Categorization of Product Feedback using a Deep CNN-based LSTM Model and a Comparative Study of Traditional ML Algorithms
2022 IEEE 7th International conference for Convergence in Technology (I2CT)(2022)
摘要
In this era, especially in the pandemic, people and businesses have shifted towards digital platforms from analog ones. These changes have resulted in a blast of information in the marketing sector that needs to be categorized and summarized. Feedback has a significant role in drawing the public towards standard and high-quality products. However, due to the abundance of information, most businesses can’t quickly determine the market value of their products. This is the point where sentimental analysis comes in, which helps in classifying positive and negative feedback. In this paper, the deep learning methods based on CNN and LSTM (Long Short Term Memory) are proposed and trained with the data set from IMDb. In addition, the well-known algorithms of machine learning methods are reviewed based on sentiment analysis. The majority of traditional methods are accurate within a margin of error of 75 to 86 percent. In our experiment, LSTM and CNN showed almost the same accuracy of 89% while the proposed one obtained 92% accuracy. It is evident from the result that, the proposed fusion model outperforms the conventional machine learning algorithms. By deploying the methods with better accuracy, the reviews could be categorized easily and the businesses could stay one step ahead of their competitors.
更多查看译文
关键词
Sentimental Analysis,Machine Learning,Deep Learning,Natural Language Processing,CNN,LSTM,Word Embedding
AI 理解论文
溯源树
样例
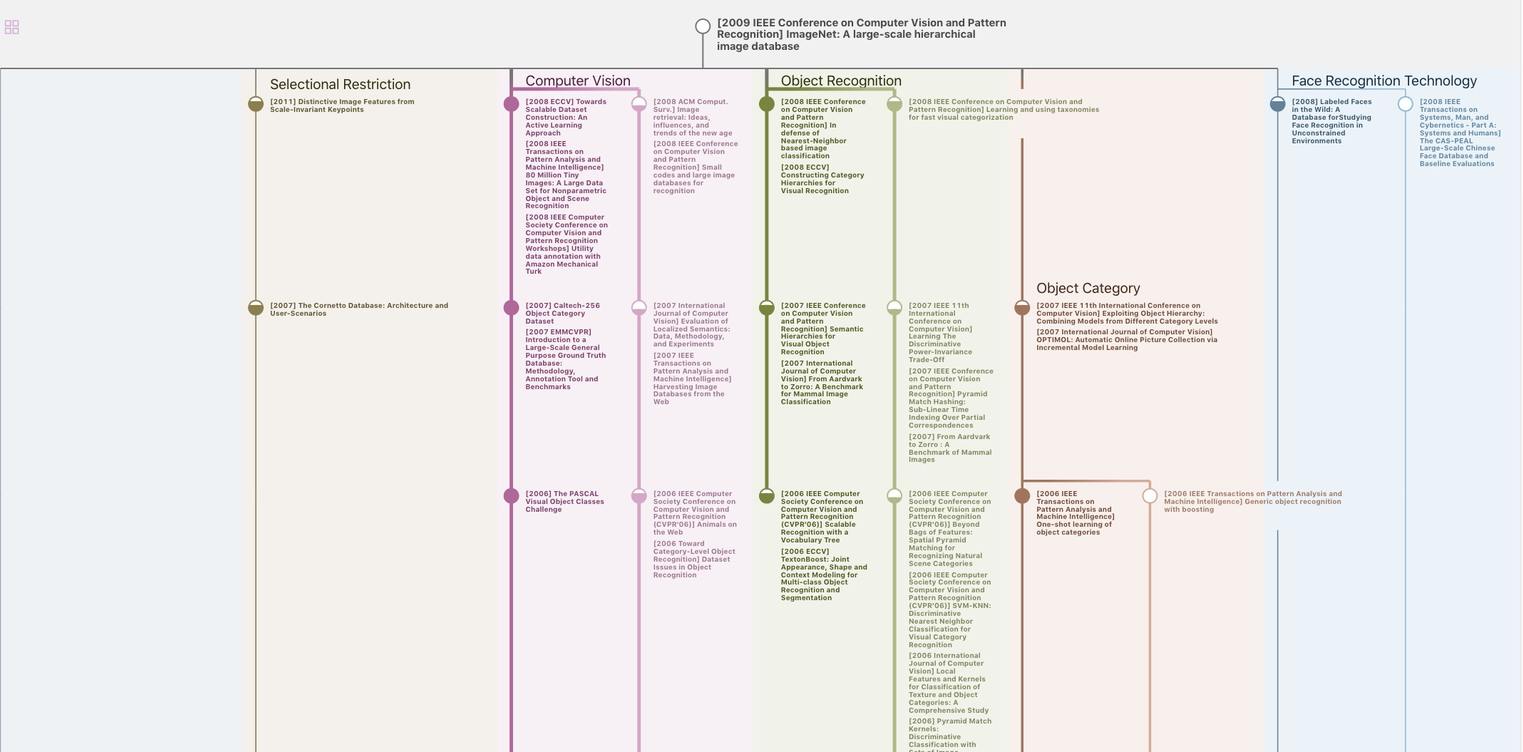
生成溯源树,研究论文发展脉络
Chat Paper
正在生成论文摘要