Siamese Network with Multi-scale Feature Fusion and Dual Attention Mechanism for Template Matching
2022 41st Chinese Control Conference (CCC)(2022)
Abstract
Factors like object's appearance changes, occlusions, and background changes have a negative impact on template matching tasks. To suppress this effect and improve template matching accuracy, we propose a Siamese network with multiscale feature fusion and dual attention module in this paper. A multi-scale feature fusion module is introduced to extract shallow structural and deep semantic features, and learnable weight coefficients are used to adaptively fuse multi-scale features to improve representation capabilities of the object. In order to improve feature recognition and eliminate redundancy caused by feature fusion, a dual attention mechanism module is introduced, which applies weights to features from separate channels and spatial positions. Finally, the features are normalized, and the cross-correlation is calculated to produce a similarity score map and locate the best matching region. When compared to the two state-of-the-art CNN-based template matching algorithms, QATM and Deep-DIM, the experimental results show that the algorithm in this paper improves template matching accuracy by 4.72 percent and 2.30 percent, respectively, and has the least time-consuming.
MoreTranslated text
Key words
feature fusion,dual attention mechanism,matching,multi-scale
AI Read Science
Must-Reading Tree
Example
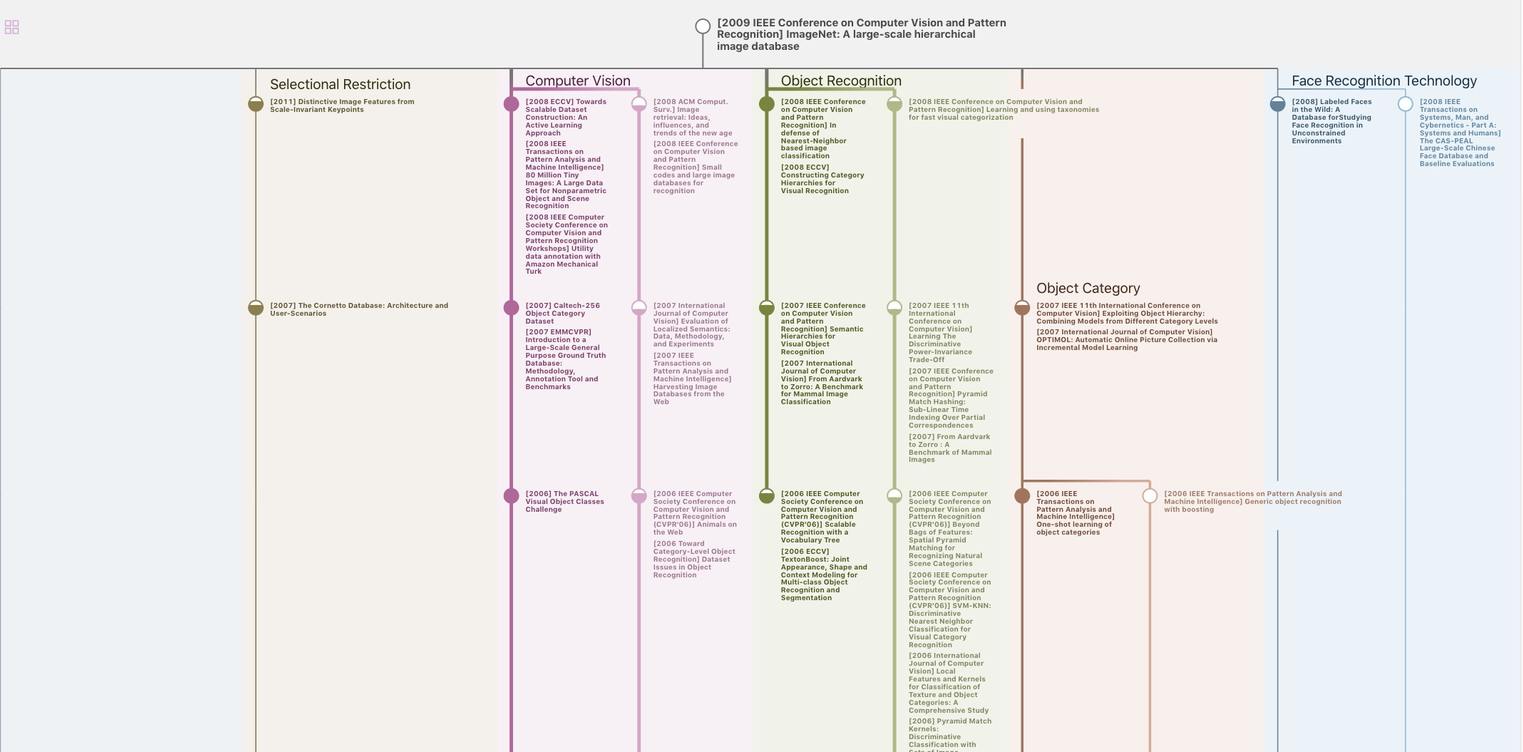
Generate MRT to find the research sequence of this paper
Chat Paper
Summary is being generated by the instructions you defined