例
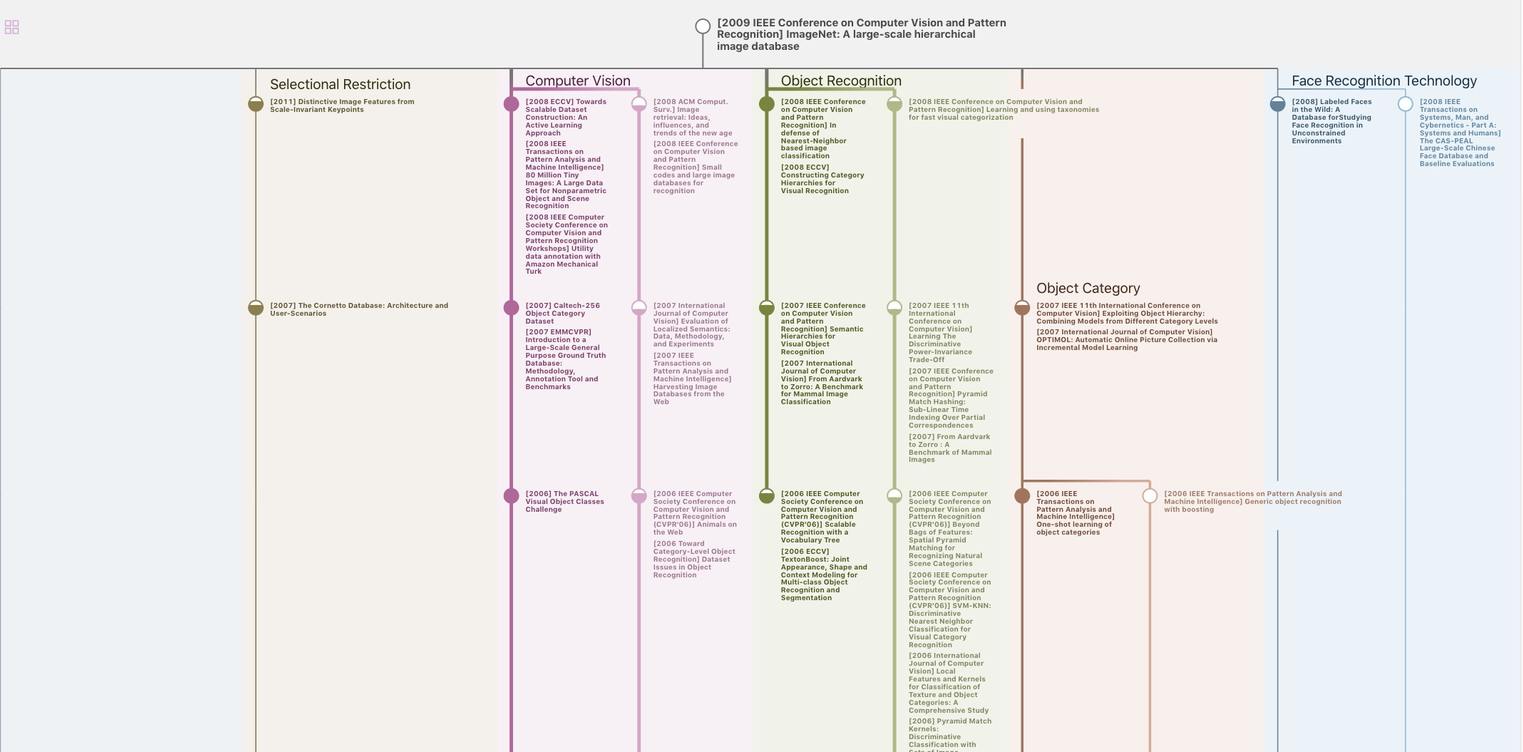
生成溯源树,研究论文发展脉络
Chat Paper
正在生成论文摘要