A New Initialization Technique: Truncated Towers
2022 30th Signal Processing and Communications Applications Conference (SIU)(2022)
摘要
Artificial Neural Networks (ANN) can perform various tasks by modifying their parameters, determined by initialization methods, using activation functions and learning algorithms. Alongside chosen training dataset, suitability and success of the chosen methods determine how well they can fulfill these tasks. With the ‘Truncated Tower Distribution’, which we developed to find a better initialization method, a partial and visible increase in success rates have been achieved in classification tasks on different data sets, using neuron number-independent and neuron-number-dependent initialization methods.
更多查看译文
关键词
artificial neural networks,initialization methods,truncated towers distribution,residual networks
AI 理解论文
溯源树
样例
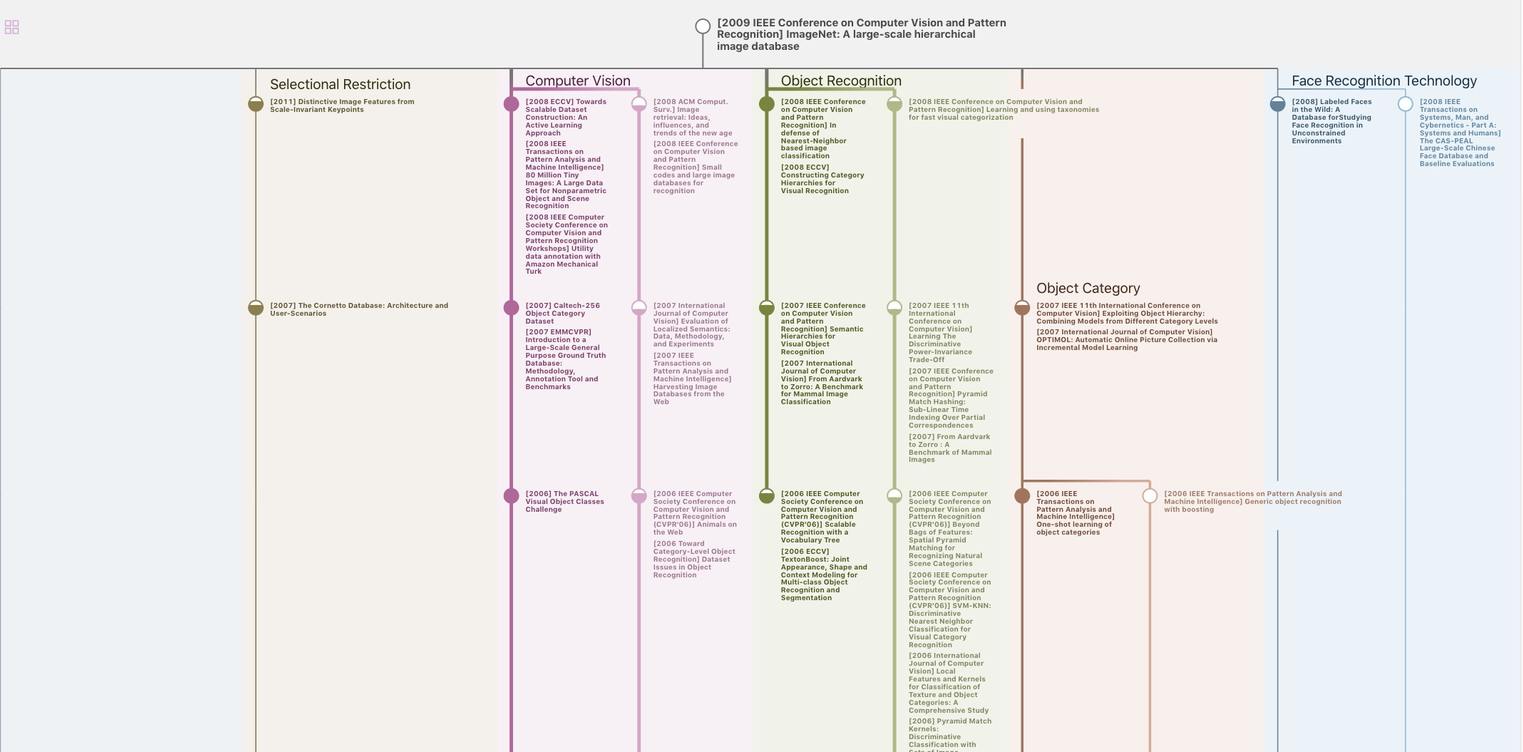
生成溯源树,研究论文发展脉络
Chat Paper
正在生成论文摘要