The Spring Minimum in Subseasonal 2-m Temperature Forecast Skill over North America
Monthly Weather Review(2022)
摘要
Abstract On average, 2-m temperature forecasts over North America for lead times greater than two weeks have generally low skill in operational dynamical models, largely because of the chaotic, unpredictable nature of daily weather. However, for a small subset of forecasts, more slowly evolving climate processes yield some predictable signal that may be anticipated in advance, occasioning “forecasts of opportunity.” Forecasts of opportunity evolve seasonally, since they are a function of the seasonally varying jet stream and various remote forcings such as tropical heating. Prior research has demonstrated that for boreal winter, an empirical dynamical modeling technique called a linear inverse model (LIM), whose forecast skill is typically comparable to operational forecast models, can successfully identify forecasts of opportunity both for itself and for other dynamical models. In this study, we use a set of LIMs to examine how subseasonal North American 2-m temperature potential predictability and forecasts of opportunity vary from boreal winter through summer. We show how LIM skill evolves during the three phases of the spring transition of the North Pacific jet—late winter, spring, and early summer—revealing clear differences in each phase and a distinct skill minimum in spring. We identify a subset of forecasts with markedly higher skill in all three phases, despite LIM temperature skill that is somewhat low on average. However, skill improvements are only statistically significant during winter and summer, again reflecting the spring subseasonal skill minimum. The spring skill minimum is consistent with the skill predicted from theory and arises due to a minimum in LIM forecast signal-to-noise ratio.
更多查看译文
关键词
North America, Teleconnections, Surface temperature, Machine learning, Subseasonal variability
AI 理解论文
溯源树
样例
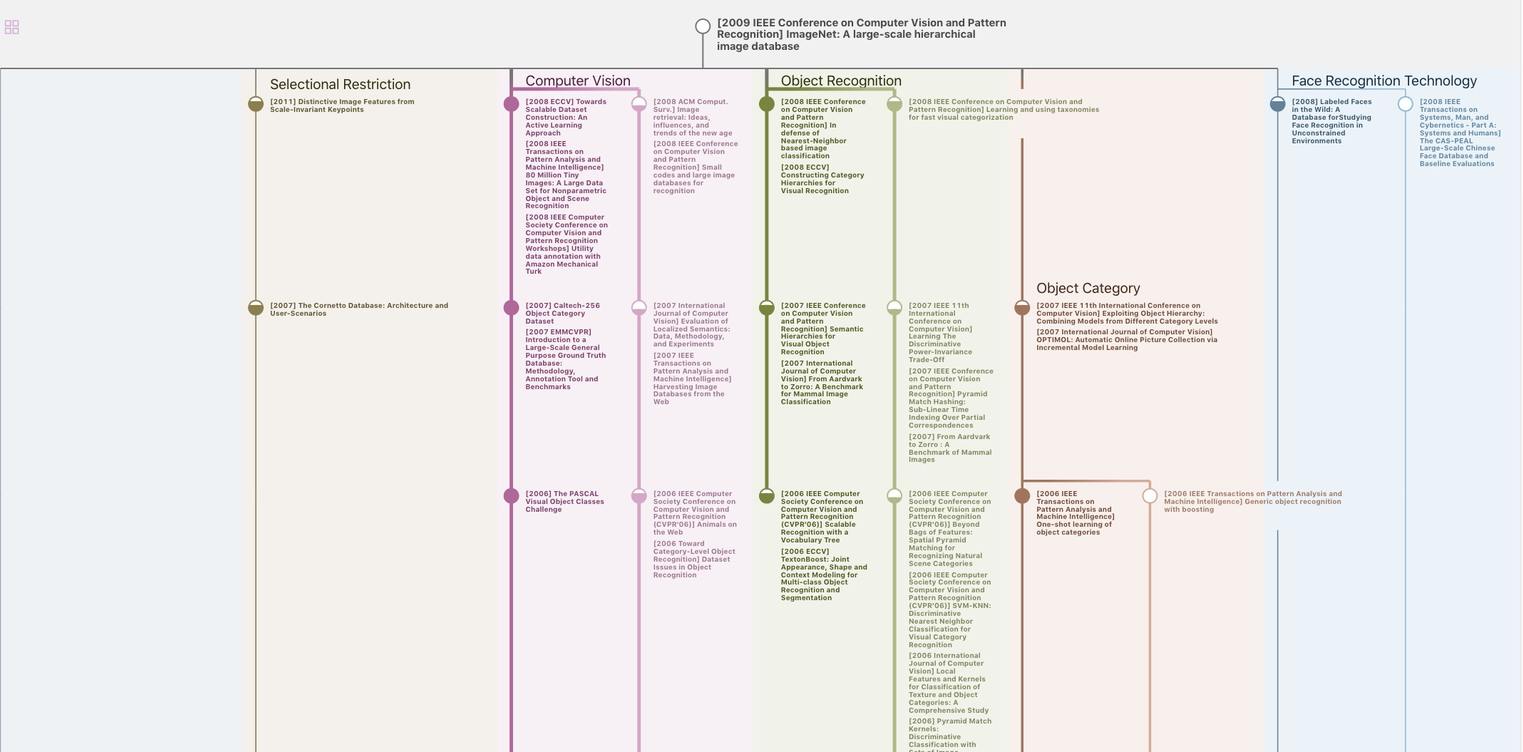
生成溯源树,研究论文发展脉络
Chat Paper
正在生成论文摘要