Mechanisms of adjustments to different types of uncertainty in the reward environment across mice and monkeys
Cognitive, affective & behavioral neuroscience(2023)
摘要
Despite being unpredictable and uncertain, reward environments often exhibit certain regularities, and animals navigating these environments try to detect and utilize such regularities to adapt their behavior. However, successful learning requires that animals also adjust to uncertainty associated with those regularities. Here, we analyzed choice data from two comparable dynamic foraging tasks in mice and monkeys to investigate mechanisms underlying adjustments to different types of uncertainty. In these tasks, animals selected between two choice options that delivered reward probabilistically, while baseline reward probabilities changed after a variable number (block) of trials without any cues to the animals. To measure adjustments in behavior, we applied multiple metrics based on information theory that quantify consistency in behavior, and fit choice data using reinforcement learning models. We found that in both species, learning and choice were affected by uncertainty about reward outcomes (in terms of determining the better option) and by expectation about when the environment may change. However, these effects were mediated through different mechanisms. First, more uncertainty about the better option resulted in slower learning and forgetting in mice, whereas it had no significant effect in monkeys. Second, expectation of block switches accompanied slower learning, faster forgetting, and increased stochasticity in choice in mice, whereas it only reduced learning rates in monkeys. Overall, while demonstrating the usefulness of metrics based on information theory in examining adaptive behavior, our study provides evidence for multiple types of adjustments in learning and choice behavior according to uncertainty in the reward environment.
更多查看译文
关键词
Value-based decision making,Risk,Uncertainty,Flexibility,Information theory
AI 理解论文
溯源树
样例
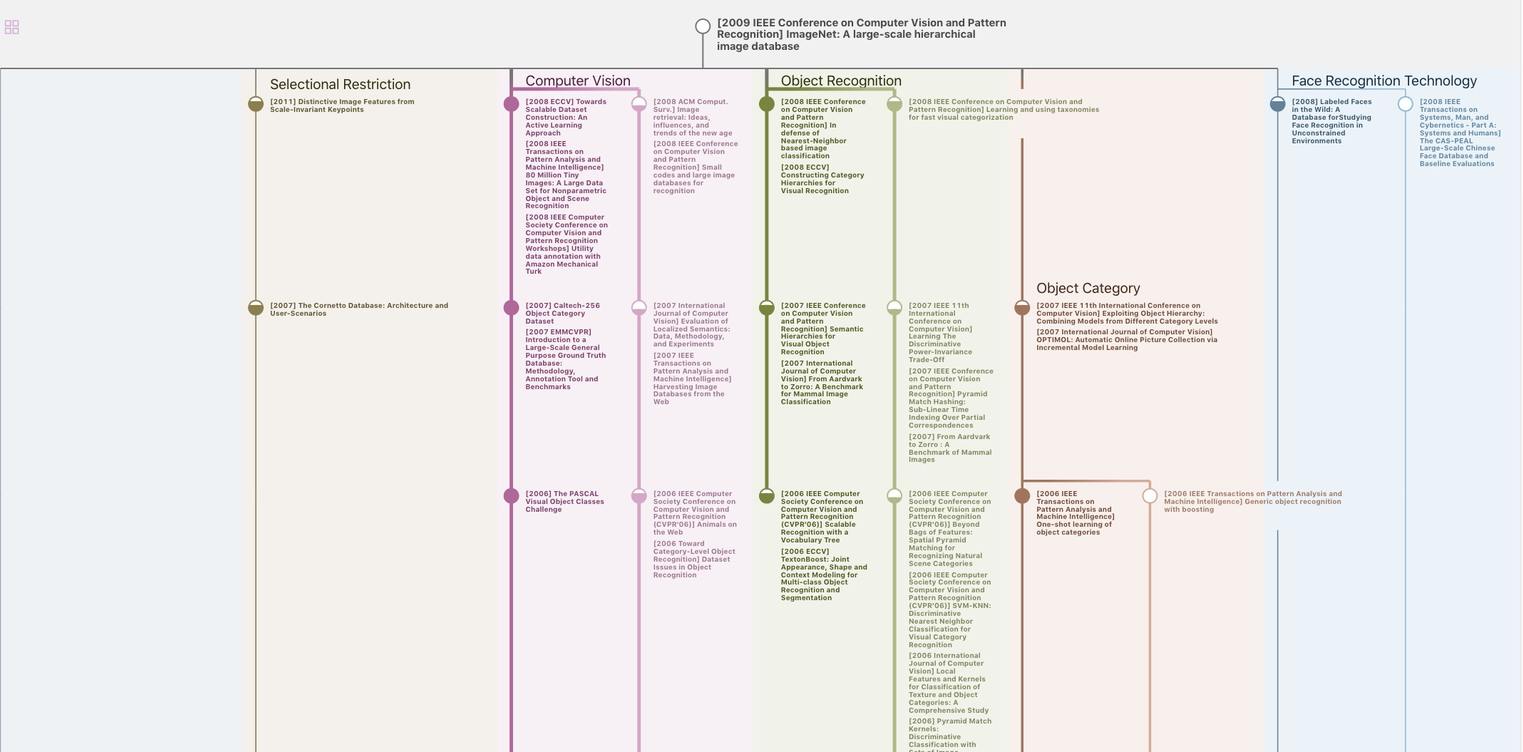
生成溯源树,研究论文发展脉络
Chat Paper
正在生成论文摘要