Parameter estimation of the homodyned K distribution based on neural networks and trainable fractional-order moments
CoRR(2022)
Abstract
Homodyned K (HK) distribution has been widely used to describe the scattering phenomena arising in various research fields, such as ultrasound imaging or optics. In this work, we propose a machine learning based approach to the estimation of the HK distribution parameters. We develop neural networks that can estimate the HK distribution parameters based on the signal-to-noise ratio, skewness and kurtosis calculated using fractional-order moments. Compared to the previous approaches, we consider the orders of the moments as trainable variables that can be optimized along with the network weights using the back-propagation algorithm. Networks are trained based on samples generated from the HK distribution. Obtained results demonstrate that the proposed method can be used to accurately estimate the HK distribution parameters.
MoreTranslated text
Key words
parameter estimation,neural
AI Read Science
Must-Reading Tree
Example
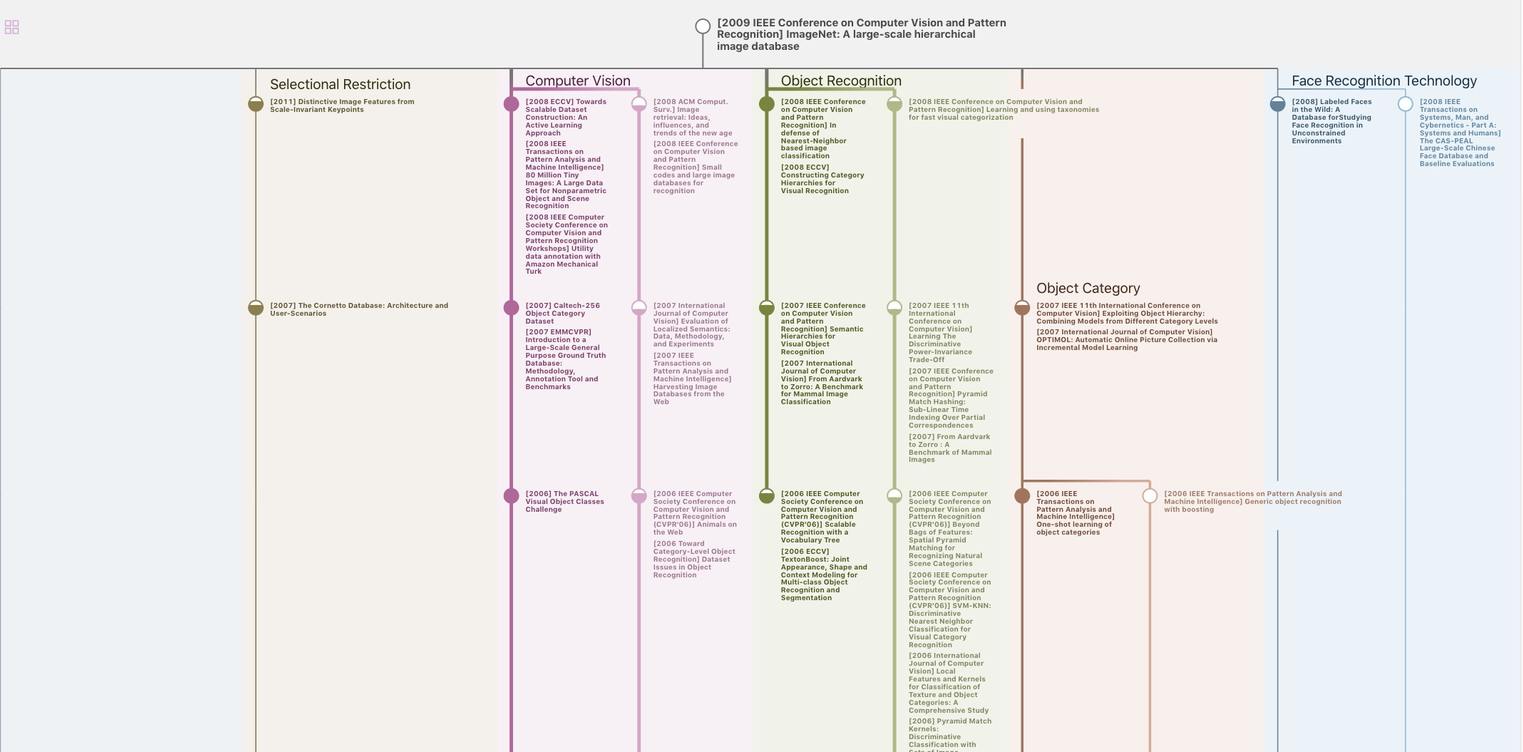
Generate MRT to find the research sequence of this paper
Chat Paper
Summary is being generated by the instructions you defined