Data enhancement based on M2-Unet for liver segmentation in Computed Tomography
Biomedical Signal Processing and Control(2023)
摘要
Liver cancer is one of the diseases with the highest mortality in the world. Accurate segmentation of liver regions from liver CT data is a necessary preprocessing stage of computer-aided detection and diagnosis algorithms. In view of the small number of existing medical image data sets and difficulty in acquisition, this paper expanded the effective data set based on an improved conditional generation adversarial network pix2pix. We added random noise to the input of the generator, and the last layer of the output of the discriminator. Moreover, the original fully connected layer was replaced with a probability matrix with a size of 16 × 16. This is to make the discriminator more delicate. We merged the block module in MobileNet-v2 and U-Net (M2-Unet) to achieve liver segmentation. In addition to the training and validation using the public competition data set LiTs, we also constructed a new liver data set. The Dice similarity coefficient of the algorithm in this paper is 88.7%. The proposed method has improved to the ordinary U-Net algorithm. In the test phase, each image only needs 0.072 s to complete the segmentation on average, which exceeds the segmentation speed of specialists. Experimental results show that algorithm in this paper can accurately segment the liver and meet the requirements of realtime segmentation.
更多查看译文
关键词
Liver segmentation,Conditional GAN,MobileNet-v2,U-net
AI 理解论文
溯源树
样例
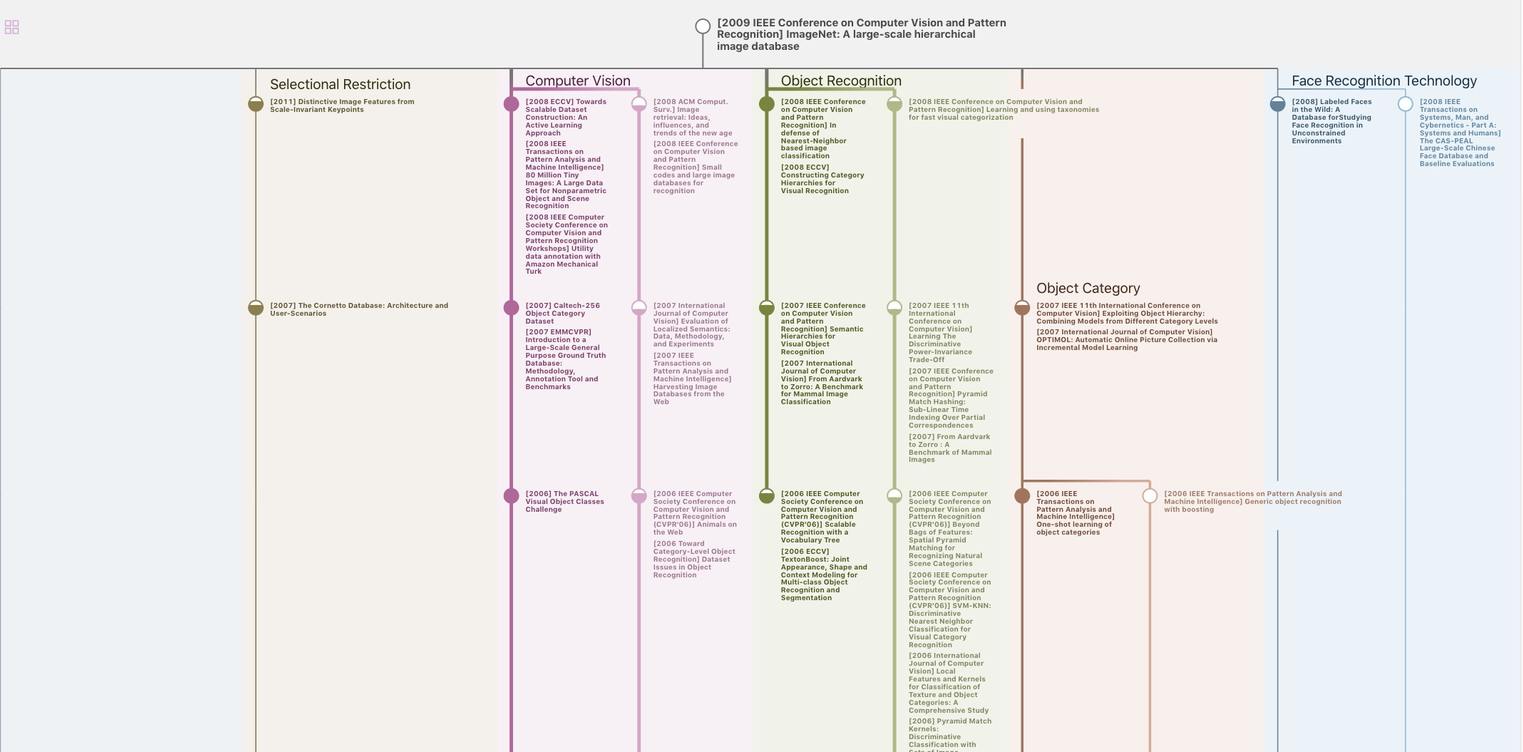
生成溯源树,研究论文发展脉络
Chat Paper
正在生成论文摘要