Soft Sensor for Anaerobic Digestion of Kitchen Waste with Semi-Supervised Extreme Learning Machine-based Graph Convolutional Network
2022 41st Chinese Control Conference (CCC)(2022)
摘要
Anaerobic digestion (AD) has become the mainstream technology of kitchen waste. Volatile fatty acid (VFA) is an important parameter for monitoring AD, which currently cannot be monitored in real time and can only be obtained by sampling assays, so soft sensor techniques were introduced to estimate the VFA concentration. In this paper, for the problems that soft sensors exist without considering the spatial relationship between variables, we propose a graph convolutional network (GCN) to construct the graph structure of the spatial relationship between different variables, which extracts spatial feature information of the samples data. In order to prevent the lack of training data from over-fitting problem, we introduce an approach based on extreme learning machine auto-encoder (ELM-AE) to train GCN for feature extraction. Additionally, the idea of manifold regularization is introduced into the model to mine the data of unlabeled samples. Then, the manifold regularization extreme learning machine (MRELM) algorithm is used for the regression prediction. Finally, a graph convolutional network soft sensor based on semi-supervised extreme learning machine (S
2
-EGCN) is built to predict the concentration of VFA in real time. The simulation on the industrial data of a kitchen waste digestion plant shows that our model achieves good prediction results compared to existing methods.
更多查看译文
关键词
Anaerobic digestion, soft sensor, graph convolutional network, extreme learning machine
AI 理解论文
溯源树
样例
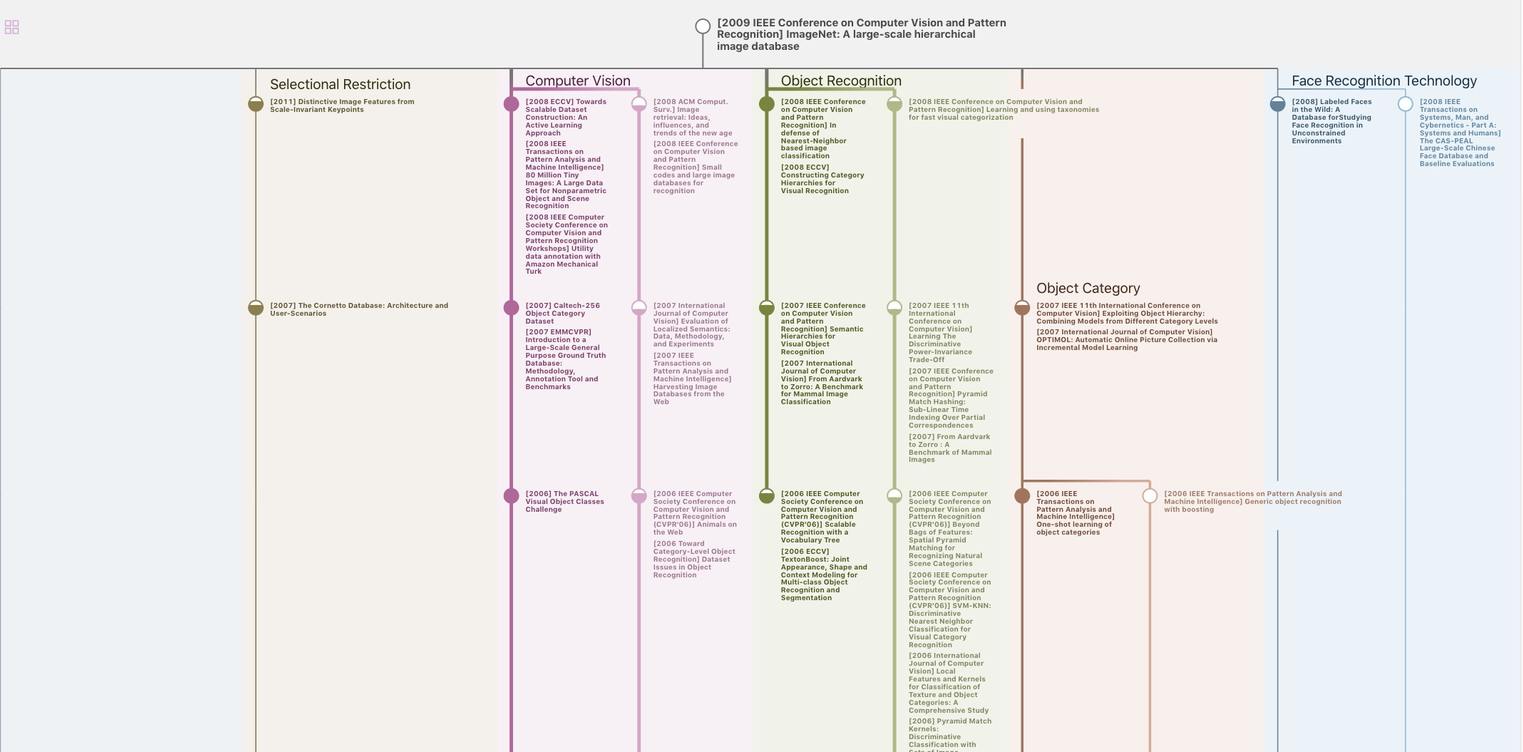
生成溯源树,研究论文发展脉络
Chat Paper
正在生成论文摘要