Relational Attention: Generalizing Transformers for Graph-Structured Tasks
ICLR 2023(2022)
摘要
Transformers flexibly operate over sets of real-valued vectors representing task-specific entities and their attributes, where each vector might encode one word-piece token and its position in a sequence, or some piece of information that carries no position at all. But as set processors, transformers are at a disadvantage in reasoning over more general graph-structured data where nodes represent entities and edges represent relations between entities. To address this shortcoming, we generalize transformer attention to consider and update edge vectors in each transformer layer. We evaluate this relational transformer on a diverse array of graph-structured tasks, including the large and challenging CLRS Algorithmic Reasoning Benchmark. There, it dramatically outperforms state-of-the-art graph neural networks expressly designed to reason over graph-structured data. Our analysis demonstrates that these gains are attributable to relational attention's inherent ability to leverage the greater expressivity of graphs over sets.
更多查看译文
关键词
Graph Neural Networks,Transformers,Graph Representation Learning,Neural Algorithmic Reasoning
AI 理解论文
溯源树
样例
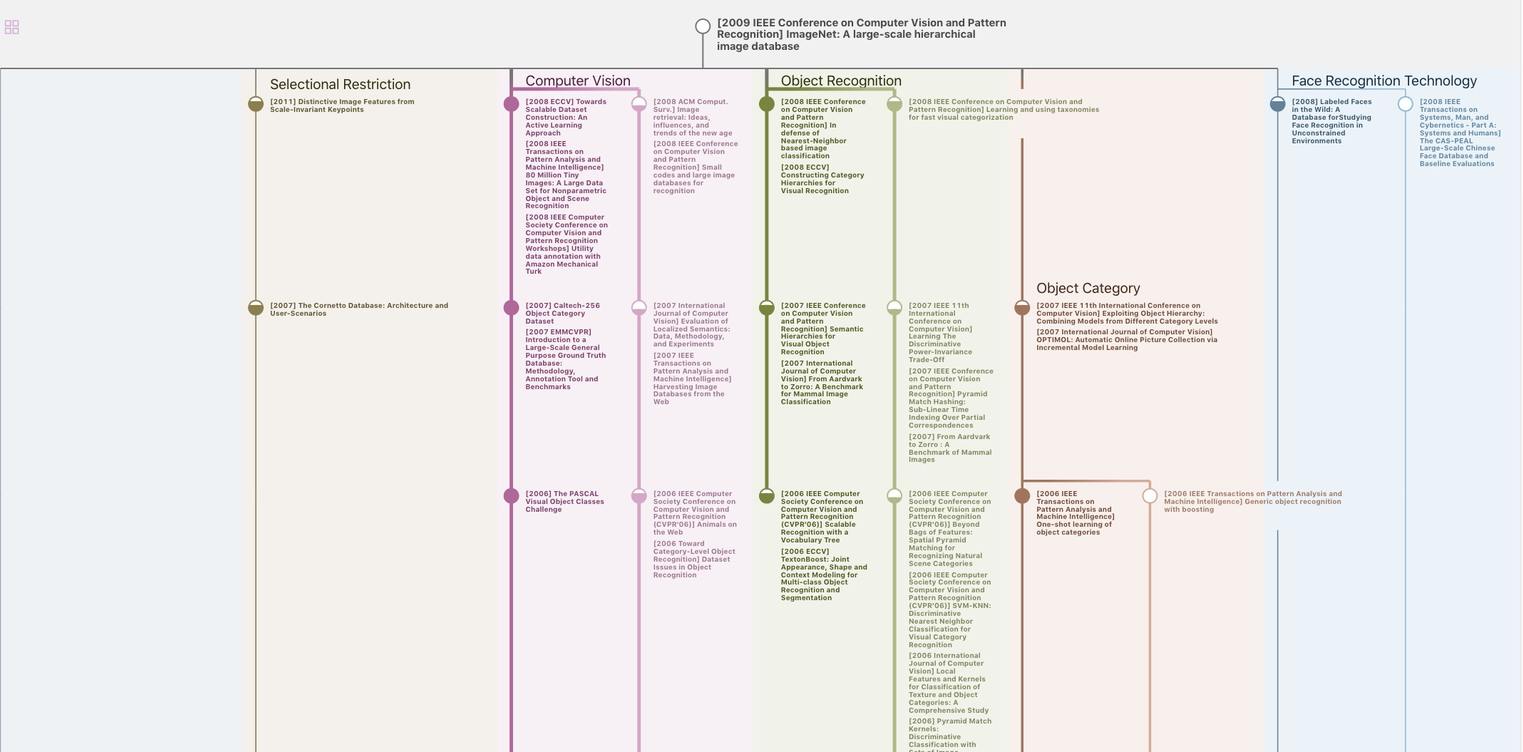
生成溯源树,研究论文发展脉络
Chat Paper
正在生成论文摘要