A Fusion Neural Network Incorporating Attention for Sensor-Based Human Activity Recognition
2022 3rd International Conference on Computer Vision, Image and Deep Learning & International Conference on Computer Engineering and Applications (CVIDL & ICCEA)(2022)
摘要
Even though the RNN, LSTM, and other networks are used to extract dependencies in time series, sensor-based human behavior recognition (HAR) still faces some difficulties, and the ability of deep learning (DL) networks to extract features still needs to be improved. We propose a fusion neural network in which an optimized small optimized Convolutional Block Attention Module (MP-CBAM) is suitable for HAR tasks to extract samples. The MP-CBAM is added to two branches Convolutional Neural Network (CNN) with different convolution kernel sizes, and the fused features are labeled with GRU for temporal dependence. Then the softmax function is used for classification. We validate on benchmark datasets UCI-HAR and WISDM and accuracies of 97.15% and 9S.9S% are obtained, respectively, demonstrating the adaptation of our framework to both single-sensor and multi-sensor devices.
更多查看译文
AI 理解论文
溯源树
样例
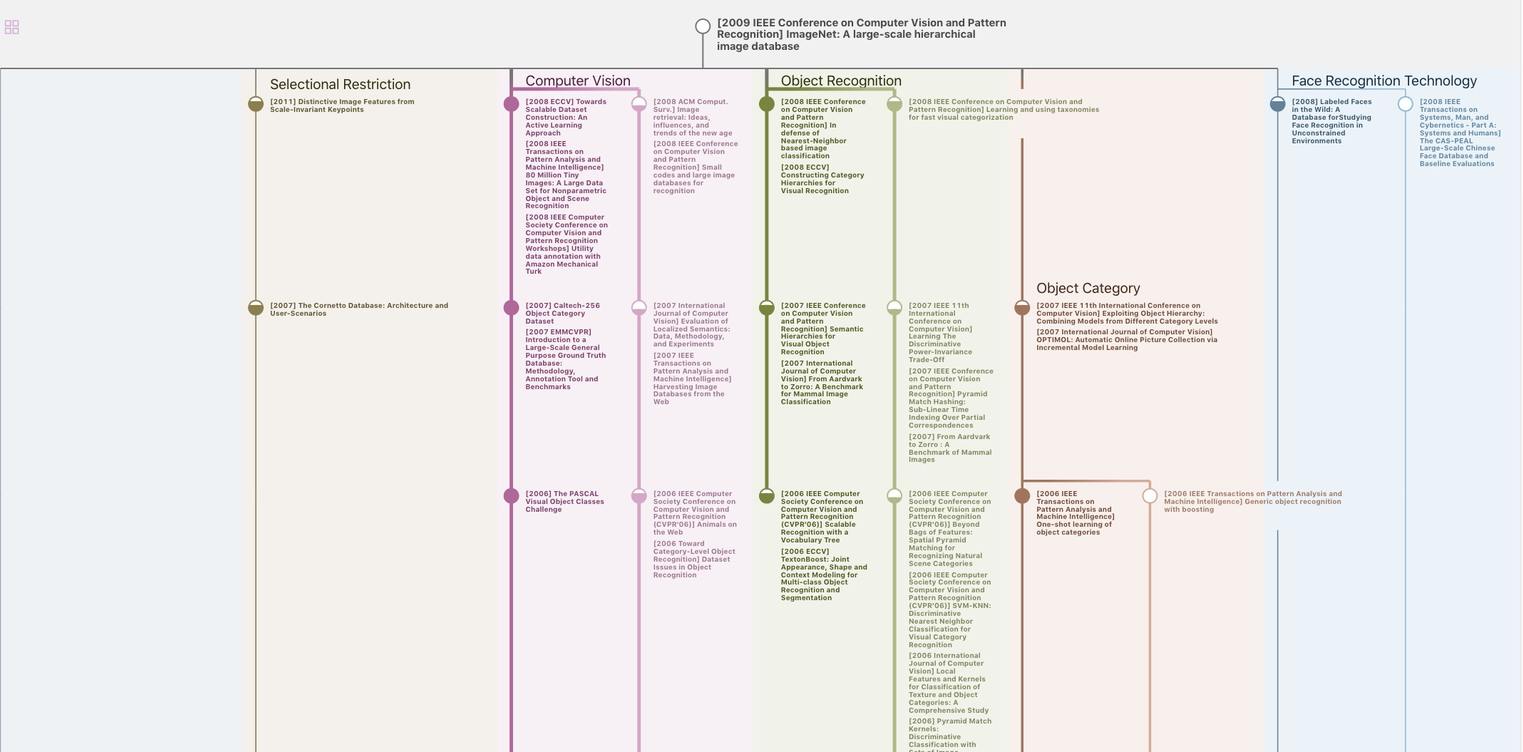
生成溯源树,研究论文发展脉络
Chat Paper
正在生成论文摘要