A Segmented PageRank-Based Value Compensation Method for Personal Data in Alliance Blockchains
Big Data Research(2022)
摘要
Alliance blockchains provide a multi-party trusted data trading environment, promoting the development of the data trading market in which the value compensation for personal data is still a key issue. However, limited by the data format and content, traditional attempts on data value compensation cannot form a widely applicable solution. Therefore, we propose a universal value compensation method for personal data in alliance blockchains. The basic idea of this method is to evaluate the value weight of data based on the collaborative relationship of data value. First, we construct a Data Collaboration Markov Model (DCMM) to formalize the collaboration network of data value. Then, aiming at data collaboration networks with different structures, the corresponding Segmented PageRank (SPR) algorithm is proposed. SPR can universally evaluate the value weight of each data account without being subjected to the data format or content. Finally, we theoretically deduce that the time complexity and space complexity of SPR algorithm are respectively 1/K and 1/K2 taken by PageRank algorithm. Experiments show the feasibility and superior performance of SPR.
更多查看译文
关键词
Alliance blockchains,Personal data,Value compensation,Markov model,Segmented PageRank (SPR)
AI 理解论文
溯源树
样例
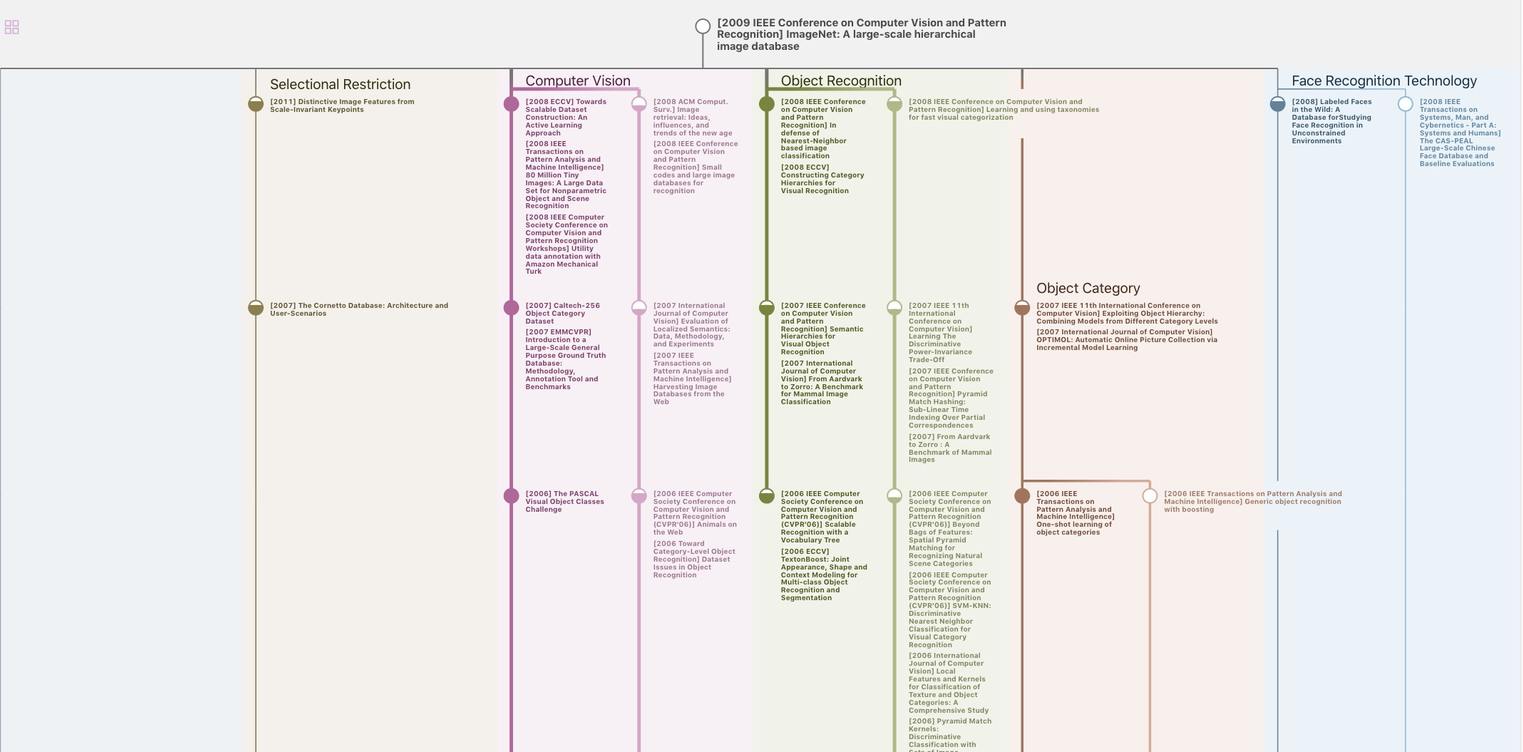
生成溯源树,研究论文发展脉络
Chat Paper
正在生成论文摘要