Towards edge-assisted real-time 3D segmentation of large scale LIDAR point clouds
Mobile Systems, Applications, and Services(2022)
摘要
BSTRACTLight detection and ranging (LIDAR) has become a cost-effective and accessible sensor for a broad range of embedded devices including mobile phones and drones. Vision applications of these embedded devices require fast and accurate inferences to drive them, while at the same time power consumption should be kept low. Achieving both these requirements is hard due to the size of high quality LIDAR point cloud data streams - significantly larger than vision inputs such as images. The complexity of point cloud segmentation adds further difficulty for achieving high quality, realtime LIDAR data driven vision applications on battery powered embedded devices. We consider edge offloading as a potential approach to reconcile these conflicting requirements. Specifically, we present an experimental characterization study exploring the benefit of edge-assisted LIDAR point cloud segmentation, considering diverse set of embedded devices and state-of-the-art semantic segmentation models. Our results indicate that edge offloading is always beneficial from a device energy efficiency perspective and can also significantly reduce inference latency, especially with compressive edge offloading. These latency improvements however fall short of meeting real-time requirements. We outline a number of potential follow-on research directions to enable edge assisted accurate and real-time LIDAR point cloud segmentation.
更多查看译文
AI 理解论文
溯源树
样例
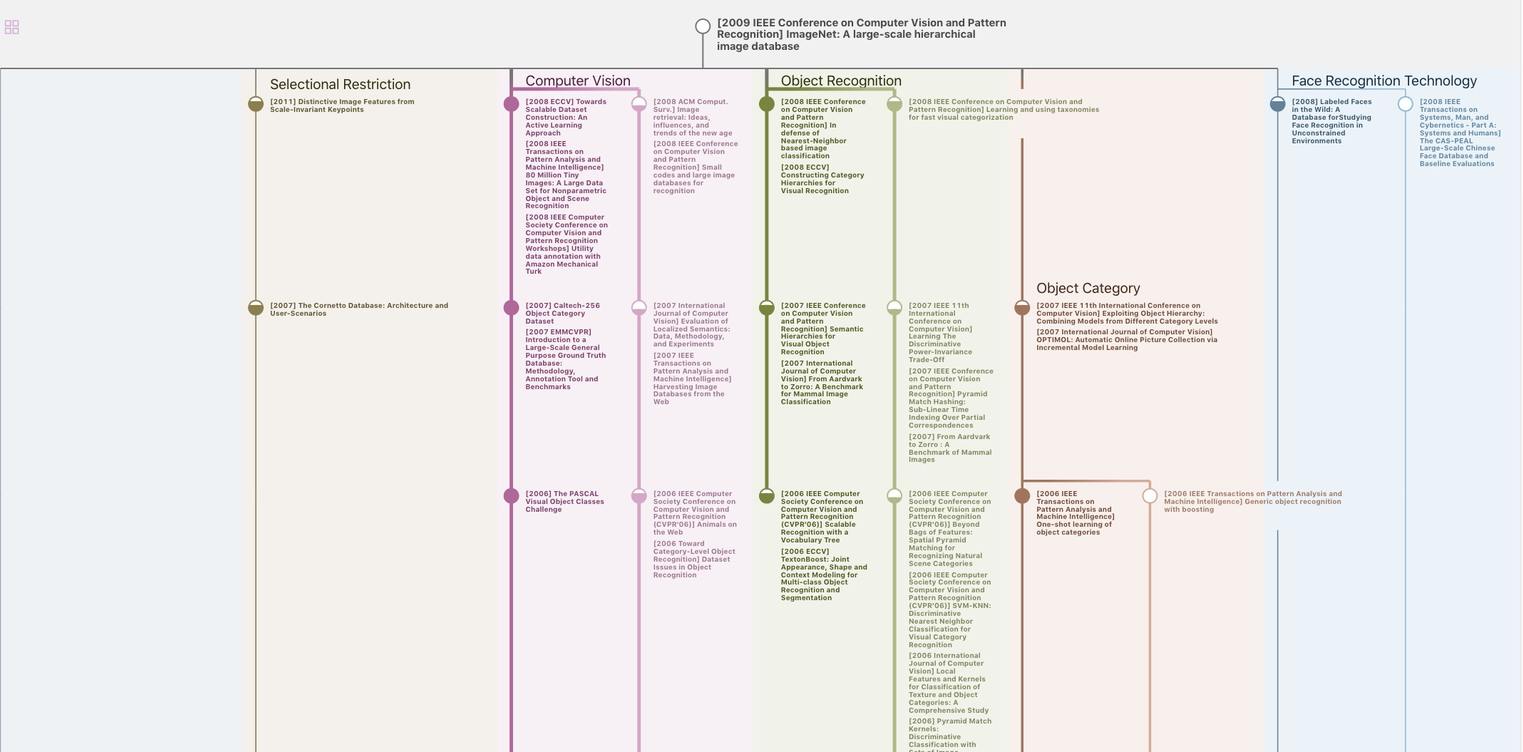
生成溯源树,研究论文发展脉络
Chat Paper
正在生成论文摘要