Deep Reinforcement Learning-based Optimization for End-to-end Network Slicing with Control- and User-Plane Separation
IEEE Transactions on Vehicular Technology(2022)
摘要
Control- and user-plane separation (CUPS) and network slicing are two key technologies to support increasing network traffic and diverse wireless services. However, the benefit of CUPS in decoupling the network coverage and data service functions has not been fully utilized to facilitate network slicing. In this paper, we present a novel CUPS-based end-to-end (CUPS-E2E) network slicing scheme. First, the base stations (BS) are classified into control BSs (CBS) that provide control plane (CP) coverage and traffic BSs (TBS) that deliver user plane (UP) traffic. Next, upon CBSs and TBSs being virtualized, we define four typical end-to-end (E2E) network slices: one for CP coverage, one for high-throughput services, one for computation-intensive services, and the other for delay-sensitive services. The utilities of the four E2E network slices are defined based on their coverage, throughput, computing capability and delay requirements, respectively. Then, a deep deterministic policy gradient (DDPG)-based algorithm is employed to maximize the long-term sum-utility of the four E2E network slices by jointly optimizing the allocation of communication and computing resources to the four network slices and the activation of virtual TBSs, while meeting the service requirements of all users. Simulation results show that our proposed CUPS-E2E network slicing scheme in conjunction with a DDPG-based sum-utility maximization algorithm can support the CP wide-coverage and massive access requirements as well as the UP high-throughput, computation-intensive and delay-sensitive services simultaneously, and outperforms the existing E2E network slicing schemes in terms of the sum-utility, coverage percentage, throughput and delay.
更多查看译文
关键词
Control- and user-plane separation,deep deterministic policy gradient,end-to-end network slicing,utility
AI 理解论文
溯源树
样例
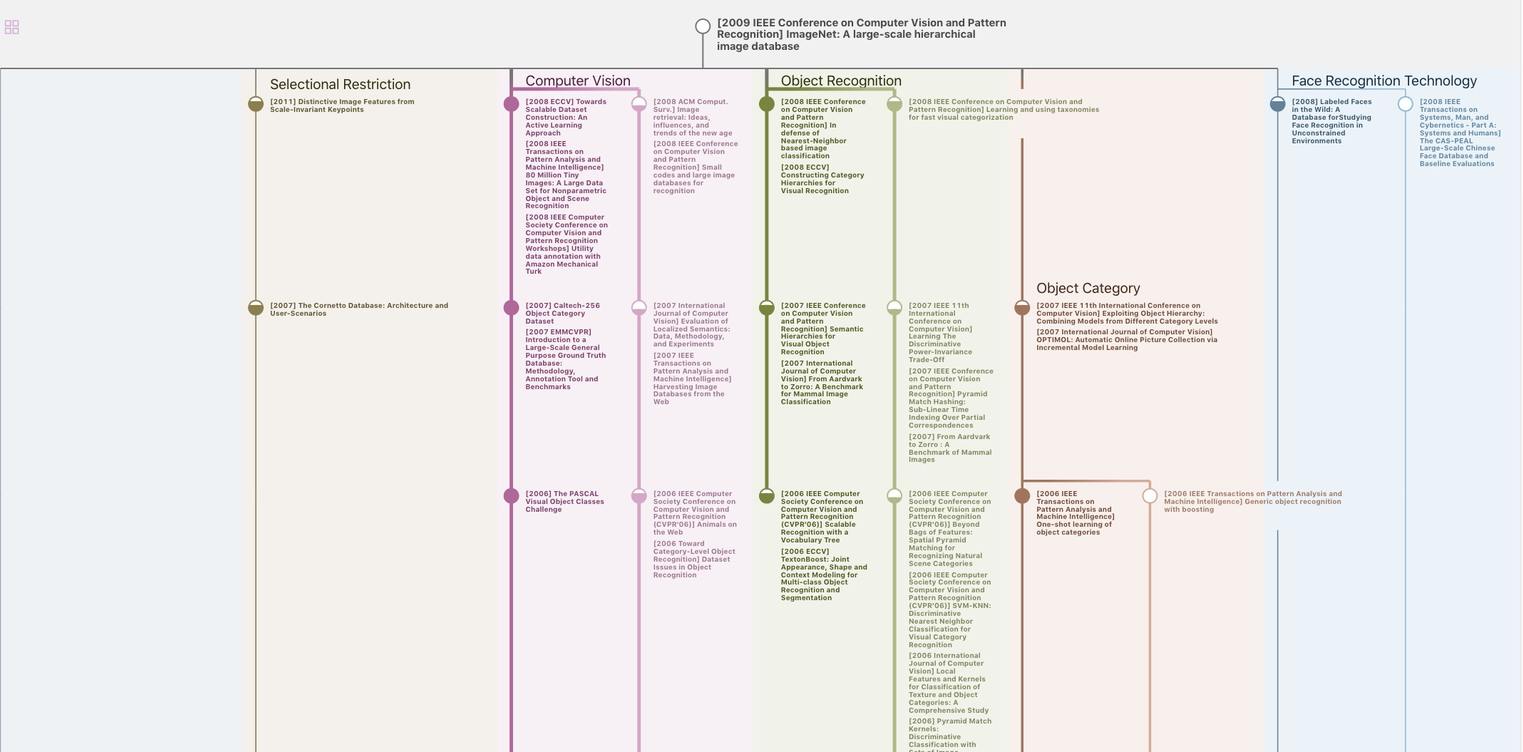
生成溯源树,研究论文发展脉络
Chat Paper
正在生成论文摘要