A KLIEP-based Transfer Learning Model for Gear Fault Diagnosis under Varying Working Conditions
2020 International Conference on Sensing, Measurement & Data Analytics in the era of Artificial Intelligence (ICSMD)(2020)
摘要
Considering the fact that gear often works under varying working conditions, i.e., different domains, this paper presents a new approach that utilizes intrinsic time-scale decomposition (ITD) to extract features from vibration signals and transfer learning (TL) to achieve domain adaptation for gear fault diagnosis (GFD). The ITD first decomposes the vibration signal into several proper rotation components (PRCs). Then, the singular value vectors from the PRCs are extracted to provide differential indexes. TL aims to minimize the distribution distance among domains at most and solve the problem of distribution change, where a weight adjustment mechanism is involved in the Kullback-Leibler importance estimation procedure (KLIEP) for domain adaptation. Finally, the weighted domain vectors are applied to the GFD model. Experimental study performed on a drivetrain dynamics simulator (DDS) show that KLIEP performs well for domain adaption and the classification results also prove the superiority of proposed method in GFD when working condition changes.
更多查看译文
关键词
Gearbox fault diagnosis,Transfer learning,KLIEP,varying working conditions
AI 理解论文
溯源树
样例
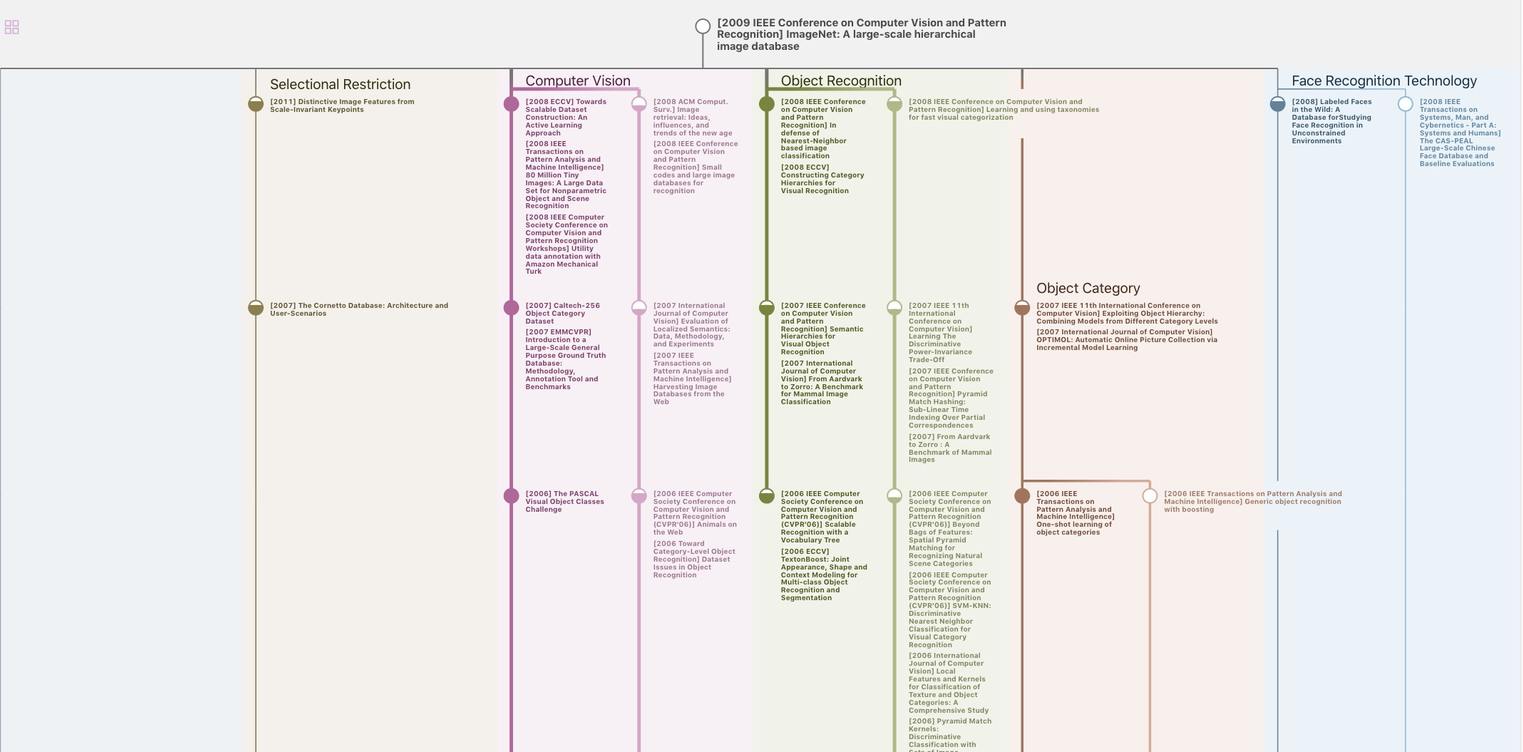
生成溯源树,研究论文发展脉络
Chat Paper
正在生成论文摘要