Improving Dense Contrastive Learning with Dense Negative Pairs
arXiv (Cornell University)(2023)
Abstract
Many contrastive representation learning methods learn a single global representation of an entire image. However, dense contrastive representation learning methods such as DenseCL (Wang et al., 2021) can learn better representations for tasks requiring stronger spatial localization of features, such as multi-label classification, detection, and segmentation. In this work, we study how to improve the quality of the representations learned by DenseCL by modifying the training scheme and objective function, and propose DenseCL++. We also conduct several ablation studies to better understand the effects of: (i) various techniques to form dense negative pairs among augmentations of different images, (ii) cross-view dense negative and positive pairs, and (iii) an auxiliary reconstruction task. Our results show 3.5% and 4% mAP improvement over SimCLR (Chen et al., 2020a) andDenseCL in COCO multi-label classification. In COCO and VOC segmentation tasks, we achieve 1.8% and 0.7% mIoU improvements over SimCLR, respectively.
MoreTranslated text
Key words
dense contrastive learning,improving
AI Read Science
Must-Reading Tree
Example
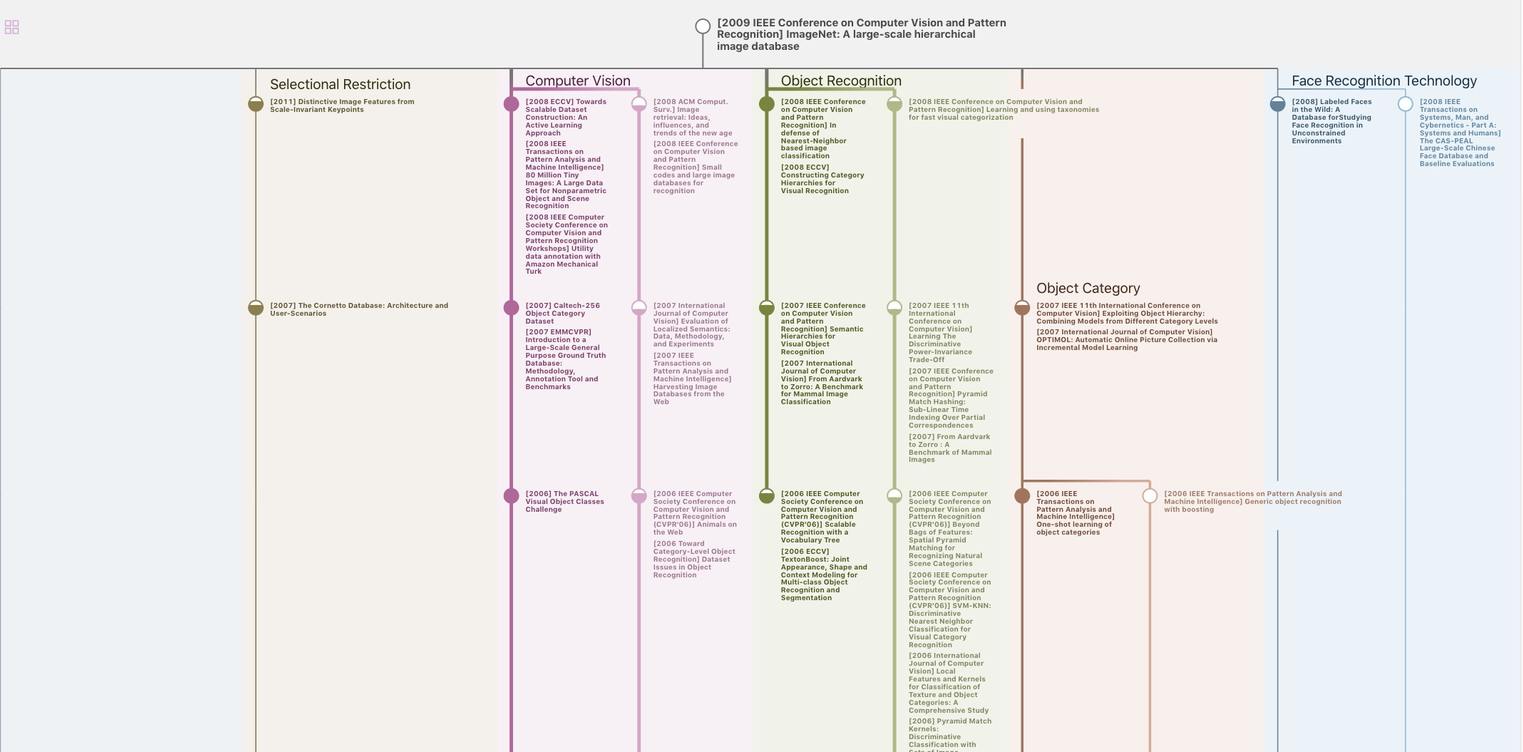
Generate MRT to find the research sequence of this paper
Chat Paper
Summary is being generated by the instructions you defined