Improving Retrieval Augmented Neural Machine Translation by Controlling Source and Fuzzy-Match Interactions
arxiv(2022)
摘要
We explore zero-shot adaptation, where a general-domain model has access to customer or domain specific parallel data at inference time, but not during training. We build on the idea of Retrieval Augmented Translation (RAT) where top-k in-domain fuzzy matches are found for the source sentence, and target-language translations of those fuzzy-matched sentences are provided to the translation model at inference time. We propose a novel architecture to control interactions between a source sentence and the top-k fuzzy target-language matches, and compare it to architectures from prior work. We conduct experiments in two language pairs (En-De and En-Fr) by training models on WMT data and testing them with five and seven multi-domain datasets, respectively. Our approach consistently outperforms the alternative architectures, improving BLEU across language pair, domain, and number k of fuzzy matches.
更多查看译文
关键词
translation,fuzzy-match
AI 理解论文
溯源树
样例
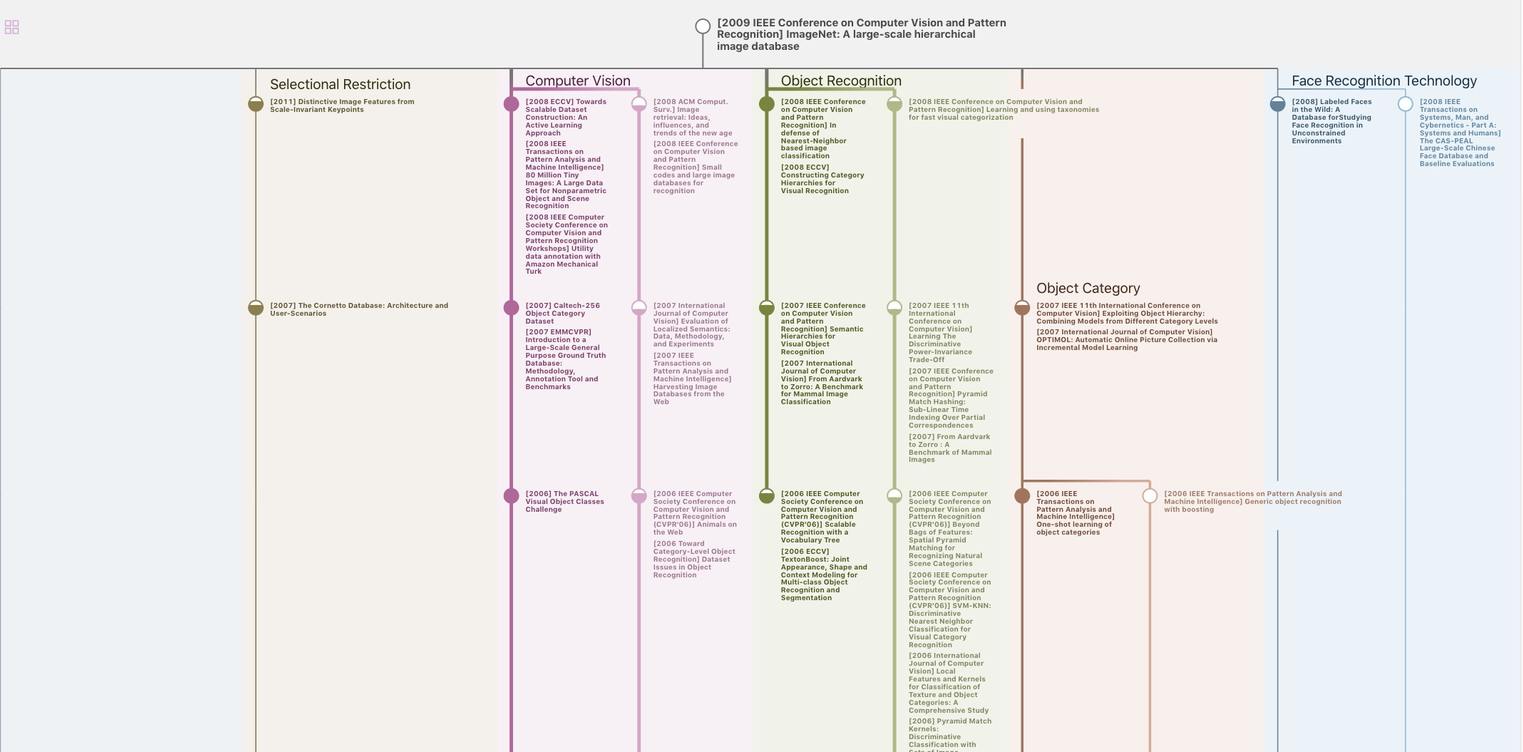
生成溯源树,研究论文发展脉络
Chat Paper
正在生成论文摘要