Efficient Gaussian Process Model on Class-Imbalanced Datasets for Generalized Zero-Shot Learning
2022 26th International Conference on Pattern Recognition (ICPR)(2022)
摘要
Zero-Shot Learning (ZSL) models aim to classify object classes that are not seen during the training process. However, the problem of class imbalance is rarely discussed, despite its presence in several ZSL datasets. In this paper, we propose a Neural Network model that learns a latent feature embedding and a Gaussian Process (GP) regression model that predicts latent feature prototypes of unseen classes. A calibrated classifier is then constructed for ZSL and Generalized ZSL tasks. Our Neural Network model is trained efficiently with a simple training strategy that mitigates the impact of class-imbalanced training data. The model has an average training time of 5 minutes and can achieve state-of-the-art (SOTA) performance on imbalanced ZSL benchmark datasets like AWA2, AWA1 and APY, while having relatively good performance on the SUN and CUB datasets.
更多查看译文
关键词
efficient gaussian process model,learning,class-imbalanced,zero-shot
AI 理解论文
溯源树
样例
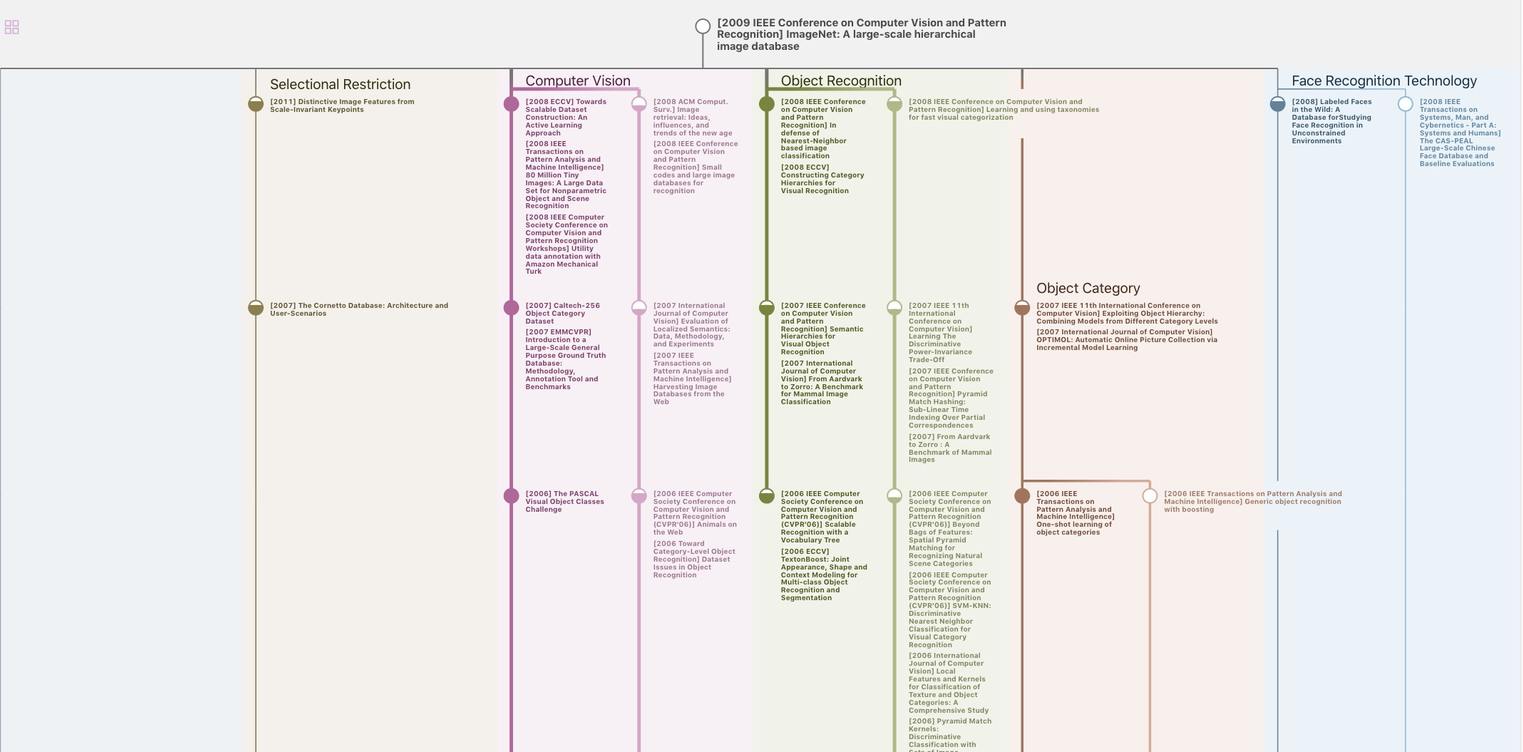
生成溯源树,研究论文发展脉络
Chat Paper
正在生成论文摘要