Memory transformers for full context and high-resolution 3D Medical Segmentation
arxiv(2022)
摘要
Transformer models achieve state-of-the-art results for image segmentation. However, achieving long-range attention, necessary to capture global context, with high-resolution 3D images is a fundamental challenge. This paper introduces the Full resolutIoN mEmory (FINE) transformer to overcome this issue. The core idea behind FINE is to learn memory tokens to indirectly model full range interactions while scaling well in both memory and computational costs. FINE introduces memory tokens at two levels: the first one allows full interaction between voxels within local image regions (patches), the second one allows full interactions between all regions of the 3D volume. Combined, they allow full attention over high resolution images, e.g. 512 x 512 x 256 voxels and above. Experiments on the BCV image segmentation dataset shows better performances than state-of-the-art CNN and transformer baselines, highlighting the superiority of our full attention mechanism compared to recent transformer baselines, e.g. CoTr, and nnFormer.
更多查看译文
关键词
Transformers,3D segmentation,Full context,High-resolution
AI 理解论文
溯源树
样例
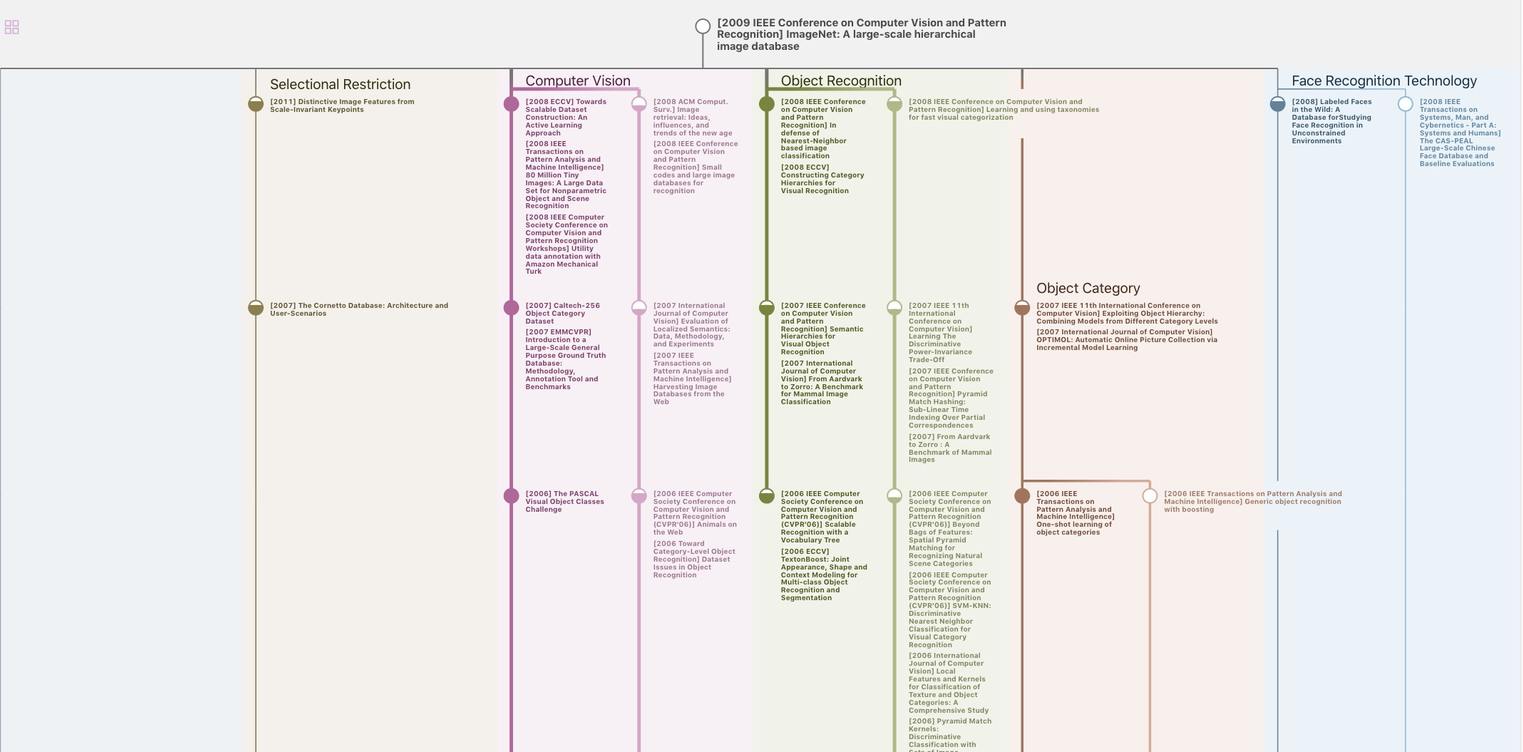
生成溯源树,研究论文发展脉络
Chat Paper
正在生成论文摘要