Machine learning-assisted design of porous carbons for removing paracetamol from aqueous solutions
Carbon(2022)
摘要
To accelerate the design and production of porous carbons targeting desired performance characteristics, we propose to incorporate machine learning (ML) regression into pore size distribution (PSD) analysis. Here, we implemented a ML algorithm for predicting paracetamol adsorption capacity of porous carbons from two pore structure parameters: total surface area and surface area of supermicropores-mesopores. These structural parameters of porous carbons are accessible from the software provided with automatic volumetric gas adsorption analyzers. It was shown that theoretical paracetamol capacities of porous carbons predicted using the ML algorithm lies within the range of experimental uncertainty. Nanoporous carbon beads with a high surface area of supermicropores (997 m 2 /g) and mesopores (628 m 2 /g) had the highest adsorption capacity of paracetamol (experiment: 480 ± 24 mg/g, ML predicted: 498 mg/g). The novel strategy for designing of porous carbon adsorbents using ML-PSD approach has a great potential to facilitate production of novel carbon adsorbents optimized for purification of aqueous solutions from non-electrolyte contaminates.
更多查看译文
AI 理解论文
溯源树
样例
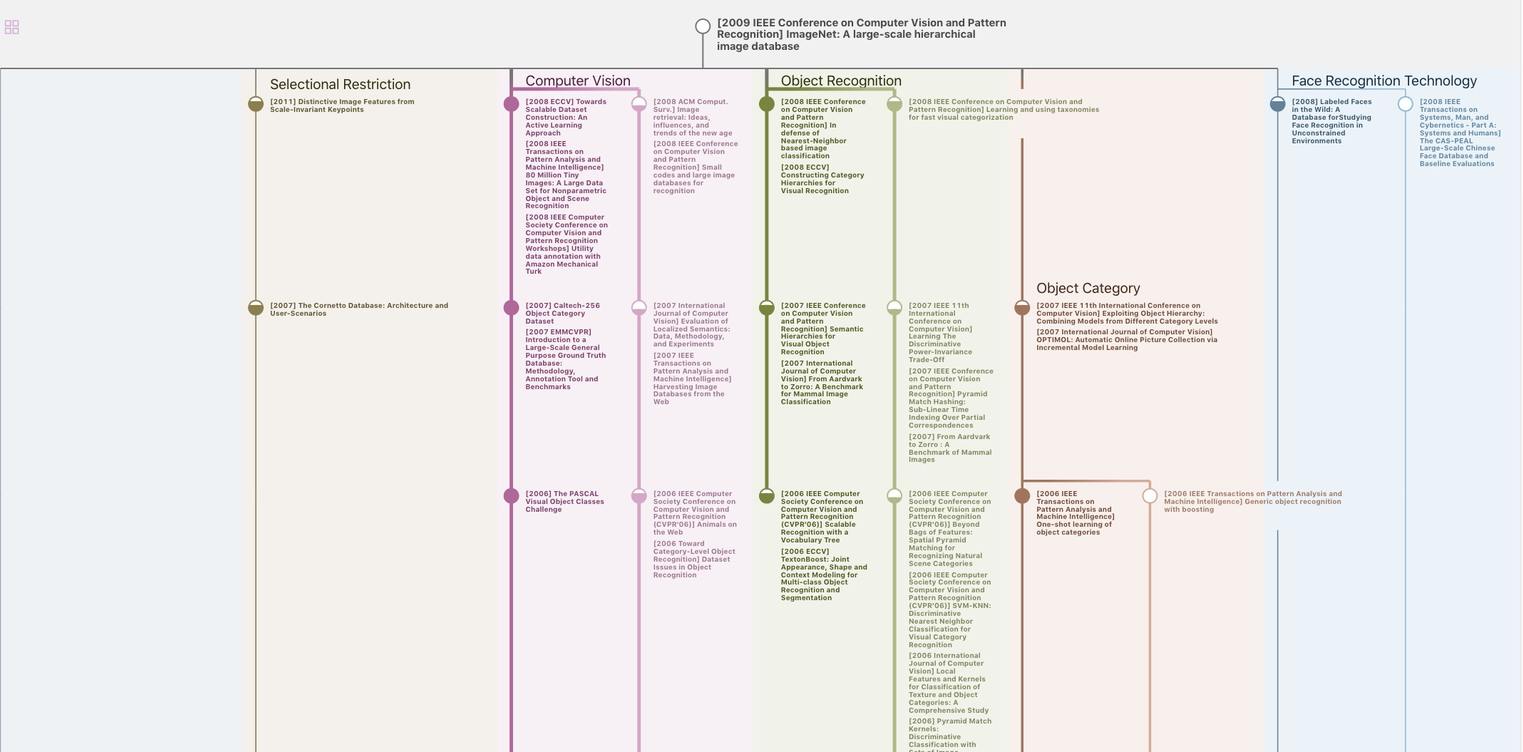
生成溯源树,研究论文发展脉络
Chat Paper
正在生成论文摘要