Principal Component Analysis Based Kullback- Leibler Divergence for Die Cracks Detection
2020 International Conference on Sensing, Measurement & Data Analytics in the era of Artificial Intelligence (ICSMD)(2020)
Abstract
Die cracks are a vital issue that directly influences the quality of chip assemblies. In this paper, we focus on detecting die cracks using principal component analysis (PCA) and Kullback-Leibler (K-L) divergence. Our method involves data fusion, including three steps: 1) apply PCA to convert high-dimensional data to low-dimensional data; 2) obtain the frequency distribution histograms of the transformed data and fit them; 3) use K-L Divergence based state index to quantitatively evaluate die cracks. Our method works very well with real-life data. Die cracks are identified according to die cracks data showing skewed distribution, while normal data have Gaussian distribution. Moreover, the proposed state index could successfully detect die cracks.
MoreTranslated text
Key words
PCA,data fusion,Gaussian distribution,die cracks,K-L divergence,state index
AI Read Science
Must-Reading Tree
Example
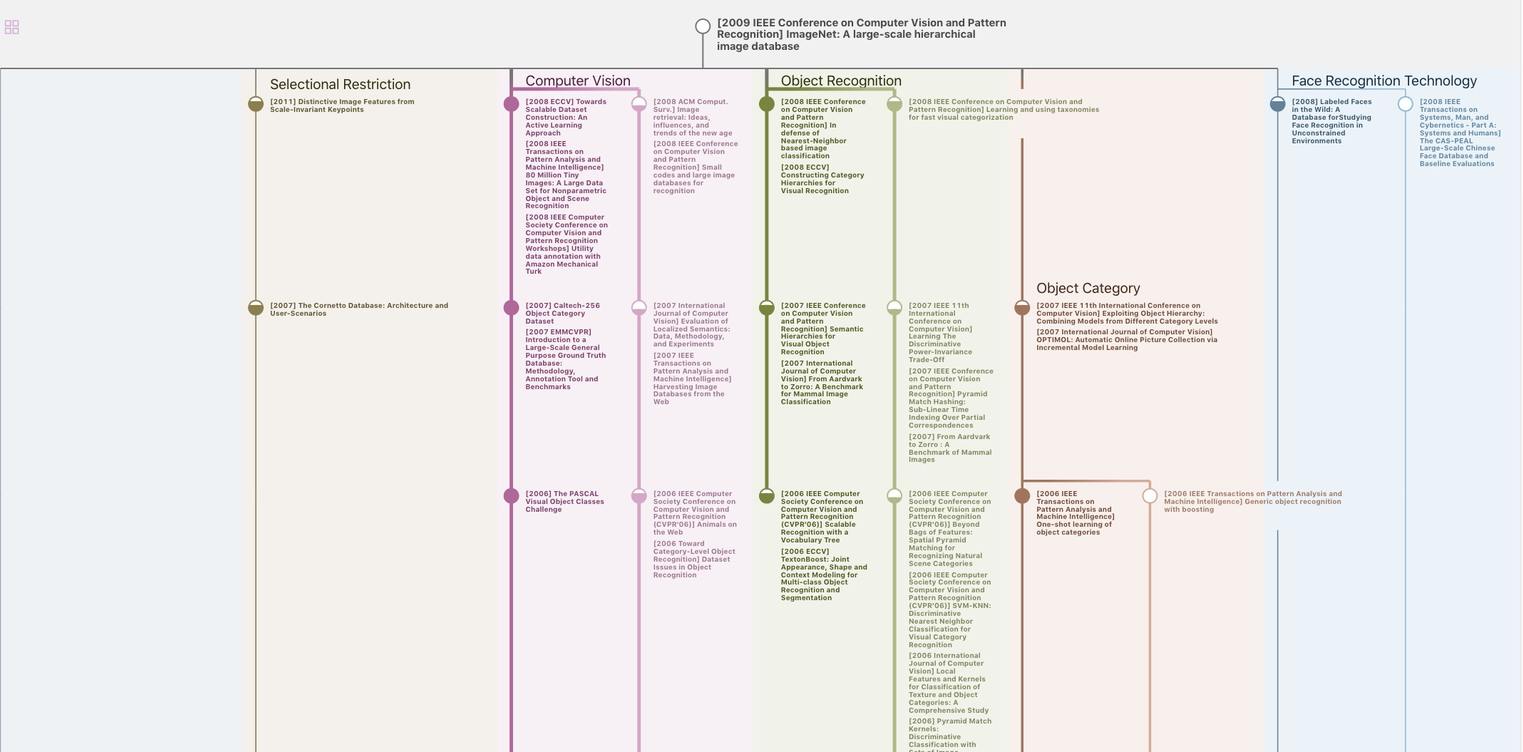
Generate MRT to find the research sequence of this paper
Chat Paper
Summary is being generated by the instructions you defined