Artificial intelligence algorithms for the diagnosis of urothelial carcinoma based on urine cytology: A noninvasive and efficient diagnostic approach.
Journal of Clinical Oncology(2022)
Abstract
4590 Background: Urine cytology is a noninvasive and relatively inexpensive approach that plays an important role in both screening/initial diagnosis of urothelial carcinoma, and surveillance of tumor recurrence; However, interpretation of urine cytology is labor-intensive and time-consuming. Artificial intelligence (AI) has been widely used in the field of medically assisted diagnosis, but it is almost blank in the field of urothelial carcinoma diagnosis based on urine cytology. In this study, artificial intelligence (AI) algorithms have been developed to efficiently and automatically analyze urinary cytological specimens stained with Acridine orange fluorescence for screening and diagnosis of urothelial carcinoma with a good clinical application prospect. Methods: To develop the algorithms, images of urinary cytological specimens with Acridine orange Fluorescence staining were collected from patients who underwent examination, surgery, or both at the Second Hospital of Tianjin Medical University from August 2015 to August 2021. To standardize the urine cytological determination, the images were determined by 3 certified and experienced cytopathologists in this hospital. Images were collected to establish a dataset containing two major categories: Malignant (Defined as images containing malignant cells) and Benign (Defined as images containing malignant cells). From the acquired database, 2794 malignant and 2787 benign eligible images were selected for AI training/validation/testing. Inception V3, a specific type of neural network optimized for image classification called a deep convolutional neural network was trained based on the database. To prevent the model from over-fitting during training, data augmentation methods, such as rotation and flipping were adopted. The diagnosing performance of the binary classification model was analyzed using accuracy, F1 score, operating characteristic (ROC), and the area under the curve (AUC) generated from the sensitivity and specificity to evaluate. Results: A total of 21022 slides of urine cytological specimens with Acridine orange Fluorescence staining were collected. The AI algorithms achieved excellent performance, which showed the best prediction with an accuracy of 92.25%, a sensitivity of 98.50% along with a specificity of 86.00%, the F1score was 0.9271, and the area under the ROC curve was 0.96. Conclusions: Our novel artificial intelligence algorithms could accurately classify urine specimens as malignant or benign efficiently and automatically. The use of AI during cytopathology screening provides a new strategy and technical support for the diagnosis and screen of urothelial carcinoma. [Table: see text]
MoreTranslated text
Key words
Urothelial Carcinoma
AI Read Science
Must-Reading Tree
Example
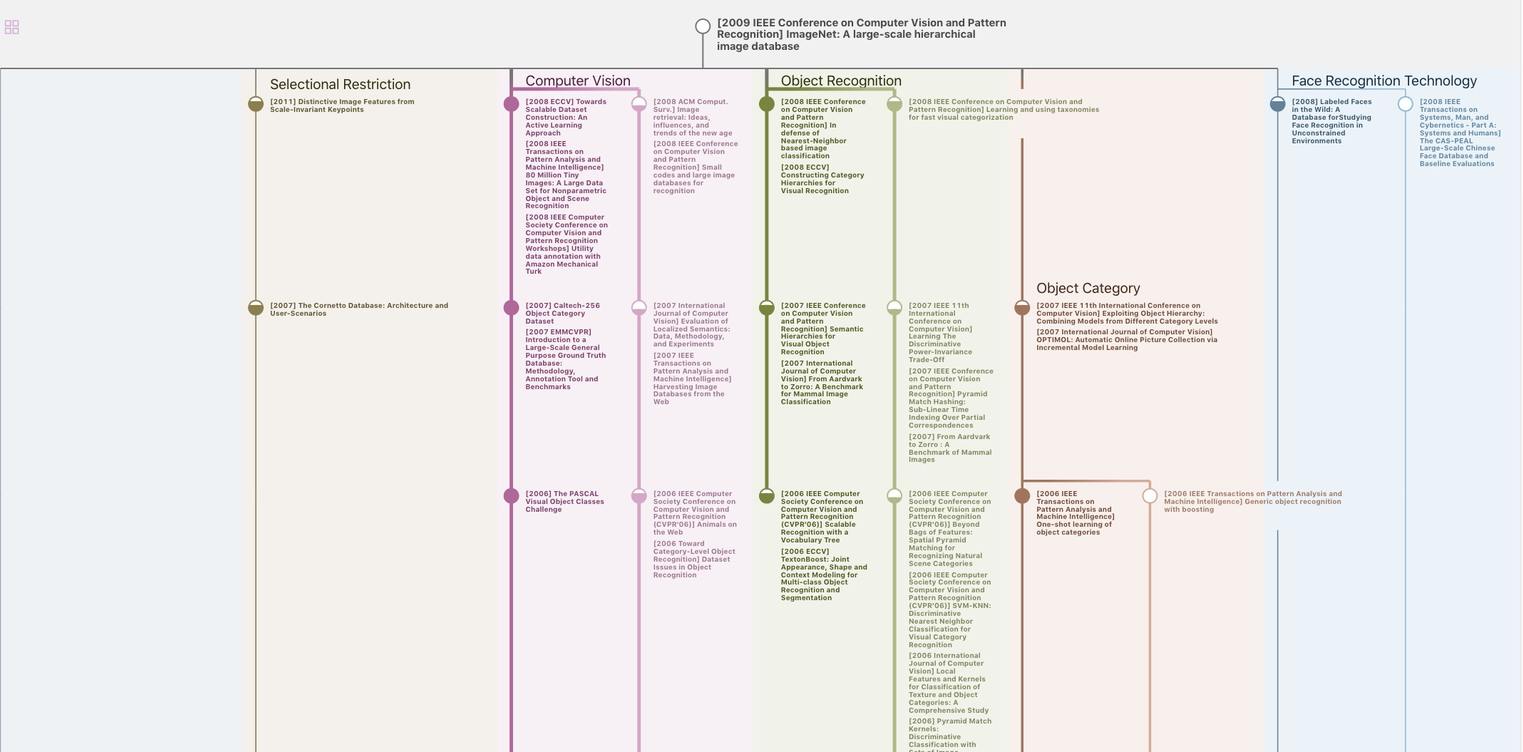
Generate MRT to find the research sequence of this paper
Chat Paper
Summary is being generated by the instructions you defined