Electrocardiograph signal recognition using wavelet transform based on optimized neural network
International Journal of Power Electronics and Drive Systems(2022)
Abstract
Due to the growing number of cardiac patients, an automatic detection that detects various heart abnormalities has been developed to relieve and share physicians’ workload. Many of the depolarization of ventricles complex waves (QRS) detection algorithms with multiple properties have recently been presented; nevertheless, real-time implementations in low-cost systems remain a challenge due to limited hardware resources. The proposed algorithm finds a solution for the delay in processing by minimizing the input vector’s dimension and, as a result, the classifier’s complexity. In this paper, the wavelet transform is employed for feature extraction. The optimized neural network is used for classification with 8-classes for the electrocardiogram (ECG) signal this data is taken from two ECG signals (ST-T and MIT-BIH database). The wavelet transform coefficients are used for the artificial neural network’s training process and optimized by using the invasive weed optimization (IWO) algorithm. The suggested system has a sensitivity of over 70%, a specificity of over 94%, a positive predictive of over 65%, a negative predictive of more than 93%, and a classification accuracy of more than 80%. The performance of the classifier improves when the number of neurons in the hidden layer is increased.
MoreTranslated text
Key words
Arrhythmia Detection,Deep Learning for EEG,Heartbeat Classification,Epilepsy Detection,EEG Analysis
AI Read Science
Must-Reading Tree
Example
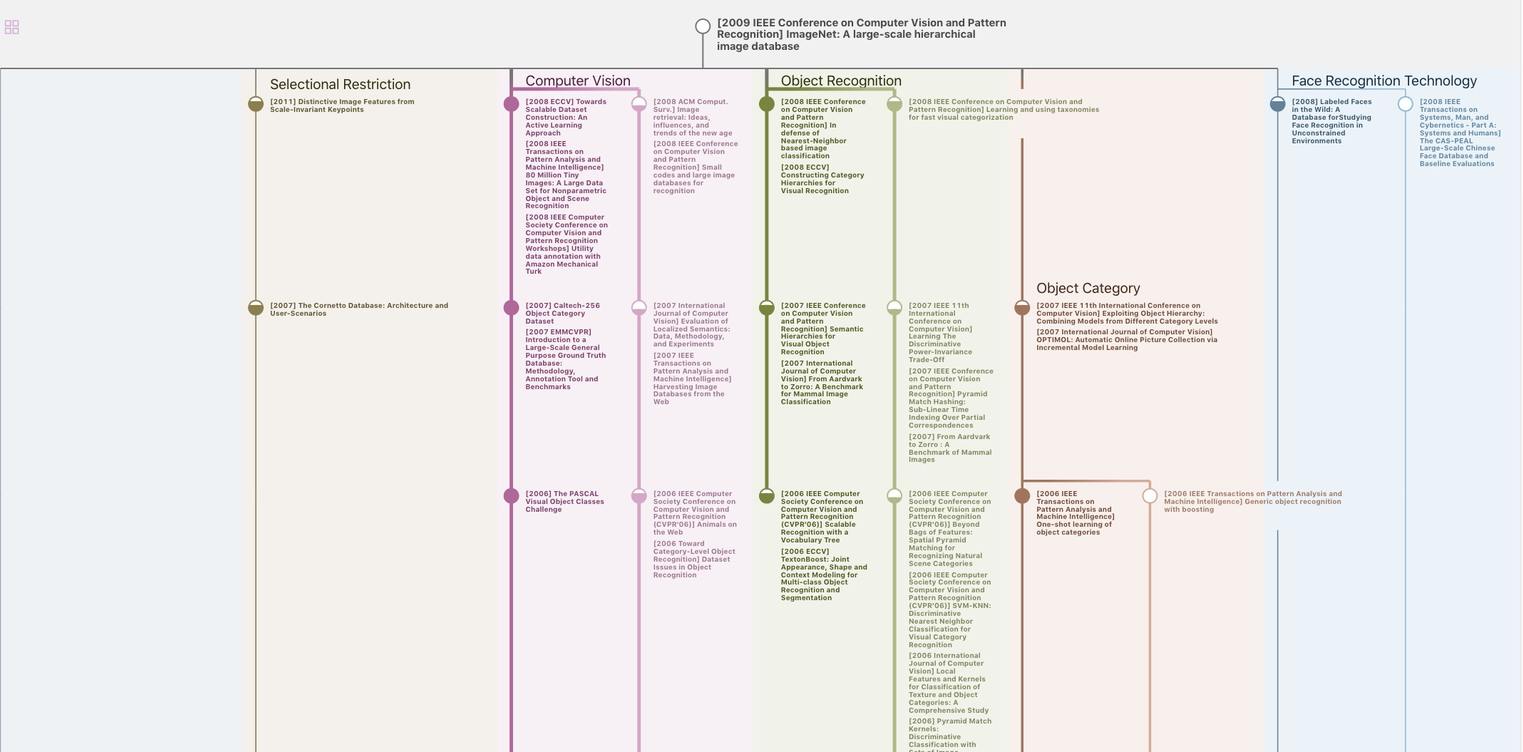
Generate MRT to find the research sequence of this paper
Chat Paper
Summary is being generated by the instructions you defined