SFVNet: Stable and Fast Network for Real-Time Video Semantic Segmentation
2022 41st Chinese Control Conference (CCC)(2022)
摘要
Real-time video semantic segmentation in dynamic scenes is a dense prediction task. This task is computationally expensive but has high requirements for speed and stability, especially on mobile devices. In this paper, a stable and fast real-time video semantic segmentation network (SFVNet) is presented to deal with the above problems. Each module of SFVNet can be designed or selected freely. SFVNet propagates temporal information and extracts spatial information through several lightweight modules instead of large general networks. The temporal features and spatial features are aggregated under the guidance of inter-frame discontiguous features. An end-to-end training is implemented with a joint loss function, including temporal consistency constraint and sparsely annotated video frame supervision. A new metric is proposed for more appropriate evaluation of video semantic segmentation. It takes both temporal consistency and semantic accuracy into account. Experiments on Cityscapes dataset and various devices demonstrate that SFVNet achieves faster speed and higher consistency with controllable accuracy degradation than the baseline methods. SFVNet shows state-of-the-art efficiency in real-time video semantic segmentation.
更多查看译文
AI 理解论文
溯源树
样例
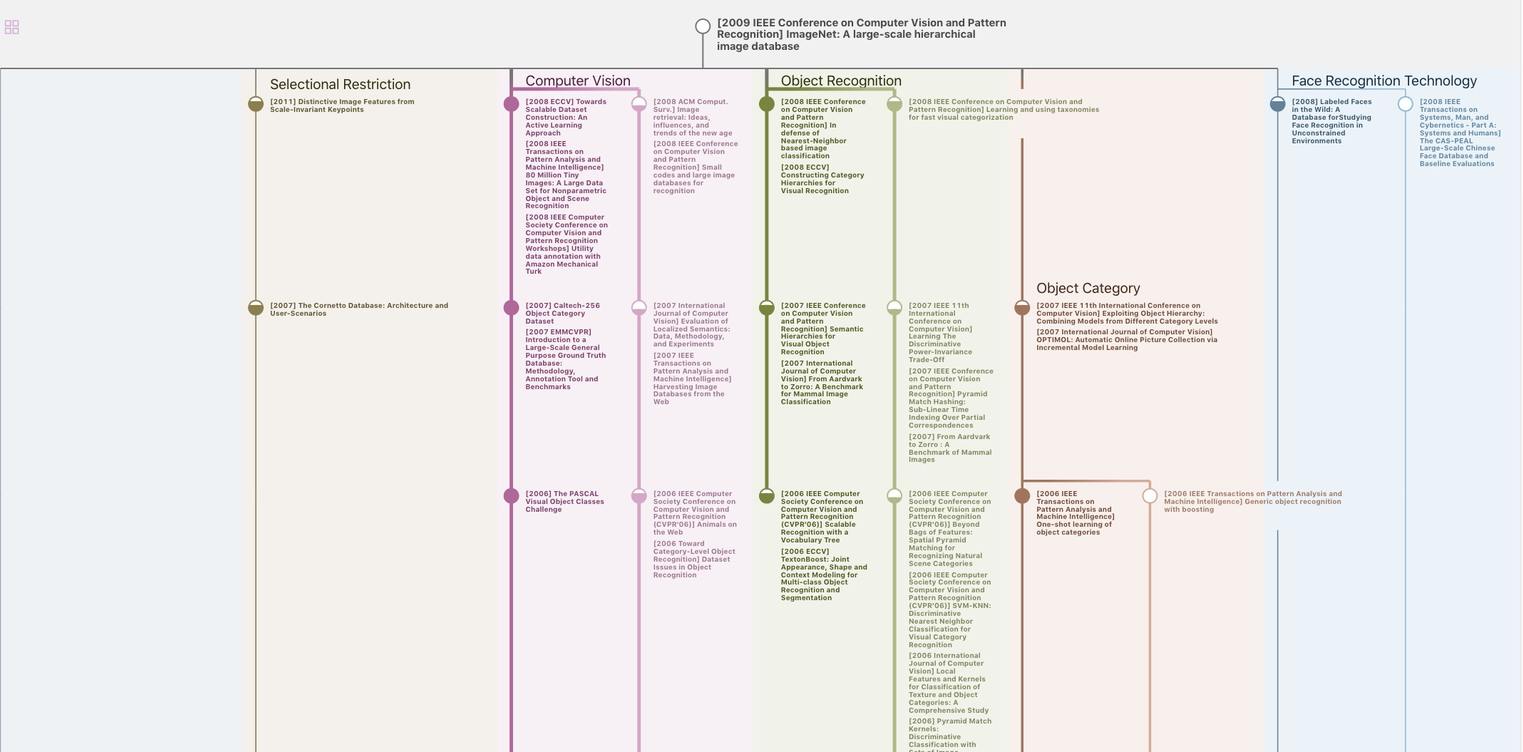
生成溯源树,研究论文发展脉络
Chat Paper
正在生成论文摘要