Classification of cardiac electrical signals between patients with myocardial infarction and normal subjects by using nonlinear dynamics features and different classification models
Biomedical Signal Processing and Control(2023)
摘要
Myocardial infarction (MI) is one of the leading causes of human mortality and morbidity around the world. Despite that much progress has been made for MI detection based on medical image analysis in recent years, most of them suffer from their expensiveness and invasive nature. In this paper, we demonstrate abnormalities of electrocardiogram (ECG) in a new quantifiable manner using nonlinear dynamics features and different classification models. Time-varying ECG data are represented as three-dimensional vectorcardiogram (VCG) and the underlying cardiac dynamics. Nonlinear dynamics measures, including entropy variability measures, complexity measures and chaotic measures, are calculated and fed into different machine learning methods for the classification task. The extracted nonlinear dynamics measures reflect in-depth cardiac dynamics characteristic, which is shown to be more sensitive to subtle ECG modifications. Therefore, it is expected to provide an early detection tool for latent ECG modifications before obvious diagnostic changes are observed. Experiments on the PTB database are conducted to demonstrate the efficiency of the proposed method.
更多查看译文
关键词
Myocardial infarction (MI) detection,Electrocardiogram (ECG),Vectorcardiogram (VCG),Cardiac dynamics,Feature selection
AI 理解论文
溯源树
样例
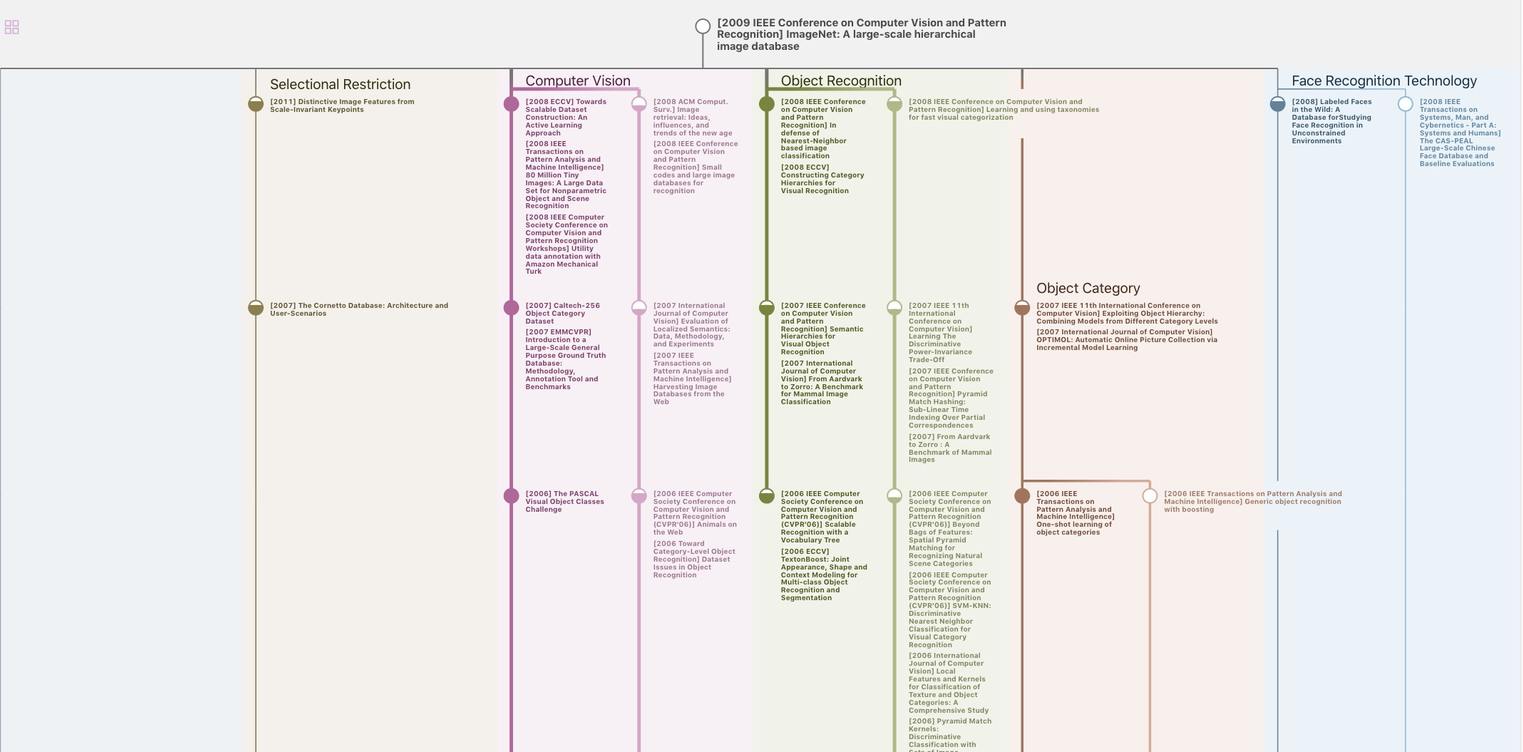
生成溯源树,研究论文发展脉络
Chat Paper
正在生成论文摘要