A Multi-Agent Approach for Adaptive Finger Cooperation in Learning-based In-Hand Manipulation
2023 IEEE INTERNATIONAL CONFERENCE ON ROBOTICS AND AUTOMATION, ICRA(2023)
Abstract
In-hand manipulation is challenging for a multi-finger robotic hand due to its high degrees of freedom and complex interaction with the object. To enable in-hand manipulation, existing deep reinforcement learning-based approaches mainly focus on training a single robot-structure-specific policy through the centralized learning mechanism, lacking adaptability to changes like robot malfunction. To solve this limitation, this work treats each finger as an individual agent and trains multiple agents to control their assigned fingers to complete the in-hand manipulation task cooperatively. We propose the Multi-Agent Global-Observation Critic and Local-Observation Actor (MAGCLA) method, where the critic can observe all agents' actions globally, and the actor only locally observes its neighbors' actions. Besides, conventional individual experience replay may cause unstable cooperation due to the asynchronous performance increment of each agent, which is critical for in-hand manipulation tasks. To solve this issue, we propose the Synchronized Hindsight Experience Replay (SHER) method to synchronize and efficiently reuse the replayed experience across all agents. The methods are evaluated in two in-hand manipulation tasks on the Shadow dexterous hand. The results show that SHER helps MAGCLA achieve comparable learning efficiency to a single policy, and the MAGCLA approach is more generalizable in different tasks. The trained policies have higher adaptability in the robot malfunction test compared to the baseline multi-agent and single-agent approaches.
MoreTranslated text
Key words
adaptive finger cooperation,multi-agent,learning-based,in-hand
AI Read Science
Must-Reading Tree
Example
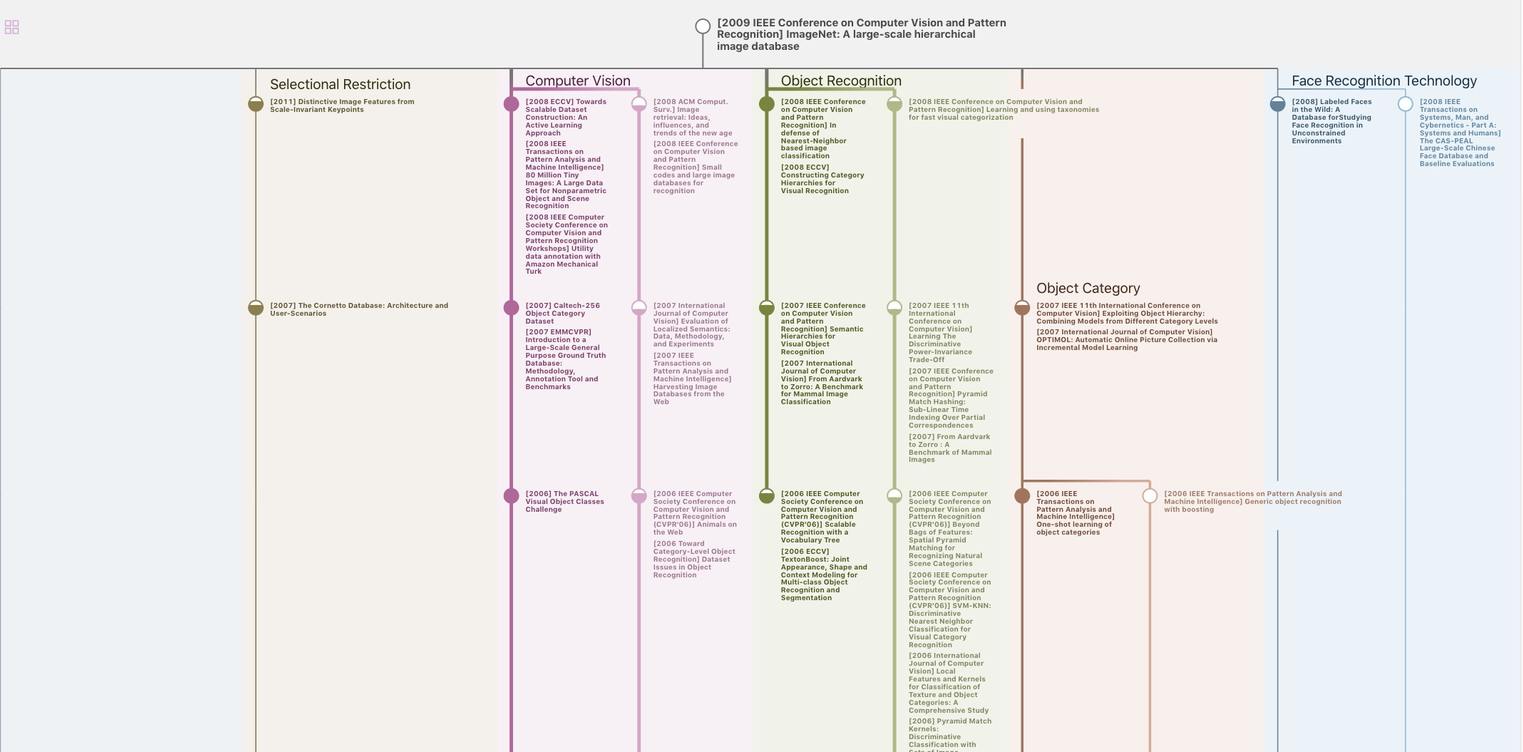
Generate MRT to find the research sequence of this paper
Chat Paper
Summary is being generated by the instructions you defined