Improving Robustness of Retrieval Augmented Translation via Shuffling of Suggestions
CoRR(2022)
Abstract
Several recent studies have reported dramatic performance improvements in neural machine translation (NMT) by augmenting translation at inference time with fuzzy-matches retrieved from a translation memory (TM). However, these studies all operate under the assumption that the TMs available at test time are highly relevant to the testset. We demonstrate that for existing retrieval augmented translation methods, using a TM with a domain mismatch to the test set can result in substantially worse performance compared to not using a TM at all. We propose a simple method to expose fuzzy-match NMT systems during training and show that it results in a system that is much more tolerant (regaining up to 5.8 BLEU) to inference with TMs with domain mismatch. Also, the model is still competitive to the baseline when fed with suggestions from relevant TMs.
MoreTranslated text
AI Read Science
Must-Reading Tree
Example
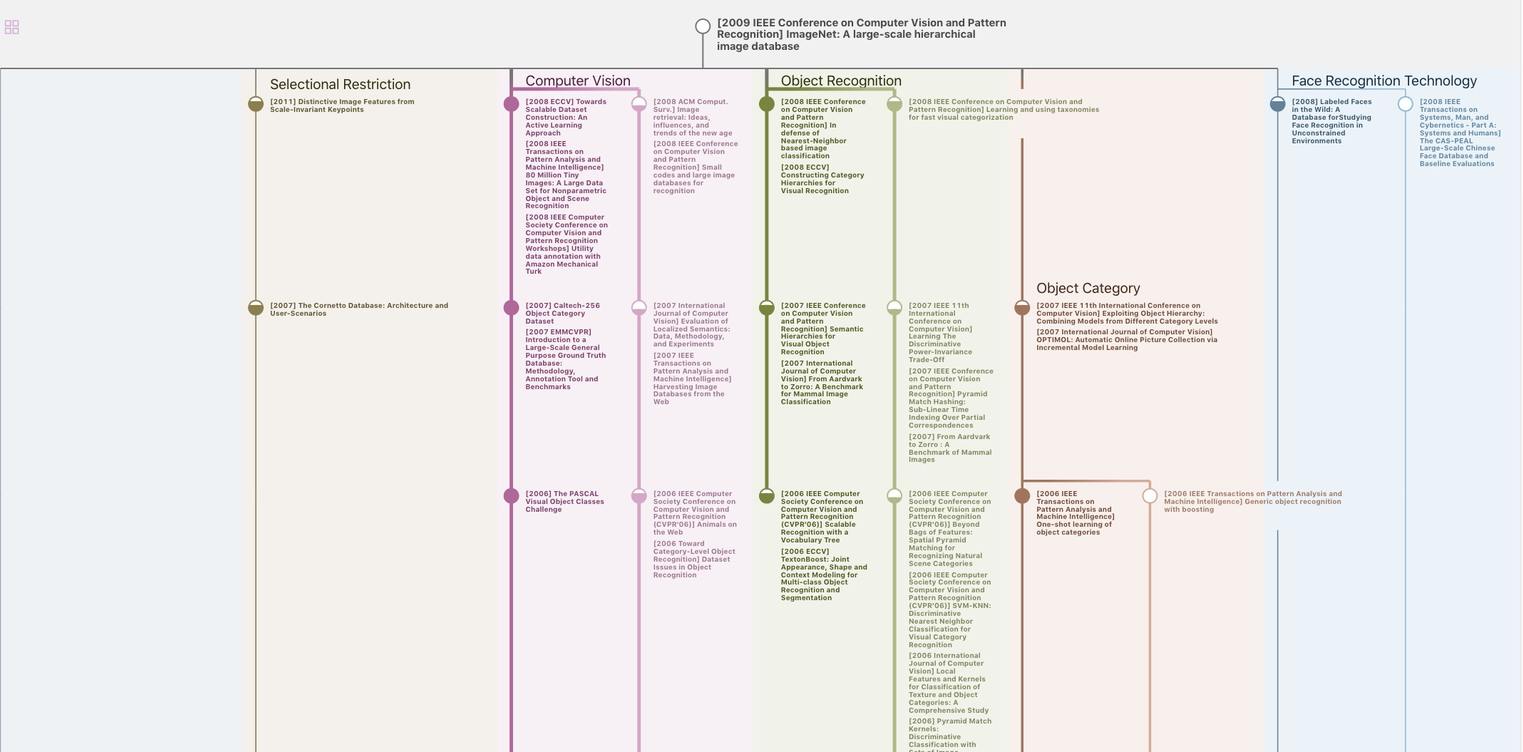
Generate MRT to find the research sequence of this paper
Chat Paper
Summary is being generated by the instructions you defined