Neural Networks Techniques for Fault Detection and Offset Prediction on Wind Turbines Sensors
17th International Conference on Soft Computing Models in Industrial and Environmental Applications (SOCO 2022)(2022)
摘要
Digital Twins (DT) are one of the basis of Industry 4.0. DTs are used to simulate physical assets and improve the efficiency and decision making of industrial production. DT models are usually fitted with data collected from their physical counterparts through sensor readings. The data quality of the information retrieved by sensors is one main problem when training and retraining DT models. Poor data quality leads to low-accuracy DT predictions. In this study, a methodology is proposed for fault detection and offset error prediction problems related to retraining the DT of two wind turbine systems. Wind turbines are of utmost importance in Industry 4.0, as the use of renewable energy reduces production cost and benefits the environment. Having time series data sets with sensor readings of two real wind turbines, machine learning techniques based on Recurrent Neural Networks (RNNs) with Long Short Term Memory (LSTM) layers were implemented for multiple sensor fault forecasting. High-precision models were obtained and experiments were designed and performed to test the effectiveness of the proposed multi-fault detection system. The strengths and weaknesses of the approach are presented, which shows the relevance of this methodology for the DT retraining process.
更多查看译文
关键词
Digital twin, Wind turbine, Sensors, Fault detection, LSTM networks
AI 理解论文
溯源树
样例
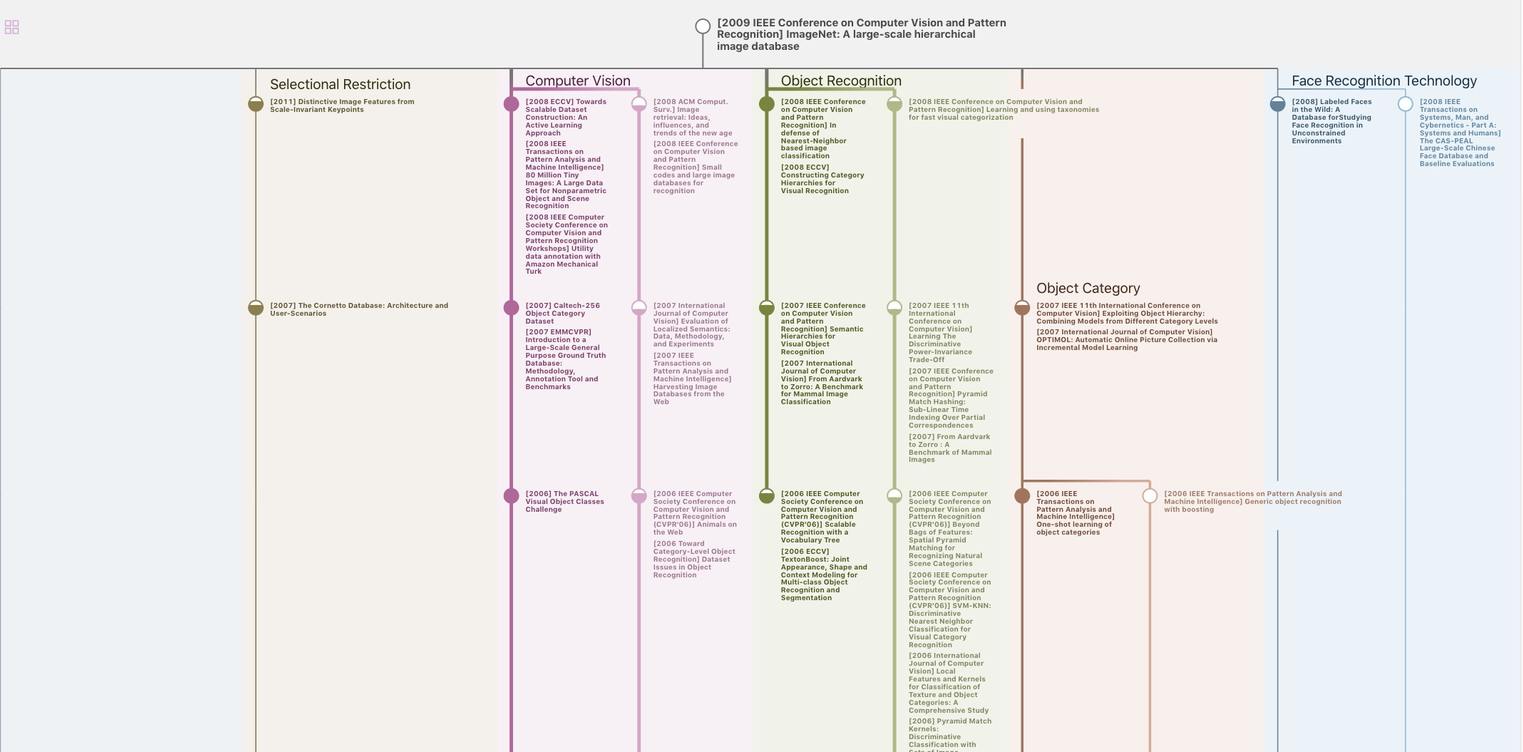
生成溯源树,研究论文发展脉络
Chat Paper
正在生成论文摘要